Elevator pitch
Discrimination is a complex, multi-factor phenomenon. Evidence shows widespread discrimination on various grounds, including ethnic origin, sexual orientation, gender identity, religion or beliefs, disability, being over 55 years old, or being a woman. Combating discrimination requires combining the strengths of a range of anti-discrimination policies while also addressing their weaknesses. In particular, policymakers should thoroughly address prejudice (taste-based discrimination), stereotypes (statistical discrimination), cognitive biases, and attention-based discrimination.
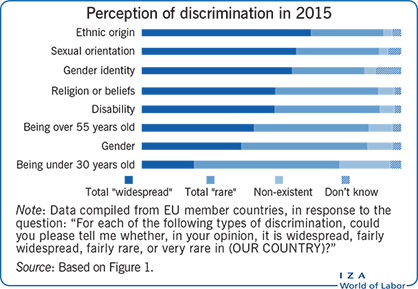
Key findings
Pros
Banning discrimination against at-risk groups likely contributes to protecting them once they are hired.
Monitoring the composition of the workforce together with wage gaps helps identify non-complying firms and, hence, improves the share and earnings of individuals at risk of discrimination.
Prejudice-reducing policies, such as de-biasing interventions at school, should curtail taste-based discrimination.
Narrowing the productivity differential across groups and easing employers’ access to information on candidates’ individual productivity can reduce statistical discrimination.
Machine learning for screening résumés and managing careers limits the expression of cognitive biases and attention-based discrimination.
Cons
Anti-discrimination laws do not seem to reduce hiring discrimination, and may even increase it.
Defining target values for the workforce composition and wage gaps of different groups is not feasible.
Little is known about how to calibrate de-biasing interventions in order to maximize their impact and persistence.
Policies aiming to counter statistical discrimination are known to generate perverse effects.
It is not possible to develop human resource (HR) analytics, the use of big data for human resources, in small firms.