Elevator pitch
Risk aversion is an important factor in many settings, including individual decisions about investment or occupational choice, and government choices about policies affecting environmental, industrial, or health risks. Risk preferences are measured using surveys or incentivized games with real consequences. Reviewing the different approaches to measuring individual risk aversion shows that the best approach will depend on the question being asked and the study's target population. In particular, economists’ gold standard of incentivized games may not be superior to surveys in all settings.
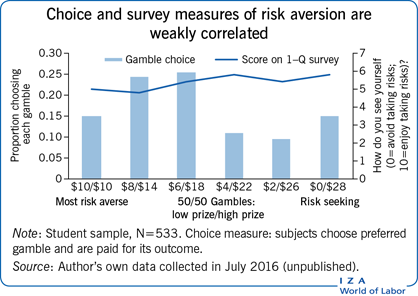
Key findings
Pros
Incentivized tasks are designed so that subjects have an incentive to truthfully report their preferences, while it is costless for subjects to misrepresent their preferences in surveys.
Incentivized games can be structured to provide a precise measure of preferences that can be used to discriminate between different theories of decision making and to statistically estimate a subject's underlying value function (utility function).
Survey measures lack a clear connection to theory, and therefore cannot be structured in the same way as incentivized games.
Cons
Subjects may find incentivized games to be difficult or confusing, while surveys tend to be simpler to understand; this is particularly true for less-educated subjects.
Incentivized games are costly to administer in terms of time (instructions can be complex) and money (incentives must be provided).
It is difficult to adapt incentivized games to represent different decision contexts, while surveys are easier to adapt.
Author's main message
In measuring risk aversion, it is important to consider carefully the purpose of the measure, the costs of alternative measures, and the abilities and experiences of the target respondents. Incentivized tasks provide a more precise measure, but are costly to implement and may be difficult for some subjects to comprehend. Survey measures are easier and less costly to implement and may be better for nonfinancial domains, but may not be sufficiently precise. Overall, the evidence does not support the general belief that incentivized tasks are superior in all (or most) cases, implying that surveys may warrant increased usage in certain contexts.
Motivation
Measuring risk aversion is important. Assumptions about the risk preferences of individuals (risk aversion or risk tolerance) provide the underpinnings for theoretical models of decision-making behavior in areas as diverse as investment, savings, trust, worker compensation, or choice of profession, and for policy recommendations in areas such as environmental regulation, occupational safety, health policy, or the social safety net. Differences in risk preferences across individuals and between groups have been implicated for behaviors such as inadequate retirement savings, poor credit scores, the gender gap in earnings, patterns of wage differences across occupations, use of recreational drugs, and reluctance to invest in new technologies. Reducing the risk of danger or harm is a primary focus of many, even most, regulatory policies, and estimates of aggregate risk aversion play an important role in determining the appropriate level of risk reduction.
Theoretical models of these behaviors rely on empirical estimates of preferences for policy-relevant calibration. Methods for measuring preferences have been developed in two main categories: (i) self-reported survey measures, where subjects report their perceptions of their own risk tolerance or report the likelihood of engaging in specific behaviors; and (ii) incentivized tasks or games, where subjects evaluate or make choices among risky alternatives. Surveys rely on self-perceptions, and their accuracy depends on self-awareness as well as honest reporting. It seems intuitively plausible, then, that incentivized choices are superior to surveys, because the decisions that participants make have real consequences. But surprisingly little evidence supports the superiority of incentivized measures in terms of the consistency and stability of such measures or their correlation with actual risky behavior. Furthermore, little is known about whether preferences are inherent, stable attributes of an individual, like personality, or whether they evolve over the life cycle or in response to specific types of events. If preferences are malleable, the factors affecting preferences are only beginning to be explored.
Discussion of pros and cons
How economists approach risk aversion
The rational actor model is pervasive in economics, and has had some success invading other social sciences. In this model, an actor is assumed to have consistent preferences, and to make choices that best satisfy those preferences subject to existing constraints. Measuring individual risk preferences with incentivized tasks involves constructing sets of decisions for subjects to make. In order for a measure to accurately capture individual preferences, the decisions that make up the measure should have real financial consequences, so that subjects experience the associated gains and losses in payoffs. For example, given a choice between a risky, higher-expected-payoff gamble (say a 50/50 chance of winning $100 or $0) and a less risky, lower-payoff gamble (say, a 50/50 chance of winning $70 or $20), a choice of the latter indicates greater risk aversion. Theory guides the construction of the decision set so that choices reveal a subject's underlying risk tolerance. The decisions that subjects make in a constructed set of these tasks then demonstrate a “revealed” preference, and can be used to estimate risk tolerance in a utility-function framework.
As mentioned above, preferences are measured with two main types of procedures: survey questions and incentivized tasks. In the academic fields of psychology, sociology, and political science, the most common approach is the survey method. Hypothetical questionnaires are also the method of choice for the financial services industry. For example, risk tolerance might be assessed by asking a subject to report his past risky behaviors, or his perceptions of his own desire to take risks, and then constructing an index from a series of answers. Reliability and validity are assessed by testing how survey items collectively form a coherent measure, by repeating the test on different samples, and by examining the correlation between such measures and the behaviors they should predict. Thus, survey measures are fundamentally empirical, developed by finding questions that are correlated with one another that, intuitively, seem to capture the desired construct.
By contrast, economists have a preference for theoretically elegant measures (see e.g. [1]) but may fail to notice the difficulty facing a subject (lacking training in economics and mathematics) who must understand the incentive structure in a complex procedure in order to make good decisions [2].
Economists tend to prefer these incentivized measures on the grounds that, in the absence of incentives, subjects may misrepresent their preferences. Economists typically prefer so-called “incentive compatible” mechanisms that induce truth-telling. Indeed, without incentives there is little cost to misrepresenting one's preferences. One can imagine that a subject may lie to make other subjects or the experimenter think well of him, or even to bolster his own self-image. But when money is at stake in the decision, misrepresentation becomes more costly. Clearly, incentives are likely to make a substantial difference in the outcomes of preference-elicitation procedures under conditions where choices have a social-desirability element. To make this clear, measuring altruism is a case where social desirability could play a role. If a person were asked how kind or altruistic they are on a scale of 1–10, they might claim to be a great benefactor of others. But if that person were given $100 and asked how much they wish to donate to a charitable organization or another individual, his claimed altruism might evaporate. In the latter task, the person must actually give up money in order to indicate altruistic preferences.
It is not immediately clear that risk-taking has the same kind of social desirability, but there are instances where it is the case. Accordingly, men have been shown to take greater risks when observed by attractive women, for example.
Now, suppose one wants to measure an individual's propensity to take risks. A survey might ask subjects to self-report previous risky behavior, or rate their own preferences for risky options. Alternatively, incentivized elicitation procedures place subjects in carefully controlled settings where they make choices involving substantial stakes. In order to exhibit risk aversion, the subject must pay for it in the form of lower expected earnings. This kind of reasoning makes a skeptical social scientist believe that the incentivized task is more valid and reliable. However, there are very few studies that attempt to empirically test which measures of preferences are superior to others, or that even explore criteria by which to judge superiority.
Survey measures
Several survey measures can be divided into single-question measures and multi-question indexes of preferences. Both have been used extensively. The most widely adopted single question was developed for use in the German Socio-Economic Panel (GSOEP) survey, and asks subjects to rate themselves on a scale from 0 to 10 as to whether they prefer to avoid risks (=0) or enjoy taking risks (=10); a similar question is used in the UK Household Longitudinal Survey. These domain-general questions have been shown to correlate positively with self-reported behavior and preferences across many domains and with behavior in incentivized tasks [3], [4], [5].
The Zuckerman Sensation Seeking Scale, an early multi-question survey measure, assesses risk preferences with subscales for thrill and adventure seeking, experience seeking, disinhibition, and boredom susceptibility, and has been used in hundreds of studies. To explore further the variability of preferences across domains, a 2002 study subsequently developed a Domain-Specific Risk-Taking (DOSPERT) scale that explicitly considers several domains where risk is likely to matter [6]. It assesses both risk perceptions and risk preferences in the domains of financial decisions (separately for investing versus gambling), health/safety, recreational, ethical, and social decisions. This survey uses rating scales asking subjects to indicate how risky they believe a given activity to be (e.g. betting a day's income at the horse races), and then to report how likely they are to engage in the activity. The answers are combined to create domain-specific and general indices.
Incentivized measures
Several incentivized measures have been developed using different strategies for determining an individual's degree of risk aversion. These methods include valuation tasks, choice tasks, and framed incremental tasks.
Valuation
The earliest example of a valuation task for assessing risk aversion adapts the method developed in [1] to elicit valuations of lotteries or gambles (the Becker-Degroot-Marschak (BDM) procedure). Subjects first state a value for a lottery in the form of a minimum price for which they are willing to sell the lottery back to the experimenter. A price is then generated (randomly) from a specified distribution (usually a uniform distribution between the low- and high-payoff prizes in the lottery). If the stated minimum selling value is below the randomly generated price, then the subject sells the lottery at that price, and the price constitutes the subject's earnings. If the stated value is greater than the generated price, then the subject keeps (and plays out) the lottery to determine her earnings. The method is intuitively appealing to economists; it is incentive-compatible for the subject to reveal her true value, because this value does not affect the potential selling price of the good. The typical BDM task would elicit values for a set of 10–20 lotteries, with subjects indicating a valuation for each lottery. These would then be used to estimate risk aversion.
Choice
Several tasks have been developed using choices between or among lotteries. In the most widely adopted of these, subjects make ten choices between paired lotteries (the Holt Laury (HL) procedure) [7]. The set of choices is structured to produce a relatively precise measure of a utility-function risk aversion parameter. Each binary choice is between a lower-risk and a higher-risk lottery, and each lottery has a high and a low prize; the probability of winning the higher prize in the lottery varies from 0% to 100%. The number of lower-risk choices is used as a summary measure, with a higher number indicating greater risk aversion. The full set of choices provides a lot of information, and can be used to estimate structural models of utility functions. This elegant measure of risk aversion is both theoretically sound and relatively easy for well-educated individuals to understand. It is very widely used in experimental economics, in both the lab and the field, and is limited only by its complexity, which may make it somewhat challenging for less-educated participants, as shown in [2].
A second, simpler choice method by Eckel and Grossman (B/EG), based on Binswanger's earlier work, presents subjects with a set of gambles that have an equal chance of a high or low payoff, and asks them to select their preferred option [8], [9]. This gamble is then played out to determine earnings. In the most current EG version specifically [2], subjects are given a choice of six lotteries, and the choices are structured so that the subject's preferred lottery indicates a level of risk aversion. The first lottery is riskless (the subject earns a fixed amount, because the high and low payoffs are equal). The next four lotteries increase in expected value and variance. The final lottery has an expected value that is equal to the fifth lottery, but has higher variance; this lottery is preferred only when a subject has risk-seeking preferences. This measure is appealing both for its simplicity, in that 50/50 gambles are relatively easy to understand, and because it allows risk seekers to be identified.
HL is an example of what is sometimes called a “multiple price list” (MPL) elicitation method [10]. The term makes sense when considering a simpler version of this task, where, instead of making a series of binary choices between lotteries, subjects instead make a series of choices between a single lottery and a sequence of prices. Consider a lottery consisting of a 50% chance of $100. Subjects might make a sequence of choices between a certainty of $10, $20, $30, $40, and so on, and the lottery—i.e. a price list. The point at which the subject switches over from the certain amount to the lottery gives a valuation of the lottery, and an estimate of risk aversion.
Framed incremental tasks
Another simple measure mimics an investment decision by giving subjects a fixed endowment, any part of which can be invested in a risky asset (the Gneezy Potters (GP) procedure, described in [11]). The asset pays off 2.5 times the investment with probability 50%, and zero otherwise. Risk aversion is gauged by the subject's allocation, with more investment indicating less risk aversion. This is a more natural task for subjects, and one that may be useful for that reason. On the other hand, this measure does not have a way to identify risk-seeking subjects, as a risk-neutral payoff-maximizer would invest everything in the asset.
Finally, the so-called “bomb” task frames risky choice as a kind of investment, but one where the subject specifies a number of boxes to collect, one of which might contain a bomb [12]. The subject earns a positive payoff for each box, but if any box contains a bomb, then earnings are zero. A very similar “balloon” task has subjects decide how many puffs of air to blow into a balloon, which might burst; again, payoffs increase in the number of puffs, but if the balloon explodes then earnings are zero. Risk attitudes are elicited by asking subjects to pre-specify a number of boxes or puffs. This task is intuitive, and has the advantage of maintaining a kind of “feel” of riskiness.
Comparing different measures
In general, it is easy to implement survey methods for measuring risk aversion, and subjects seem to be able to answer them without difficulty. The main shortcoming of survey methods seems to be that economists do not believe them, because there is nothing at stake for subjects. They also do not yield sufficiently precise information to allow estimation of parameters of a utility function, thus limiting their usefulness in structural modeling.
While both psychologists and economists think of risk aversion as a stable trait, which then applies across domains, it is easy to imagine someone who makes conservative investment choices but enjoys risky sports or health behaviors. For that reason, a domain-general measure may not predict well across domains. This is the appeal of the DOSPERT scale. It is worth mentioning, though, that even domain-specific measures are no panacea. While the scale has been tested on both student and adult populations, the relevant domains might vary across populations, so that the questions and even the domains may require tinkering to be population-relevant.
Incentivized measures developed by economists are both clever and promising, but their use is not without controversy. All of these tasks are based on the idea that people value money, but that the subjective value of an additional dollar of income falls as income increases. This assumption implies that a decision maker will be risk-averse. But risk aversion has a special definition in this context: it means that a decision maker will avoid variance; i.e. for a given average return, subjects will prefer gambles or investments with less variability in outcomes. A variety of ingenious tasks have been developed, some simple and some more complex, and there is a trade-off between the ease of comprehension and the sophistication or theoretical precision of the measure. Perhaps the biggest problem with these methods is that they often fail to take into account how people (who are not academics) make decisions. A method that might be very appealing to economists on theoretical grounds nevertheless has to be explained to subjects in a way that allows them to undertake the kind of reasoning that the designers expect of them. While college students do this relatively easily, it can be a major challenge for individuals with lower levels of mathematical proficiency or less familiarity with basic economic concepts. In addition, the question of whether these structured, incentivized methods are indeed more valid, in the sense of predicting risky behavior, is still being explored.
For example, beginning with valuation tasks, the complex, two-stage BDM method may confuse even relatively sophisticated subjects. While the method is designed so that subjects have an incentive to truthfully reveal the monetary value they place on a given gamble, they may not understand that this is true. Individuals are accustomed to stating prices as a starting point in a negotiation, and so may overstate their value for a lottery, leading to an inference of risk-seeking preferences. Indeed, this method routinely produces risk-seeking estimates of preferences, and no other method does this. It is possible that the second-stage price generation leads the subjects to believe that their valuations should depend on the range of possible prices, rather than just the properties of the lottery they are faced with, biasing valuations upward.
Choice tasks appear to avoid this problem. The choice methods mentioned above are relatively easy for subjects to understand, with the possible exception of HL. The average subject in a choice task is risk averse—some say “too” risk averse—and few subjects are risk-seeking. Comparing the choice-based tasks, HL and B/EG, the easier B/EG method is a coarser measure. Its small number of options means that subjects are categorized into fewer “bins,” but with perhaps greater accuracy given the narrower scope for misunderstanding. HL has a finer classification, but since it requires a greater understanding of probabilities, it may produce more errors, making differences across individuals and groups more difficult to detect. To make matters worse, the errors that result from more complex tasks may not only generate neutral noise, but also bias elicited preferences. Of course, MPL methods can be designed to be simple or complex, and to have few or many categories. They can also be presented in an iterative framework, where the grid becomes finer in subsequent tasks.
Finally, incremental tasks tend to be more intuitive for subjects because of their contextual frame. The frame can make the decisions easier for subjects to process cognitively than the more neutrally framed MPL tasks. The GP task is framed as an investment in a risky asset, and so is likely to be correlated with actual investment behavior simply due to the frame. The bomb and balloon tasks are also framed as real tasks, and have been shown to correlate with risk taking in a variety of fields (such as health or recreation). However, behaviors in these tasks require multiple rounds of computerized testing, and the strong framing of the decisions (involving bombs or balloons) may mean that behavior is less likely to be related to financial decision making or other important economic decisions. The domain of the task may affect its ability to generalize to behavior in other domains. Thus, framing may help lower the cognitive load for subjects making decisions, but at the same time limit their generalizability [13].
An important puzzle emerges from studies that compare different methods. While no one has conducted a full meta-analysis comparing all elicitation methods, the partial attempts (such as [12] and papers in the Additional references online) yield an odd result: Different measures—whether survey- or task-based—seem to give different answers. Not only are the levels different, but the correlations across measures tend to be quite low. To clarify, suppose that a group of subjects completes several different incentivized and survey measures of risk aversion. Some time later the same subjects return to repeat the tasks. Because all of the tasks are designed to measure the curvature of the utility function, the measures should all give the same answer, both across measures and across time. But there is considerable evidence that they do not.
A 1962 paper (see [2] for details) was the first to show that risk tolerance of student subjects varies depending on the elicitation procedure and response mode. This study was followed by a number of others in the 1980s and 1990s documenting the inconsistency across measures. The profession has been slow to get the message. The typical response by non-experimental economists is that something must have been wrong with the experiment, yet the result has been replicated again and again. One might well ask whether this evidence means that economists’ conception of risk aversion needs a little work.
A study from 2010 conducted several different risk-aversion measures with a sample of about 1,000 Canadian adults [2]. The B/EG and HL methods were implemented, both of which involve choices among lotteries, and so have similar response modes, with similar stakes averaging about $75. A highly significant correlation of 0.38 between the two measures was found. While this is encouraging, the correlation is significantly different from 1, the value it should take if the two tasks are measuring the same underlying utility function.
Individual differences
Risk preferences vary systematically by gender and age, as well as cultural environment. Women are, in general, more risk averse than men, though the difference is not large. The gender difference is found across measures, though it is stronger in some than others. The gender difference in risk preferences has been associated with choice of college major and occupation; gender differences in investment portfolios and retirement savings are attributed to differences in risk aversion. Older decision makers are often more risk-tolerant in investments, but that is hard to separate from differences in wealth. It seems clear that wealthier individuals should be more risk tolerant, though this is perhaps attributable to a greater capacity to tolerate losses than a difference in risk aversion per se.
There is accumulating evidence that cultural differences importantly shape preferences [4]. Evidence is also emerging that women and men in matrilineal cultures switch roles, with women taking more risks than men. These studies suggest that culture may play an important role in shaping risk tolerance, and downplays the nature portion of the perpetual nature versus nurture debate.
Cognitive ability may also play a role in shaping elicited risk preferences in incentivized tasks. Many studies find that greater risk aversion is associated with lower cognitive ability. A recent study reviews these findings and presents new data showing that correlation between risk aversion and cognitive ability may be a kind of illusion caused by the types of errors that are made with different elicitation methods [13]. This reinforces the idea that care must be taken in preference-elicitation to ensure that subjects are cognitively capable of understanding the tasks. The evidence suggests that if subjects fully understand the task, cognitive ability is unlikely to matter [2].
Limitations and gaps
If preferences are stable behavioral traits, then they should be stable not only over domains, but over time. However, evidence from a number of studies indicates that risk preferences can change over time, in two ways. First, exposure to a different environment can gradually alter preferences. Second, a change in risk preferences may occur in the wake of a major life event, such as a natural disaster or a financial crash, and, further, that short-term and long-term consequences may also differ. Evidence further suggests that, immediately following a negative event, people appear to be risk-seeking, as they attempt to regain their pre-disaster reference point. After the dust settles and outcomes are resolved, however, people become more risk averse in the wake of a major negative event. Further theoretical development and testing is needed to understand the evolution of preferences.
The external validity of incentivized risk measures in particular settings has also received some attention, focusing on specific domains such as health and personal finance. The main shortcoming of these studies is that they tend to take a piecemeal approach, testing only one measure against a related life activity, and thereby lack general relevance.
External validity of survey measures has also been the target of considerable research. Two recent surveys incorporate a battery of risk aversion items and tasks into nationally representative surveys and relate these measures to self-reported risky behavior across a variety of domains. The first is part of the preference module of the GSOEP. An evaluation of this survey concludes by advocating the use of simple, survey-based methods for preference elicitation in large-scale surveys, arguing that the incentivized MPL measures are not worth the time and cost to implement [4]. Another study reports a similar exercise for the UK Household Longitudinal Survey [5]. It directly compares HL and B/EG elicitations along with the domain-general and domain-specific survey items. These are compared with each other, and with a one-year later elicitation. All of the measures show strong test-retest reliability a year later, and all are significantly correlated with each other at a point in time. However, tests of external validity across a variety of domains are decidedly mixed. No measure dominates the others, and the survey questions do no worse than the incentivized measures in most settings. This is not particularly good news for economists who advocate the use of incentivized measures, but it does suggest that low-cost alternatives may have reasonable usefulness as measures of preferences.
In sum, despite many studies, the jury is still out on whether and when incentivized measures are worth the additional time and effort required to use them.
Summary and policy advice
The question naturally arises as to which is the “best” way to elicit risk preferences. Is there a superior method? Does it depend on the characteristics of the study's population [2] or on the domain under consideration [6]? Does the level of incentives matter for the accuracy of the measure? Are surveys as good as incentivized tasks?
Notable in the present discussion is a lack of strong support for the notion that incentivized measures are always superior. Indeed, there is considerable evidence supporting the cost-effectiveness and efficacy of survey measures. While it seems plausible that incentivized measures should be superior for measuring risk aversion, it is not clear from the evidence that this is true.
One important factor producing these results could be the measures themselves. Economists tend to design measures that are appealing from a theoretical perspective, but that do not necessarily take into account the ability of individuals without PhDs to fully comprehend the decisions they have to make. It may be the case that measures designed with human limitations as well as theoretical considerations in mind can do a better job of accurately eliciting preferences.
Another issue worth mentioning is that the concept of risk arising from expected utility theory is quite different from a layperson's idea of the meaning of risk. Economists’ concept of risk is closer to “variance-aversion,” and is far afield from the dictionary definition, which focuses on the danger or the possibility of a significant loss. It may be that study respondents naturally think of the lay version of the concept in answering survey questions, and fail to equate risk-taking with the kind of risk that occurs with choices among gambles that differ in expected value and variance. If this were true, then it would be no surprise that the survey questions do a better job of predicting risky behavior in a variety of domains. Variance-aversion may not play a large role in the types of risky choices that individuals face in their everyday lives.
That said, some attention should be paid to the notion of using the right tool for the right job. It seems pretty clear that the assessment of risk-as-variance-aversion at the individual level is likely to be relevant for decision environments that involve financial decisions and investing. Adapting and using incentivized tasks for this purpose makes a lot of sense. Assessing willingness of voters to pay to reduce risks of various types in the policy arena may also be a good venue for the application of incentivized elicitation methods. But for many other applications, low-cost, practical alternatives should be given serious consideration. Carefully designed survey measures of individual preferences can be at least as effective, and much less costly to implement, than their theoretically elegant, incentivized counterparts.
Acknowledgments
The author thanks an anonymous referee and the IZA World of Labor editors for many helpful suggestions on earlier drafts. Previous work of the author contains a larger number of background references for the material presented here and has been used intensively in all major parts of this article (see [2], [9], and the Additional references online).
Competing interests
The IZA World of Labor project is committed to the IZA Code of Conduct. The author declares to have observed the principles outlined in the code.
© Catherine C. Eckel