Elevator pitch
Statistical models can help public employment services to identify factors associated with long-term unemployment and to identify at-risk groups. Such profiling models will likely become more prominent as increasing availability of big data combined with new machine learning techniques improve their predictive power. However, to achieve the best results, a continuous dialogue between data analysts, policymakers, and case workers is key. Indeed, when developing and implementing such tools, normative decisions are required. Profiling practices can misclassify many individuals, and they can reinforce but also prevent existing patterns of discrimination.
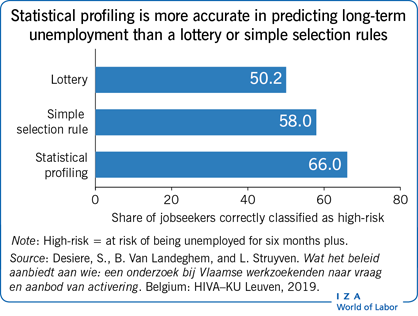
Key findings
Pros
Systematic patterns between socioeconomic and sociodemographic variables, and the outcome of interest can be revealed by statistical models.
Statistical models can direct future research on why some groups are more at risk, and on how the gap can be closed.
Statistical profiling models offer an indication of the potential duration of an unemployment spell.
Under some circumstances, statistical models can reduce existing patterns of discrimination.
Cons
The improvement in profiling accuracy when using statistical models as opposed to a lottery is modest and many individuals tend to be misclassified.
Statistical profiling risks reinforcing existing patterns of discrimination.
Current statistical profiling models predict outcomes, but do not reveal which program works for whom.