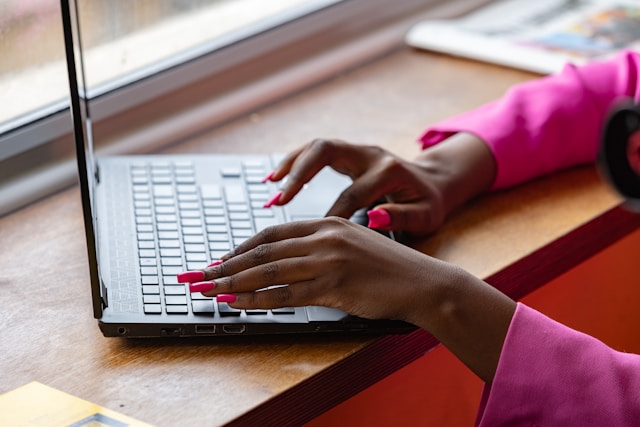
There are significant gender gaps in ratings for quality and problem-solving abilities
Subjective performance evaluations play a crucial role in hiring and promotion decisions, but they can introduce the risk of discriminatory bias. We explore this issue in a recent IZA Discussion Paper. To measure gender bias and understand its causes, we use randomized experiments combined with data from 60,000 mock video interviews on a platform for software engineers. We find significant gender gaps in ratings for code quality and problem-solving abilities: both are rated 12% of a standard deviation lower for women.
To determine if these gaps were due to actual differences in code quality, we began our analysis by controlling for the results of automated unit tests, which check if the code produces correct answers. Even after accounting for these tests, half of the gender gap remained unexplained.
Since assessing code quality is difficult, one possibility is that evaluators mistakenly believe that code written by women is worse. To test this, we examined the impact of showing evaluators the unit test results. However, better information about code quality did not reduce the gender gap in evaluations; it stayed the same.
To better understand this finding, we performed a second experiment. Video interaction was removed, allowing us to compare “blind” evaluations (where gender is unknown) to “non-blind” evaluations (where gender is revealed by the coder’s first name).
In the first step, we compared blind evaluations of code written by men and women. With gender hidden, evaluations should be unbiased. The results showed no gender gap, implying that the quality of code written by men and women is similar.
In the second step, we checked if bias appeared when gender was revealed. It did not: men and women received similar ratings. This suggests that bias is more complex than traditional models of discrimination, which would predict bias when the coder’s gender is known.
So, what explains the gender gaps in the platform evaluations? Our results point to implicit bias triggered during face-to-face interactions. Video interaction is the key difference between the platform and the experimental evaluations.
Two additional findings support this explanation. First, the disparities were larger among evaluators from regions with higher scores on the implicit association test, which measures unconscious biases. Second, longer face-to-face interactions increased the gender gaps: a 15-minute longer interview widened the gap by 4% of a standard deviation.
Our findings suggest new solutions to address gender gaps in technical evaluations. For instance, separating coding assessments from face-to-face interviews could reduce biases in evaluating cognitive skills while still gaining insights from personal interaction.
There are also implications for research. Discrimination is often measured by changing the name on a CV to signal a different group identity. These studies have produced mixed results on gender, with recent papers finding little bias against female names. However, our results indicate that this approach misses significant biases that only emerge during face-to-face interactions.
© Abdelrahman Amer, Ashley C. Craig, and Clémentine Van Effenterre
Abdelrahman Amer is PhD candidate at the University of Toronto
Ashley C Craig is Assistant Professor of Economics at the University of Michigan and IZA Research Affiliate
Clémentine Van Effenterre is Assistant Professor of Economics at the University of Toronto and IZA Research Affiliate
Please note:
We recognize that IZA World of Labor articles may prompt discussion and possibly controversy. Opinion pieces, such as the one above, capture ideas and debates concisely, and anchor them with real-world examples. Opinions stated here do not necessarily reflect those of the IZA.
Related IZA World of Labor content:
Employers and the gender wage gap by John Forth Nikolaos Theodoropoulos
Eliminating discrimination in hiring isn’t enough by Mackenzie Alston
Correspondence testing studies by Dan-Olof Rooth
Key Topics page on What is the gender divide?
Key Topics page on Workplace discrimination
Foto by ThisisEngineering on Unsplash