Elevator pitch
Discrimination is a complex, multi-factor phenomenon. Evidence shows widespread discrimination on various grounds, including ethnic origin, sexual orientation, gender identity, religion or beliefs, disability, being over 55 years old, or being a woman. Combating discrimination requires combining the strengths of a range of anti-discrimination policies while also addressing their weaknesses. In particular, policymakers should thoroughly address prejudice (taste-based discrimination), stereotypes (statistical discrimination), cognitive biases, and attention-based discrimination.
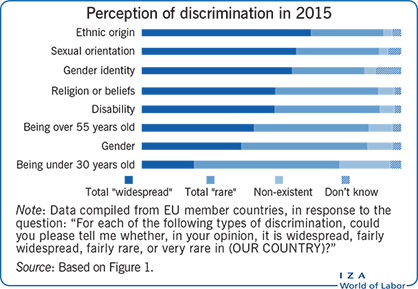
Key findings
Pros
Banning discrimination against at-risk groups likely contributes to protecting them once they are hired.
Monitoring the composition of the workforce together with wage gaps helps identify non-complying firms and, hence, improves the share and earnings of individuals at risk of discrimination.
Prejudice-reducing policies, such as de-biasing interventions at school, should curtail taste-based discrimination.
Narrowing the productivity differential across groups and easing employers’ access to information on candidates’ individual productivity can reduce statistical discrimination.
Machine learning for screening résumés and managing careers limits the expression of cognitive biases and attention-based discrimination.
Cons
Anti-discrimination laws do not seem to reduce hiring discrimination, and may even increase it.
Defining target values for the workforce composition and wage gaps of different groups is not feasible.
Little is known about how to calibrate de-biasing interventions in order to maximize their impact and persistence.
Policies aiming to counter statistical discrimination are known to generate perverse effects.
It is not possible to develop human resource (HR) analytics, the use of big data for human resources, in small firms.
Author's main message
Anti-discrimination policies that rely on a punitive approach are not sufficient to combat discrimination. They must be complemented by policies that counter prejudice and stereotypes and that limit the expression of cognitive biases and attention-based discrimination. New research is shedding light on the impact of interventions that aim to reduce taste-based discrimination, dampen statistical discrimination, and address people’s mental limitations. However, much more research is needed to understand how to calibrate the various components of this policy mix in order to improve its effectiveness.
Motivation
In 2015, a majority of the population of the EU viewed discrimination as being widespread, with this proportion having increased over time (Figure 1). Moreover, 62% of those surveyed were in favor of new measures to protect groups at risk of discrimination (as opposed to 27% who did not consider such measures necessary and 11% who did not know). Many Europeans are indeed critical in their assessment of the effectiveness of national efforts to fight discrimination; less than a third believe the efforts made in their country are impactful. In particular, a large share of respondents perceive that discrimination exists in recruitment practices. Yet little is known about how to devise effective anti-discrimination policies, particularly in the labor market, although new evidence is providing clearer insights into “what works.”
Discussion of pros and cons
Discrimination in the labor market refers to a situation in which equally productive individuals are rewarded differently due to their membership of different groups (defined on grounds at least as diverse as those presented in the illustration on page 1 and Figure 1). Consistent with the divide between prejudice and stereotypes in social psychology, the economics literature distinguishes two potential sources of discrimination. On the one hand, employers, co-workers, and/or customers may harbor a distaste for a particular group of individuals that often turn out to be members of the so-called “out-group.” Such “taste-based discrimination” flows from prejudice (or prejudgment), i.e. beliefs without sound knowledge of the relevant facts. On the other hand, discrimination can also derive from a more rational process. Because they do not observe candidates’ productivity perfectly, profit-maximizing recruiters rely on their supposedly rational beliefs about how the distribution of unobserved productive individual characteristics correlates with group membership. This is the so-called “statistical discrimination” that constitutes a manifestation of stereotypes, i.e. mental representations aiming to identify real differences between groups. While taste-based discrimination relies on misrepresentations about the out-group, statistical discrimination is supposed to reflect actual differences in productivity across groups.
In considering potential solutions to the discrimination problem, it is possible to distinguish four broad categories of anti-discrimination policies: (i) policies that aim to sanction discriminatory behavior toward job applicants and employees, irrespective of their source; (ii) policies that specifically target taste-based discrimination; (iii) policies that mainly focus on statistical discrimination; and (iv) policies that seek to limit cognitive biases and attention-based discrimination (the latter being a consequence of taste-based and statistical discrimination during résumé screening).
The punitive approach
The punitive approach consists of legally banning discrimination on various grounds as well as developing means of identifying discrimination in order to enforce the threat of sanction. The punitive approach is obviously a necessary step toward combating discrimination in the labor market. But it is not sufficient. First, it does not aim to change the basic or more sophisticated beliefs about different groups that constitute the source of taste-based and statistical discrimination, respectively. Moreover, this approach is not able to identify unfair treatment systematically and, hence, to prevent discrimination.
Since the early 2000s, EU labor laws have prohibited discrimination based on gender, age, racial or ethnic origin, religion or beliefs, sexual orientation, and disability. Such laws are also enforced in a wide range of non-EU countries. They put employers at risk of litigation and, eventually, compensatory and punitive damages if intentional discrimination at the hiring, wage determination, or firing stages of employment is found. As such, they should dissuade employers from discriminating against legally protected groups. Yet proving discrimination is much easier for victims once they are hired rather than at the hiring stage, unless the recruiter explicitly expresses a preference for a specific group, either in the job advertisement or during the job interview. In this context, anti-discrimination laws likely contribute to reducing unfair treatment against members of the groups they protect after they are hired. But, these laws may also compromise their chances of being recruited in the first place, due to employers’ fear of litigation for terminating their contracts. For instance, a US anti-discrimination law, the Americans with Disabilities Act (ADA), has had a negative impact on the employment of the targeted minority [1]. That said, it has not been possible to identify the component of the ADA that generates this result, since the ADA not only bans discrimination against people with a disability, but also requires employers to accommodate them in the workplace (e.g. by providing wheelchair access, purchasing special equipment, or restructuring jobs to enable part-time or home working). In other words, this negative finding may arise from a higher cost of firing and/or of hiring disabled employees.
Monitoring the workforce composition and wage gaps within firms is viewed as the most straightforward way to identify discriminatory behavior. Workforce composition monitoring typically occurs through the imposition of quotas. As early as 1923, Austria, France, Germany, Italy, and Poland had adopted quota systems, which obliged employers to hire disabled war veterans. Many other countries introduced similar systems after World War II. These quota systems were eventually extended to cover disabled civilians. Firms that fail to comply are typically subject to a tax. Similarly, Executive Order 11246, enacted in 1965, requires US firms with federal contracts to pinpoint under-representation of racial minorities and women in any occupation group relative to availability, and work toward remedying it based on numerical goals. The ultimate punishment for a contractor is to be debarred from doing business with the federal government, sometimes permanently. Monitoring workforce composition allows for the identification of non-complying firms and thus induces compliance with the quota. As an illustration, comparing the number of disabled workers in firms just below and just above the quota threshold in Austria (defined relative to the number of employees) reveals that the latter employ about 12% more disabled workers than the former [2]. Similarly, the share of black employees rose substantially once establishments became subject to federal affirmative action regulation in the US [3].
However, the proportion that discriminated groups would represent were they not discriminated against is unknown, and surely varies across firms (depending on the types of skills that the firm demands and the local supply of skills). Executive Order 11246 mandates that federal contractors seek to employ minorities at rates (at least) proportional to shares of the local and qualified workforce, though “local” and “qualified” are not defined. In other words, monitoring the composition of the workforce together with wage gaps does not inherently prevent discrimination: there is always a risk that the quota is set too low or, too high. The latter case, whereby members from discriminated groups receive more than their fair share of roles, is often mentioned to discredit the use of quotas. This approach thus becomes counterproductive by feeding resentment against the groups it is supposed to help. Various studies have confirmed the stigmatizing effects of affirmative action programs. The hiring and career advancement of their beneficiaries are easily seen as undeserved, i.e. dependent solely on their status as minorities, not on their competence. A similar problem arises with equal pay legislation or the obligation that individuals in the same workplace be given similar wages. Indeed, it is not clear that wage gaps would disappear in the absence of discrimination. Many factors can account for the gender pay gap, on top of employers’ discriminatory practices. In particular, social norms impose specific gender roles to men and women that contribute to women’s lower supply and lower hourly labor earnings: women are generally expected to bear the brunt of child rearing and to shy away from risk, competition, and negotiation.
To identify discrimination at the hiring stage, some stakeholders advocate the use of correspondence studies. From the early 1990s to the mid-2000s, the ILO launched a series of such studies covering eight countries (Belgium, France, Italy, Germany, the Netherlands, Spain, Sweden, and the US). These studies applied to real job offers using the résumés of fictitious applicants who were identical in every respect except their ethnic origin (i.e. nationals versus applicants of non-European backgrounds). If employers invited the two types of applicants at different rates, then ethnicity-based discrimination was revealed (the results indeed pinpointed massive discrimination against ethnic minorities). However, correspondence studies have rarely been used as a basis to sanction discrimination. It is believed that this approach has so far been implemented only in France. Starting from the 2000s, the French mass retailer Casino Group launched several rounds of correspondence studies to self-test for discriminatory practices. This approach has proven successful, since the level of hiring discrimination substantially decreased from one round to the next. In 2016, the French Ministry of Labour went a step further. They decided to implement a correspondence study to monitor potentially discriminatory recruitment practices among 40 companies based in France. This strategy allowed the ministry to sanction, through naming and shaming, those firms that, although they had been found to discriminate on prohibited grounds, did not establish a satisfactory action plan to counter this trend. However, using this method to measure hiring discrimination at the firm level is only feasible if the investigated firm posts a sufficiently high number of job advertisements during the study period. As such, monitoring recruitment practices through correspondence studies is not a realistic option for small- and medium-sized enterprises, which represent the large majority of employers.
Policies aiming to reduce taste-based discrimination
Two main approaches could theoretically help undermine taste-based discrimination: (i) enacting inclusive laws (e.g. same-sex marriage) and (ii) providing “diversity training” for the general public via mass media and/or among subgroups (such as students, employers, or workers). Yet little is known about whether these prejudice-reducing policies are indeed effective at changing behaviors, or for how long.
Laws have the potential to alter the general public’s perception of norms. Individuals likely view laws as reflecting public opinion, either purposefully, to maintain support for these laws, or incidentally, because lawmakers are subject to the same social forces as the public [4]. Moreover, extensive research has shown that people often do conform to a change in the perception of social norms, in part to avoid social rejection.
Do inclusive laws influence individuals’ perception of social acceptance of groups at risk of discrimination? Do they positively impact individual opinion and behavior toward these groups beyond self-reported attitudes, which are prone to social desirability bias (where people respond in a manner that will be viewed favorably by others)? The first experimental evidence on this issue investigated reactions to the June 2015 US Supreme Court ruling in favor of same-sex marriage nationwide [4]. Participants were invited to read a brief article about the likely outcome of the upcoming Supreme Court ruling: they were randomly assigned to read either a positive version, titled “Supreme Court likely to rule in favor of gay marriage,” or a negative one, titled “Supreme Court unlikely to rule in favor of gay marriage.” The results show that institutional decisions shape individuals’ perception of social norms: participants who read the positive article perceived Americans’ current support for gay marriage to be significantly higher, compared with those who read the negative version. Moreover, participants in the former group registered significantly more positive attitudes in support of gay marriage and gay people. Finally, the findings reveal that laws inclusive of lesbian, gay, bisexual, and transgender (LGBT) people may affect opinion and behavior toward this population, beyond self-reported attitudes.
Diversity training is also supposed to help combat taste-based discrimination. The first (and, thus far, only) randomized field experiment to evaluate the impact of prejudice-reducing interventions consists of randomizing whether canvassers visit voters in South Florida to discuss transgender rights (treatment group) or recycling (control group) [5]. The intervention mixes a variety of prejudice-reducing techniques, including perspective taking and empathy. The results show that these conversations (between 56 canvassers and 501 voters) substantially reduce self-declared transphobia, with effects still visible three months after the intervention. Further research is needed, however, to determine which prejudice-reducing technique(s) made the intervention successful and whether the intervention changes behaviors beyond self-reported attitudes.
Policies seeking to dampen statistical discrimination
The most straightforward way to dampen statistical discrimination is to eliminate its source by narrowing the productivity differential across groups. But such an approach is not always possible. For instance, the scope for reducing the productivity gap between disabled and non-disabled individuals is limited by definition, although technological change is dramatically improving the labor market and social inclusion of people with disabilities. Moreover, statistical discrimination can flow from a self-fulfilling process. Evidence suggests that taste-based discrimination induces withdrawal behaviors among the out-group’s members. This situation leaves room for statistical discrimination to arise, which presumably further encourages separation on the part of the out-group. In this regard, analysis of how the upsurge of Islamophobic acts in the wake of “9/11” (September 11, 2001) has impacted on the integration of immigrants from Muslim-majority countries in the US is telling [6]. Even ten years later, immigrants in states where this upsurge was felt most strongly were behaving in ways closer to their origin countries’ norms (such a trend was not observed before the attacks): the rates of endogamy (the custom of marrying within the limits of a local community, clan, or tribe) and fertility were higher, while female participation in the labor market and English proficiency among the whole community were weaker. Similarly, the perception by sexual minorities of being socially rejected impairs their psychological well-being. As an illustration, the reduction in the difference in the number of suicide attempts between those who are lesbian, gay, or bisexual and heterosexuals is substantially smaller in US states that did not adopt same-sex marriage than in others (a trend that was not apparent before the enactment of same-sex marriage protections) [7]. The resulting harmed mental health of sexual minorities in turn likely feeds statistical discrimination against them.
Gender-based statistical discrimination constitutes one of the few illustrations of a productivity gap across groups that does not stem from a self-fulfilling process of the type described above. As such, policymakers can focus on the most straightforward way to dampen statistical discrimination: eliminate its source. In essentially every country, social norms entail that women, due to their biological characteristics, devote more time to childrearing, while men devote more time to working in the labor market. This pervasive norm is only partly counteracted by a general trend toward gender equality. Even in Scandinavian countries, which are the most advanced on this issue, gender norms remain fairly traditional: survey respondents in these countries support the view that women should work full-time before having children and after children have left home, but should work only part-time or not at all when children live at home. In this setting, women likely suffer from statistical discrimination when it comes to high-responsibility jobs, especially when they are of childbearing age. Because women typically bear the brunt of childrearing, recruiters associate them with a lower expected productivity at positions that often entail sacrificing at least some components of family life.
To counter gender-based statistical discrimination, a more radical shift toward egalitarian gender norms is necessary. Such a cultural change can be achieved by better balancing the time that men and women spend at home with children, through the reform of parental leave policies. Many governments are attempting to increase fathers’ share of parental leave in order to promote gender equality and, hence, correct for unequal labor market outcomes. Yet the literature points to the necessity of implementing these policies in stages rather than in one step, or else the reform becomes counterproductive. This lesson is well illustrated by the Swedish case [8], [9]. In particular, when a reform sharply impacts whether fathers take up any parental leave, unintended effects emerge provided the length of the compulsory leave is substantial. Rather than inducing less traditional views on gender roles, the reform is detrimental to women: they compensate for the decreased paid parental leave with additional unpaid leave, leading to a lower total income for the household. Moreover, the probability of the couple’s relationship dissolving increases. This latter effect is concentrated among couples where the mother had relatively low labor income and, hence, where traditional views of gender roles were likely dominant. These perverse effects do not occur when the reform is less intrusive, such as when it only moderately affects the length of fathers’ parental leave.
In instances where statistical discrimination results from a self-fulfilling process, its eradication is trickier. However, a potential solution entails improving employers’ access to information on candidates’ individual productivity, thus limiting their reliance on stereotypes (rather than changing stereotypes themselves, which is surely a desirable but harder to reach outcome). Affirmative action, either through quotas or hiring subsidies, incentivizes recruiters to hire individuals from discriminated groups that are the most productive [3]. In particular, it leads employers to invest in “screening capital”—investments that improve an employer’s ability to screen potential workers, such as employing and training personnel specialists, developing job tests, and building relationships with and utilizing intermediaries, i.e. employment agencies and schools.
However, as already stressed, affirmative action is known to generate perverse effects: because the fair share of people from the minority group is often unknown, there is always the suspicion that the quota or hiring subsidy would be excessively advantageous to minority groups. There are three joint ways to mitigate the resentment among non-discriminated groups induced by affirmative action policies. First, these policies should favor hiring subsidies over quotas since, by offering more flexibility, hiring subsidies better take into account that the (unknown) proportion that discriminated groups would represent were they not discriminated against varies greatly from one firm to the next. Put differently, hiring subsidies do not penalize a firm where this proportion is lower than the quota would be, which is an important step toward stressing the fairness of affirmative action policies and gathering support for them. Second, the level of the subsidies should be computed based on (i) the extent of hiring discrimination against various groups, as measured by correspondence studies, and (ii) the sensitivity of labor demand with respect to labor costs. In other words, these subsidies must be set at a level that ensures closing the average hiring gap across groups ceteris paribus, no more, no less, which is also an important prerequisite to getting the general public on board. Third, hiring subsidies should be accompanied by the development of employment intermediaries specialized in certifying the cognitive and non-cognitive skills of the individuals the subsidies target. In this way, it will be easier for co-workers to view beneficiaries as having been hired based on their competence rather than their status as “eligible for affirmative action programs.”
Obviously, affirmative action policies also require that the information used as a criterion for the hiring subsidy (e.g. race, ethnicity, or country of birth/ancestry) be collected on every individual, a condition that many detractors deem to be too intrusive. Yet this opinion does not seem to be held by the general public. According to the 2015 Eurobarometer on discrimination, a large majority of respondents in the EU are in favor of providing sensitive personal information on an anonymous basis in order to combat discrimination: on average, 72% of respondents are willing to reveal their ethnic origin and 63% their sexual orientation.
Limiting the expression of cognitive biases and attention-based discrimination
Taste-based discrimination is a widespread cognitive bias: people tend to be more hostile toward out-group members, even when they do not expect these individuals to represent any real threat to them. But statistical discrimination, which is supposed to be more rational, is not devoid of cognitive bias either. The stereotypes on which statistical discrimination relies do not always represent real differences between groups [10]. First, stereotypes tend to amplify supposed differences. This implies that stereotypes are especially inaccurate when groups are similar. Second, they are context dependent, to the extent that the assessment of a given target group depends on the group to which it is compared. For instance, the stereotype of Irish typically changes from “red-haired” to “Catholic” when comparing Irish to Scottish people. Third, stereotypes distort reactions to information: stereotypical thinking implies overreaction to information that generates or confirms a stereotype, and underreaction to information that contradicts it (although stereotypes can change if enough contrary information is received). Finally, on top of cognitive biases, an additional source of unfair treatment across groups at the hiring stage lies in attention-based discrimination. The models of taste-based and statistical discrimination implicitly assume that individuals are fully attentive to available information. However, as long as acquiring information is costly, it should be rational for decision makers to optimize how much information to acquire based on expected net benefits. Therefore, in the labor market, where selectivity is high (since firms choose only a few top applicants for an interview), the expected benefits from reading a résumé are smallest for the candidates belonging to the a priori least attractive group. Consequently, these candidates are victims of attention-based discrimination, on top of being subject to taste-based and/or statistical discrimination [11].
In this setting, the use of big data for human resources (HR), i.e. HR analytics, might represent the next frontier to limiting the expression of cognitive biases and attention-based discrimination in recruitment and career management. More precisely, relying on machine learning trained on historical HR data should improve the probability that individuals from discriminated groups will be invited to and will pass job interviews as well as be promoted, for two main reasons. First, although historical data are plagued by cognitive biases, machine learning integrates over each individual’s idiosyncratic biases, thereby cancelling out some (although not all) of the flaws of the human-led processes. Second, and more importantly, machine learning does not indulge attention-based discrimination: it takes all variables into account, and therefore does not underweight positive signals from minority applicants. In particular, HR analytics constitute a better response to discrimination than do anonymous job applications in which the “civil status” of the applicant (including the surname, the given name, the address, and the date of birth of the applicant) is suppressed. Many other variables not hidden in anonymous job applications (such as an applicant’s educational background) can indeed generate attention-based discrimination. Moreover, algorithms can extract information based on the interaction between an applicant’s group membership and education or work experience and, hence, be more accommodating if a candidate with a migration background or from a disadvantaged neighborhood shows below-average education outcomes, labor market experience, or language skills. By contrast, anonymity prevents employers from taking extenuating circumstances into account since they do not observe the applicant’s group membership. These shortcomings of anonymous job applications might explain why no government has passed and enforced laws that mandate them thus far.
One of the first pieces of experimental evidence about the impact of algorithms on the probability of atypical job candidates being hired finds that the machine is better at identifying the “right” candidates. The machine and human screeners disagree on about 30% of candidates: the candidate picked by the machine (but not by the human) is 17% more likely to pass a double-blind face-to-face interview with incumbent workers and receive a job offer, while the marginal candidate picked by a human (but not the machine) is less likely to pass the double-blind interview. Put differently, algorithms benefit candidates who would otherwise have been discriminated against, such as individuals who lack job referrals, those without prior experience, or those with atypical credentials. Impact evaluation of the use of job-testing recruitment technologies further confirms that firms that rely less on human judgment when making hiring decisions end up with better hires [12].
However, the potential for algorithms to limit discrimination is not fully harnessed when they are trained on historical human data, since, by definition, these data reflect discriminatory practices. In particular, observations on individuals belonging to discriminated groups might be too scarce for the algorithms to derive proper inferences about them. And the performance that the algorithm assigns to minority employees might be underestimated. Evidence notably shows that managers biased against ethnic minorities avoid contact with them, leading these minorities to exert less effort [13]. To counter these shortcomings, algorithms could be trained from scratch (what is called the “bandit method”). Whatever the approach (historical or forward-looking), a large number of observations is needed to train the algorithm, meaning that HR analytics cannot easily be internally developed in firms that publish only a few job openings and host only a few employees. It is therefore critical that governments and social partners think about ways to provide small firms with access to relevant data sets (i.e. that match their industry, sector, and position) so that they, too, can take advantage of algorithms to guide their recruitment and career management.
Limitations and gaps
Groups previously discriminated against may remain excluded long after discrimination ends, due to habitual behaviors. This can occur in a number of ways, including: (i) self-stereotyping and self-censorship (when the stigma of social exclusion is so profound that it durably negatively affects individuals’ self-perception); (ii) “fast thinking,” for instance, people living in neighborhoods that lack institutions to prevent crime develop an automatic response of non-compliance to authority that hurts them at school (by contrast, middle- and upper-class youth are not exposed to this tension since their appropriate response to authority is typically the same in the home and school environment); (iii) “adaptive preferences,” in which an oppressed group views its oppression as natural due to individuals’ limited ability to imagine counterfactuals. In other words, anti-discrimination policies must be supplemented by interventions that counter the mental models that discrimination has set up since these models may be powerful enough to outlive the elimination of discrimination. For instance, to counter self-stereotyping, interventions that frame the idea of intelligence as a malleable trait that grows in response to hard work (rather than as a fixed trait) have proven to help socially excluded groups improve their performance.
Summary and policy advice
Anti-discrimination policies that rely on a punitive approach are necessary but not sufficient to combat discrimination. They must be complemented by policies that specifically target (i) taste-based discrimination, through the enactment of inclusive laws and prejudice-reducing interventions, (ii) statistical discrimination, through, for instance, the increase in fathers’ share of parental leave (for a better inclusion of women) and the creation of hiring subsidies (for a better inclusion of other discriminated groups), and (iii) cognitive biases combined with attention-based discrimination, through the use of HR analytics.
However, much more research is needed to measure the impact of prejudice-reducing approaches and its persistence. It is also critical to minimize the perverse effects associated with policies that seek to counter statistical discrimination. In particular, increasing fathers’ share of parental leave in order to promote gender equality can be effective provided this increase is stepwise and modest to start with. Additionally, hiring subsidies must be implemented with caution. They must be set at a level that ensures closing the average hiring gap across groups ceteris paribus, no more, no less. This condition is important to avoid having the individuals that the subsidies target be perceived as receiving more than their fair share and, hence, be further stigmatized. Hiring subsidies should also be accompanied by the development of employment intermediaries specialized in certifying the skills of their beneficiaries to ensure that their hiring and career advancement are regarded as deserved, i.e. dependent on the beneficiaries’ competence rather than their minority status.
Finally, more research should be devoted to figuring out how to limit the expression of cognitive biases and attention-based discrimination with machine learning. To the extent that historical HR data reflect discriminatory practices, it seems essential to train algorithms from scratch, by relying on the so-called bandit method. But this precaution is not sufficient. One must also ensure that the algorithms are trained on a large number of observations, suggesting that they can hardly be developed internally in small firms. In this setting, improving these firms’ access to big data is a key prerequisite to making HR analytics the next frontier for cutting unconscious biases and attention-based discrimination.
Acknowledgments
The author thanks an anonymous referee and the IZA World of Labor editors for many helpful suggestions on earlier drafts. Previous work of the author contains a larger number of background references for the material presented here and has been used intensively in some parts of this article (Valfort, M.-A. LGBTI in OECD Countries: A Review. OECD Social, Employment and Migration Working Paper No. 198, 2017).
Competing interests
The IZA World of Labor project is committed to the IZA Guiding Principles of Research Integrity. The author declares to have observed these principles.
© Marie-Anne Valfort