Elevator pitch
Statistical models can help public employment services to identify factors associated with long-term unemployment and to identify at-risk groups. Such profiling models will likely become more prominent as increasing availability of big data combined with new machine learning techniques improve their predictive power. However, to achieve the best results, a continuous dialogue between data analysts, policymakers, and case workers is key. Indeed, when developing and implementing such tools, normative decisions are required. Profiling practices can misclassify many individuals, and they can reinforce but also prevent existing patterns of discrimination.
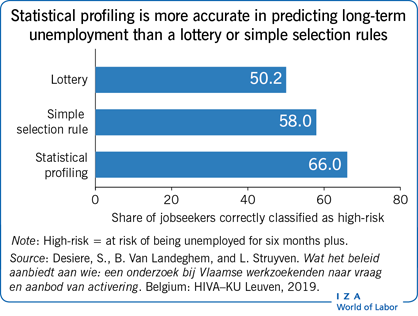
Key findings
Pros
Systematic patterns between socioeconomic and sociodemographic variables, and the outcome of interest can be revealed by statistical models.
Statistical models can direct future research on why some groups are more at risk, and on how the gap can be closed.
Statistical profiling models offer an indication of the potential duration of an unemployment spell.
Under some circumstances, statistical models can reduce existing patterns of discrimination.
Cons
The improvement in profiling accuracy when using statistical models as opposed to a lottery is modest and many individuals tend to be misclassified.
Statistical profiling risks reinforcing existing patterns of discrimination.
Current statistical profiling models predict outcomes, but do not reveal which program works for whom.
Author's main message
Statistical profiling can help identify individuals at risk of becoming long-term unemployed and highlight appropriate predictive variables. However, such models do not unravel the mechanisms behind these relationships, and will hence not inform directly about suitable policies to tackle long-term unemployment. It is also not straightforward to evaluate whether targeted policies are effective. Additionally, policymakers who consider relying on statistical profiling to direct jobseekers to job counseling, training programs, or other social programs should evaluate the ethical implications: individuals are often misclassified and statistical profiling can reinforce patterns of discrimination.
Motivation
While the act of profiling jobseekers is as old as employment activation itself, the methods of profiling have changed profoundly.
Traditionally, employment services have profiled jobseekers in a rule-based manner, often segregating them into large general groups, such as younger versus older, and affording case workers some degree of discretion. More recently, however, governments are increasingly developing and implementing statistical profiling models based on administrative and/or survey data to predict whether a jobseeker will become long-term unemployed. This development is in line with a broader expectation among governments to conduct evidence-based policy making, to prevent prolonged spells of joblessness, and to tailor services to individuals. Given the increasing popularity of statistical profiling and the increasing opportunities to build profiling models, it is useful to review these practices, to discuss how they might inform policy making, and to examine the potential moral implications policymakers face when implementing these models to target the unemployed.
Discussion of pros and cons
Transitioning from standard into advanced statistical profiling models
Statistical profiling has traditionally been based on classic analytical techniques such as cross-tabulations or regression models [1]. However, novel machine learning techniques are increasingly being implemented in standard statistical software packages. This advancement in the field is facilitated by exponential improvements in computing power and rapidly growing availability of administrative data. These developments have offered public employment services such as the Flemish Employment and Vocational Training Office the opportunity to develop statistical profiling models using modern, data-hungry, and computationally expensive machine learning techniques [2]. These machine learning techniques are better at predicting which jobseekers are at risk of becoming long-term unemployed or of exhausting their benefits than standard regression models.
Academic research has certainly been an important source of inspiration for the development of statistical profiling. The academic literature in various disciplines across the social sciences has explored the determinants of unemployment duration or benefit exhaustion. These studies often provide very useful ideas for developing profiling models. However, building such a model remains a matter of trial and error and making ad hoc decisions. First, whether or not variables are good predictors will differ across countries. Second, in smaller-scale academic studies, variables (such as measures for soft skills) that are not always straightforward to collect at the population level (e.g. if this requires filling out extensive surveys) are often used. Finally, apart from practical hurdles, legal restrictions related to privacy protection can prevent policymakers from collecting, using, or merging data for the development of profiling models.
Predictors of unemployment duration
In 2000, a case study was conducted in Minnesota, USA, to investigate predictors of unemployment duration [3]. Although small-scale (989 usable observations), the study was rather influential as it inspired, for example, the Dutch Public Employment Service when it developed its profiling model. The case study recruited unemployment insurance claimants who were then re-interviewed one year later to track their labor market history. Variables found to be associated with lower reemployment success were being non-white, having worked with the previous employer for more than five years, and being female with children under the age of 18. Variables associated with higher reemployment success were measures of economic need (number of children under the age of 18 and economic hardship). Other variables found to have explanatory power were a person's region of residence and occupation. The explanatory power of such macroeconomic variables has also been widely documented in policy reports discussing statistical profiling models based on administrative data. Interestingly, a large number of variables such as years of education, self-reported job search, and conscientiousness, were not found to be significant. This might be due to the small sample size, but there might also be several mechanisms at work that cancel each other out. For example, it is clear that a good labor market history, a resume with few gaps, and a short ongoing unemployment spell are very important for being successful in the labor market. But, as the results in this case study show, being employed with one employer for a long time predicts lower reemployment success, perhaps because skills have become obsolete or because these individuals are not used to negotiating with employers or being active in the job market. Having young children might create a need for higher income, or to accept a job more quickly and to increase search efforts, but as the above results suggest, opportunity costs can arise due to caring responsibilities. Finally, there can also be mediating effects, since variables are correlated with each other. For example, the authors found that conscientiousness predicts higher reemployment success once other variables are dropped from the model [3].
A similar project, but of a much larger scope, was set up by the Institute of Labor Economics (IZA) and is called the IZA Evaluation Dataset [4]. The IZA Evaluation Dataset is a German nationwide sample of 12 monthly cohorts of individuals who became unemployed between June 2007 and May 2008; the dataset tracks these individuals for 2.5 years after they entered unemployment. Regression analyses on these data show that higher school-aged educational attainment and further (professional) training are associated with fewer months spent in unemployment, as are being younger, having a strong employment record, having high numeracy skills, a high internal motivation or locus of control, being conscientious, being optimistic about finding a job, and having a good labor market history. Again, macroeconomic conditions (especially the local unemployment rate) can have strong predictive power.
It is interesting that in the literature, especially the economics literature, there are not many (influential) studies reporting exercises that are very close to statistical profiling of the unemployed. There are two main reasons for this. First, the international literature is often more interested in understanding how education and cognitive and non-cognitive skills are related to labor market success within the general population, and less so in examining a specific subsample of individuals who enter unemployment. Second, and more importantly, the economics literature is not just interested in models that accurately predict an outcome. The literature pays more attention to the mechanisms that actually lead to these outcomes. Take the Minnesota study above as an example, in which some variables appear to have an effect through different channels [3]. It is quite difficult to disentangle these effects; a setting is needed in which it is possible to evaluate what happens if one variable changes, all else being equal. Therefore, studies tend to focus on one factor at a time, rather than presenting a comprehensive model that accurately predicts ex post outcomes. But statistical profiling models offer a prediction of the unemployment spell for each individual, which, according to economic research, is very relevant information for jobseekers themselves. It is well-known that jobseekers are often too optimistic about their employment chances. These biased perceptions imply that they take different decisions with regard to their job search strategy than if they had the correct information [5].
Normative implications of statistical profiling
Before asking data analysts to build and implement a statistical profiling model, policymakers should define the outcome variable that the statistical model should predict (e.g. exhausting benefits, becoming long-term unemployed) and decide how they want to use this information. For example, policymakers could choose to route high-risk individuals to a mandatory training program, or to offer them subsidized training. However, this is not the endpoint of the policymaker's responsibility, as several key aspects must be considered when employing such models.
A first area of concern involves potential misclassifications produced by the model. In the last large-scale exercise to investigate profiling models used in different US states, the highest Profiling Score Effectiveness Metrics were around 0.25, and the lowest were below 0.1 [1]. As models will never perfectly predict an ex-post outcome, data analysts and policymakers must have a dialogue about which decision rule to implement, and how to trade sensitivity for specificity. Suppose a given government is very worried about people in need not getting the appropriate support while specific support is available for individuals who are categorized as high-risk. This government would hence be interested in profiling with a high specificity: those who are categorized as low-risk should indeed be those who are not exhausting their benefits ex post. If the statistical model provides predictions for the chance of exhausting benefits, the decision rule could then be adapted by choosing a lower cut-off value, above which individuals are categorized as high-risk.
Conversely, consider a post-election situation in which there is a regime switch: the new government wants to tighten budgets and might thus be worried about people having access to support who actually do not need it. This new government is hence interested in a profiling practice with a high sensitivity: those who are categorized as high-risk should indeed exhaust their benefits ex post. The new government could hence decide to increase the threshold value above which someone is classified as high-risk. The pool of high-risk individuals will then become smaller. If misclassification is less likely near the tails of the ranking, the sensitivity will increase. The specificity, however, will decrease, as the fraction of those who eventually exhaust their benefits will increase among the low-risk pool.
It is hence clear that different regimes might prefer different sensitivity–specificity combinations, and that the optimal combination (given the model) depends on how profiling is intended to be used. A mandatory program for the high-risk group might lead to a different conclusion than an opportunity for voluntary training for the high-risk group.
Another very important normative implication is the potential reinforcement of stigmatization of minorities. Statistical profiling models aim to predict outcomes but do not really focus on causal relationships. Good examples of this are the variables for someone's employment history. These often tend to be good predictors for unemployment duration, but as discussed above, do not say much about the mechanisms. When implementing these predictive models, however, it is still important to have an idea of the relationship between cause and consequence when it comes to understanding the normative implications of a profiling practice. A huge body of literature offers very convincing evidence that there is a causal relationship between race and the job finding rate, or, in other words, that labor market discrimination exists [6]. As a consequence, race can be an important determinant of whether or not someone exhausts their benefits. But is it therefore warranted to invite minority jobseekers more often to demanding mandatory training sessions? On the other hand, if profiling is primarily used to offer additional opportunities to vulnerable jobseekers (rather than to monitor job search), inviting proportionally more minority jobseekers may pose fewer ethical questions, and may even be considered positive discrimination [7].
If this relationship is causal, and ethnic minorities suffer from discrimination, policymakers might prefer not to reinforce such stigmatization. It is, however, not straightforward to avoid such reinforcement by statistical profiling models. In practice, public employment services complying with data privacy laws like the General Data Protection Regulation (GDPR) have adopted rules making it unlawful to include contentious variables such as gender, age, and ethnicity in the statistical model. However, these contentious variables are often correlated with other predictors, such as proxies for local labor market conditions. Indeed, minorities tend to be concentrated in certain areas. Recently, economists have proposed a simple methodology to partially mitigate the problem and have applied their suggestion to the Worker Profiling and Reemployment Services model in the US [8]. The main idea is that the contentious variables are still included in the model, but only at the prediction stage. The actual values of the latter variables are not used, but rather replaced with the average for the population. This practice avoids the possibility that other explanatory variables pick up the effect of labor market discrimination. Obviously, the predictive power of the model decreases, but the reinforcement of stigmatization does as well: in the study's empirical example, the percentage of black jobseekers in the high-risk group decreases from 22% to 16%.
It is worth noting that after such adjustments, statistical profiling models might even prevent discrimination, especially when the alternative is granting case workers discretionary power to allocate people to programs. In the hiring context, for example, there is evidence that limiting hiring managers’ discretionary power to overrule test results increases the quality of the pool of hired workers [9]. Algorithms are more transparent than the minds of decision-making humans, who often are prone to unconscious biases against certain groups [10].
Limitations and gaps
Profiling models reveal systematic patterns in unemployment duration, though the differences between people remain largely unexplained by the data. This means that such models do a bit better in classifying individuals ex ante than a lottery, but many individuals still tend to be misclassified ex post. Moreover, profiling models focus on predicting an outcome, and not on causal relationships. The latter implies that it can be difficult to interpret the results from a complex profiling model. Many variables in such models might also impact each other; for example, the level of education someone has will have had an impact on previously earned income. Including all these variables in a model will help policymakers to predict who is at risk of becoming long-term unemployed, but the model is not suitable to predict what will happen to unemployment duration if training vouchers are given to people with low levels of education, for example. While statistical profiling models might help identify at-risk people, they do not reveal which policy programs are effective for whom.
In any case, statistical profiling models will support rather than replace case workers. Many public employment services are currently experimenting with striking the right balance between automated and human-based decision making so that both approaches can reinforce each other. Finally, it is worth emphasizing that complex statistical profiling tools should ultimately improve the labor market outcomes of jobseekers. This does not yet appear to have been carefully evaluated in the academic literature, although studies have shown that statistical profiling rules can outperform case workers with regard to assigning jobseekers to the optimal program [11].
Summary and policy advice
Matching survey data with administrative data can offer windows of opportunity to build more accurate statistical profiling models. While statistical profiling models are helpful to improve the identification of jobseekers who are at risk of becoming long-term unemployed, there are some caveats when it comes to their efficacy at better targeting unemployed individuals. Even if models contain a rich set of variables, a large number of individuals will be misclassified. Moreover, there is a risk of reinforcing the stigmatization of minority groups if used incorrectly. Policymakers need to maintain a consistent dialogue with researchers to determine the ideal trade-off between false positives and false negatives.
Statistical profiling can provide an additional source of information for case workers and public employment services. While it does not inform about causal relationships, it can help raise the question of why one group is more at risk than another. In this way, statistical profiling can serve as a guide to develop research projects that investigate causal mechanisms. Policymakers may want to promote the combination of statistical profiling with causal inference methods such as large-scale randomized controlled trials in combination with machine learning. For example, whether people at risk of becoming long-term unemployed really do benefit more from certain types of programs can be identified [12]. Or, with regard to (causal) machine learning techniques it might be investigated whether programs have heterogeneous treatment effects [11]. Instead of profiling jobseekers with respect to the predicted unemployment duration, they could then be profiled with respect to the predicted effectiveness of a program.
Acknowledgments
The authors thank two anonymous referees and the IZA World of Labor editors for many helpful suggestions on earlier drafts.
Competing interests
The IZA World of Labor project is committed to the IZA Code of Conduct. The authors declare to have observed the principles outlined in the code.
© Bert van Landeghem, Sam Desiere, and Ludo Struyven
Key principles of statistical profiling: The statistical model and decision rules
Source: Black, D. A., J. Galdo, and J. A. Smith. “Evaluating the Worker Profiling and Reemployment Services System using a regression discontinuity approach.” American Economic Review 97:2 (2007): 104–107.
Profiling versus targeting
Evaluating profiling practices
PSEM = 1 - (100-y)/(100-x)
Where x is the overall percentage of individuals who eventually exhaust their benefits, and y the percentage of individuals with the highest profiling score who eventually exhaust their benefits.
If the model does not do any better than a lottery, the metric will be zero, whereas a perfect model will return a value of one. As an example, imagine a state where 20% of new benefit claimants will eventually exhaust their benefits. For the 20% of individuals with the highest profiling score (as predicted by a statistical model), 60% of individuals eventually exhaust their benefits. The PSEM for this model is then equal to:1-(100–60)/(100–20) = 0.5Source: Sullivan, W. F., L. Coffey, L. Kolovich, C. W. McGlew, D. Sanford, and R. Sullivan. Worker Profiling and Reemployment Services Evaluation of State Worker Profiling Models: Final Report. ETA Occasional Paper No. 15, 2007.