Elevator pitch
Using Google search activity data can help detect, in real time and at high frequency, a wide spectrum of breaking socio-economic trends around the world. This wealth of data is the result of an ongoing and ever more pervasive digitization of information. Search activity data stand in contrast to more traditional economic measurement approaches, which are still tailored to an earlier era of scarce computing power. Search activity data can be used for more timely, informed, and effective policy making for the benefit of society, particularly in times of crisis. Indeed, having such data shifts the relation between theory and the data to support it.
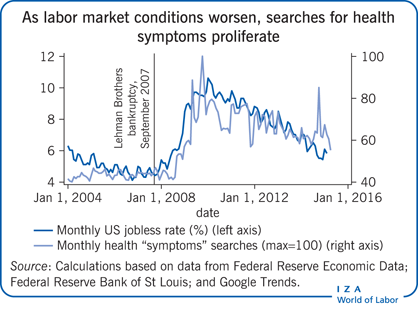
Key findings
Pros
Search activity data express the demand for a wide range of information and reveal information about the state of the searchers.
The data allow investigations in multiple combinations of space, time, and context on all facets of the human condition, enabling multidisciplinary research.
The data—seen as an involuntary, indirect panel survey data—can be more revealing than classical survey data.
The data are high in frequency and available almost in real time, allowing for the timely detection of breaking trends in times of crisis.
Cons
The data are proprietary and made available in aggregate form only.
The methodology comes without versioning and is not sufficiently described, obstructing acceptance.
The geographic distribution of search data has limited usefulness since IP addresses cannot always be properly located.
Keyword significance may change in time and across regions, reducing usefulness.
The Google page rank, as it changes in time, may affect supply and demand, as could various forms of censorship.
Author's main message
Google search activity data express the demand for information by topic from internet users around the world, so they contain insights into a large part of the human condition. The data are suitable for multidisciplinary research on individual behavior. They will become an indispensable part of modern policy making, especially in times of crisis. Google Trends data are an example of how corporate and societal interests may converge. But most of this type of digital data is proprietary and available only as aggregate data. A lot of work has to be done before social science researchers and policymakers can use this type of data effectively.
Motivation
While methodological progress in economics has been ongoing in recent decades, the same cannot be said about economic measurement, which remains tailored more to past technological capacity scarcities than to present capabilities. Even today, many indispensable economic indicators are still being estimated, revised several times, and published with a lag, depriving us of a better foundation for decision-making, possibly at great societal cost.
With most of the urban population of advanced economies linked through information and communication technologies, ideas, products, and economic behaviors are being transmitted as contagions, invading all aspects of our lives. The need for timely and accurate measurements is thus becoming increasingly necessary—and increasingly possible.
Internet search data are a first, although imperfect, example of progress in timely and accurate measurement. The data are of high frequency and can be delivered without lags. They can also be global, encompassing many aspects of socio-economic activity, and thus may be more useful for multidisciplinary research.
Discussion of pros and cons
Save data first—and ask questions later
The past scarcity of computing resources limited the amount of data that could be saved and processed in an economically viable and timely fashion. By using survey methodologies (with random sampling), researchers constructed representative but nonetheless manageable samples to investigate topics of interest. Researchers first articulated a hypothesis as a way of formalizing an idea about how the data might be related, using theory, scientific hypothesis, and intuition; drafted a questionnaire to capture the necessary variables; and then executed the survey on a randomly chosen sample. Following data collection, researchers focused on managing the error during extrapolation of the results from the sample to the original population universe. Depending on how well the analysis verified the hypothesis, researchers needed to repeat the process, altering the hypothesis and thus the survey design. In addition, whenever the hypothesis and the results diverged, it was not always clear whether the problem was a lack of adequate representativeness of the sample or simply a need for a new hypothesis. This method is slow and error-prone.
From 1956, when IBM marketed the first file-saving device, to August 2011, prices for digital data storage were cut by a factor of 35 million. As the prices of storage plummeted and computing power became affordable in a scalable manner, more degrees of freedom became possible in social science research and the way researchers conduct research.
Today, with respect to capacity, researchers can save data first and ask questions later because the marginal cost of saving an extra unit of data is negligible and new techniques and computing power allow the processing of large amounts of data. This ability to store and process data cheaply is not exactly the end of theory (as touted by some), but it is an important shift in the relationship between theory and data. Data can now guide the formulation of theory. For example, by using machine-learning techniques or by allowing a large number of models to compete with each other, researchers can guide the search for meaning in the data.
Progress in computing thus enables a shift from keeping low-frequency snapshots of the past to taking reels of film of the past and rewinding and reviewing the film over and over—and keeping the relevant snapshots at the desired intervals as methods improve and theories change.
Issues of data privacy, data protection, and law naturally arise, especially when there is no obstacle to saving “everything about everyone.” Attitudes on these issues, and thus normative decisions and eventually laws, differ from country to country and are influenced by a host of factors, including historical, sociological, and political.
A puzzling new privacy-related phenomenon is that, while people express a high sensitivity to the state collecting or reviewing data about them, they neglect to read the small print on privacy when they save their detailed, high frequency, and often intimate data into proprietary silos run by private social-media companies. Considerable multidisciplinary research will be necessary to sort these things out. Although the outcome of this process is still unclear, attitudes are shifting and will surely continue to do so.
Big data—on a large spectrum of the human condition
As more data become available, they take on the characteristics of “big data.” This type of data is not necessarily quantitatively big. It is big in the sense of containing not a sample but the entirety of the observational universe; in the sense that it is high frequency and often in real time; in the sense that it contains information on a large spectrum of the human condition; and in the sense that it covers a long time period and a broad global cross-section. These characteristics apply to the data provisioning tool that Google offers under the name of Google Trends, which is the main focus of this article.
In the years to come, this type of data will penetrate even central banking (such as the Irving Fisher Committee of the Bank of International Settlements), national archives (such as Eurostat), and government and company forecasting. Computational social science will enable a large, comprehensive, and multidisciplinary approach to understanding the human condition.
Internet searches—utterances worth investigating
An increasing number of socio-economic activities take place online, such as banking, entertainment, shopping, education, social networking, and information discovery. The internet is an ideal platform to effectively match demand and supply in a diverse array of markets (from labor markets and product markets to transportation markets and marriage markets). It has thus become a central resource for people not just in modern societies but also in less privileged societies, where landlines may be scarce but digital wireless telephony is prevalent. The study of internet activity in general will therefore be of increasing importance for the social sciences, particularly economics.
Internet search matches the demand for information to the documents that contain it. An individual’s interest in certain documents (and not in others) is defined by the set of search queries the individual uses to locate them. Such queries may be thought of as utterances worth being investigated, and their collection as an involuntary, high-frequency, irregular panel survey. The panel (longitudinal) aspect of these microdata ought to contain significant scientific insights, but that is out of reach for academic research for now because of concerns with data privacy, data protection, and proprietary interests.
The fact that individuals feel protected in the anonymity of their internet session implies that internet activity data are involuntary and thus contain no interviewer effects or biases. Assuming a suitable question, the answers from internet searches are sincere. This is very important for some types of research (such as [1]). Protecting the privacy of individuals in this context is important and raises many legal and ethical issues.
These utterances (internet activity) come in a high but variable frequency and form panel data of a sort, generating advantages and challenges. Since the data inherit the internet’s own geographic distribution, they also have huge cross-sectional potential. If researchers focus on certain types of properly chosen queries, they may be able to develop proxies for the documents the queries locate and thus measure proxies of the individual’s state. Reasonably defined aggregate measures of the intensity by which certain classes of searches are pursued may then be thought of as an indicator of the degree of proliferation of a certain condition.
Internet users are a sample selected from the total population, which becomes more representative as internet penetration increases. In many advanced economies, internet penetration is well above 90%, with the world average around 42%. For example, average penetration is 70% in Europe and 87% in North America. The type of socio-economic phenomena that might be detected range from emotional, psychological, and physical health [1], [2], [3], [4], [5] to economic conditions [6], [7], [8], [9], consumption [10], epidemics, and crime. That opens for the first time the possibility for a multidisciplinary holistic approach to social science and labor economics on a huge scale.
Search represents only data on the demand side in this market. Data on the supply of documents through internet platforms is equally important, but it is not easy to access those data now, which prevents any examination of the interaction of the supply of and demand for information.
Google Trends—a tool for data provisioning
In the summer of 2008, Google introduced a data-provisioning tool known as Google Trends (). It offers a public but limited view into relative internet search volumes for certain queries. Two examples can elucidate the informational value of this type of data.
The first example illustrates the degree of disruption this type of data may represent. Consider the analog library catalogue card of the past. Each library stored some basic metadata on every book in its collection on individual cards kept in file drawers. If it had been possible to get frequent measurements on the titles of books checked out and their bibliographic metadata, it would have been possible to monitor what people read and perhaps forecast such things as how many engineers or mathematicians there would be in four or five years. But because these data were fragmented, in analog form, and thus hard to compile and analyze, they could not be used effectively. With internet data, particularly Google Trends, this instrument is available on a global scale.
But since those data allow only relative measurements, a second example is due. Imagine being outside a soccer stadium and listening to the collective, incomprehensible chatter of some 50,000 spectators. You will never see when a goal is scored, but with some knowledge of the game, you may be able to interpret the sounds and thus to “hear” when one side senses danger or a scoring chance, when a missed scoring opportunity or a violent foul occurs, when a goal is scored, or when the home team or the visiting team attacks. Any sports fan has a built in “econometric model” that would “calculate” the final score based on sound data alone, without having viewed the game. By analogy, Google Trends gives us this type of access.
Many “nowcasting” (a combination of “now” and “forecasting”) applications measure the fit of a Google Trends time series to a more traditional variable in order to validate the data. An expected typical use of this type of data is to capture search terms that deviate from their mean and to use meaning to relate search intensity to some type of socio-economic activity for which only low frequency, lagged measurements are available from other more conventional sources. Google Trends data can provide early, high-frequency hints on breaking trends. Such an approach is necessary when one does not have access to the microdata or only a limited access to computing resources.
The rest of this section describes some aspects of Google Trends as a data-provisioning tool together with some original information for the researcher who wants to use this type of data.
Sessionization—to remove noise
According to the Google Trends team, search data are standardized in a process called “sessionization.” The precise modalities and parameters are not disclosed, but the process aims at reducing the noise from typing errors, rewrites, frivolous repetitions, and other random acts. For example, if one user is absent-minded and performs the same search 100 times back-to-back, this search is counted only once. All searches by each individual within a 24-hour period are collected and bundled into mini “search sessions” separated by time periods of idleness and contextual cohesion. These search sessions become the elementary object that contains meaning and thus the object of quantitative analysis.
With this information, researchers can select and count sessions based on whether the bundle of keywords that make up a session contains terms of interest. If, for example, a mini-session contains the words “symptoms of depression,” it can be inferred that the individual likely was looking for this type of medical information at the time of the session. For several but not all countries, based on the keywords in these mini-sessions, Google organizes search queries into contextual categories such as “travel,” “technology,” or “law and government.” No information is released on the method used to define these categories and the heuristics used to classify mini-sessions into a category.
Geotagging by country
A search session can also be geo-located using the IP address where the browser session originates. This geotagging becomes less accurate as one drills down to smaller regions, but it is highly accurate on a country level. When region, time, search, and Google category are specified, Google Trends will return weekly or monthly time series starting as far back as 2004.
One can query Google Trends for searches that contain specific terms, disjunctions, and complements. For example, “jobs -steve” will give you search intensity for the class of searches containing “jobs” without those that also contain “steve” (to avoid searches for the late Steve Jobs). But, for reasons that are easy to imagine, the query still will not yield purely labor market-related results. Volumes of search classes can be obtained relative to a category, so restricting “jobs” searches to the “classifieds” category may yield results that are closer to the labor-market context.
Comparative queries are possible: up to five search terms in a single geographic unit for any time interval or a single search term in up to five geographic regions in any time interval. Volumes are scaled and normalized. All data points of the time series are normalized by dividing the search term volume by the total search volume in the reference time interval (day, week, month).
This means that if, for a certain keyword K, there are Kᵢ number of mini-sessions (belonging to any number of users) in the i-th reference time interval containing the word K, and the total number of mini-sessions in the same time interval is Gi, then the search intensity is N(Kᵢ) = Kᵢ/Gi. The result will then be scaled by setting the maximum value equal to 100 and scaling the rest accordingly. This means that two search term queries in a comparative mode deliver relative volumes, which are nonetheless comparable with each other.
Sampling—not disclosed, not versioned, and subject to change
Sampling is involved in generating these numbers, and the results are robust for sufficiently high volumes. This sampling method is undisclosed, non-versioned, and subject to change without notice, which creates a validity issue for academic research. For low values, results may be unreliable, requiring caution in their use. Regression correlation results improve dramatically if the time series is drawn several times and the time series of point-wise averages is used instead of a time series that is drawn once [7].
Circumventing caching
Google caches the time series, however, making it difficult to draw the time series with different sampling within the same Google Trends session. This difficulty can be circumvented, however, as in [7]. To draw a certain time series for the keyword K n times, the user forms n keywords by taking the disjunctions “K + Rᵢ,” where the Rᵢ terms are random but mutually distinct strings that are unlikely to have occurred in real-world search. Since “+” is a disjunction in the Google Trends nomenclature, one is looking for mini-sessions that contain “K or Rᵢ.” Since Rᵢ never occurs, this is the same as mini-sessions that contain K. But for the Google Trends sampling mechanism, these are all different keywords and so they are sampled anew, thus circumventing the caching mechanism.
Search intensities of users
Note that Google Trends counts aggregate “searches” and not the people who perform them, which a priori does not tell whether a spike in the relative proliferation of a search term is due to a few power users or a large number of infrequent users. In other words, the volume as presently defined in Google Trends is simply the collective relative-search intensity of the Google users who are active in an observational time unit and geographic specification. Some things are known about the distribution of search frequency among the user population: 70% of searches are produced by 20% of users, and 92% of searches are produced by 50% of users [3]. So 50% of the least active users account for just 8% of the searches, but this information is not sufficient to extrapolate the share of people searching from the share of searches.
Examples of the use of Google Trends data
A growing literature is based on this type of data, with various thematic concentrations. Perhaps the best-known research example of presenting this data-provisioning tool to the world is Google Flu Trends (; ), which attempts to be an early-warning system of the prevalence of influenza. This research attempted to find out whether Google search terms could predict when a flu epidemic is about to break out [11]. Regression correlation analysis was used to test all keywords found in Google search and to determine which keywords to keep.
This method is typical of the big data approach, which sifts through large amounts of multivariate data to blindly detect important terms by simple correlation. That method is now the basis of Google Correlate (). It can be interesting to experiment with this tool and enjoy the erroneous correlations it can produce as well as the correct ones. With access to the necessary data and with adequate computational power, Google Correlate could be an important tool in developing intuitions and modifying the theories used to model the world. When access to data is more limited, keywords may be chosen based on intuition—a more theory-based identification strategy for keyword choice. One study applying such a semantic approach forecasts German unemployment using searches for the top job search engines, the German Unemployment Office, and the well-established German practice of “short-time work” (a work-sharing program) [6].
Another study examined two classes of searches. One class contains the word “symptoms” and is a proxy for medical self-diagnosis, and the other class contains the words “side effects” and is a proxy for medical treatment [1]. Google Translate was used to translate the terms into each country’s language. The study used Google Trends to see the intensity of searches for the translated terms in each country. Spikes in these searches were observed during the great recession in all G8 countries (Figure 1).
Another study monitored searches containing the word “hardship letter” to nowcast deteriorating economic conditions in the US housing market [7]. A homeowner who is unable to make an upcoming mortgage payment uses a hardship letter to inform the bank and request some leeway in mortgage payments. Searches containing the words “hardship letter” express the demand for pages that advise how to write a successful hardship letter. (The reader can verify that typing “hardship letter” into Google search will return “hardship letter samples” and “hardship letter examples” as auto-completions.)
These searches thus signal the first time a homeowner externalizes a deteriorating financial state and so are an early indicator of economic distress. The searches proved to be a good indicator of mortgage delinquency. Figure 2 is a joint plot of two time series. One is the percentage of delinquent mortgages, as reported in press releases of the Mortgage Bankers Association, a quarterly time series published with a six-week lag, and the other is the weekly time series of the Google searches for “hardship letter.” The graph shows a somewhat noisy weekly picture of upcoming mortgage delinquencies during the Great Recession.
Limitations and gaps
Google Trends data are a powerful new tool that deserves a place in the toolbox of social scientists. It nonetheless comes with deficits, the most important of which are: it allows only an aggregate look into what ought to be rich behavioral microdata; it comes with an insufficiently described methodology; it lacks versioning; it works well only for large search volumes; it is representative only where internet penetration is sufficiently high; although these data are geotagged, IP-based geolocation is accurate only at a country level; and access to these data are at the discretion of Google, whose commitment to providing the data may change (as far as this author knows, neither Bing nor Yahoo offer similar data).
In addition, very little data are available on the supply of documents through online platforms. Future research would benefit from merging data on the supply of documents by topic with internet search data (demand for the documents). The interaction of the supply and demand for information ought to inform the conclusions that can be drawn when interpreting internet searches. A trivial example is to think about extremes. If there is no supply of documents on a certain topic, then matching queries will ultimately perish since the population of internet users will eventually stop performing fruitless searches. But if there is an overabundance of documents on a topic, that supply alone may generate queries for the topic as searchers randomly stumble on such documents and become curious for more. Or it may generate no queries at all if the documents can be found everywhere, so that no searching is necessary.
These deficits, combined with the fact that most social scientists are trained in statistical methods that originate in an era of scarce computing, hinder the wider adoption of Google Trends data as a serious tool for social science research, making it simply another source of experimental data for investigating possibilities rather than establishing useful tools.
Summary and policy advice
Google Trends data are an example of how business interests may be applied to achieve societal common good. This type of data should be researched by social scientists, methodologists, and data privacy experts. And it should become part of the arsenal of policymakers for nowcasting and understanding breaking trends.
However, governments will have to encourage or even legislate for some kind of corporate good practice (for example, in the form of a data tax) to motivate firms with large amounts of data in their proprietary silos to open up the data in aggregate form for the benefit of society, while also protecting their legitimate corporate interests and privacy concerns.
Such data may be more accurate and timely than official data. In an economic crisis, for example, looking at official epidemiological data will give the registered incidents but not the whole rapidly evolving picture, in particular it will miss individuals who are no longer able to afford medical treatment. But Google search would capture the attempts to seek information on alternative treatment.
Getting an accurate reading on current trends without a lag is possible with today’s technology and should be a high priority issue for policymakers.
Acknowledgments
The author thanks three anonymous referees and the IZA World of Labor editors for many helpful suggestions on earlier drafts. The author also acknowledges the gentle but persistent reminders of the IZA World of Labor editorial staff.
Competing interests
The IZA World of Labor project is committed to the IZA Guiding Principles of Research Integrity. The author declares to have observed these principles.
© Nikolaos Askitas