Elevator pitch
Over recent decades, Western countries have admitted many immigrants from non-traditional regions (e.g. Philippines, India, China), which has coincided with poor economic integration. Language proficiency is an important determinant of economic integration; in addition to being a component of human capital, it plays a key role in facilitating the transmission of other components of human capital. Examining the strengths and weaknesses of objective and subjective measures of language proficiency is crucial for good integration policy, as is understanding the relationship between these measures and earnings, a key indicator of economic integration.
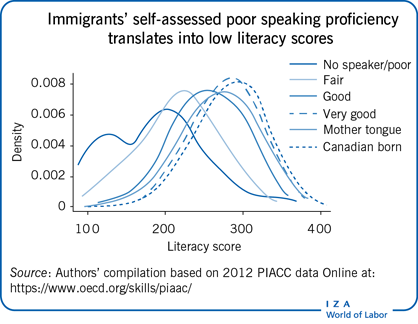
Key findings
Pros
Language proficiency is a component of human capital and facilitates the transmission of other components.
Differences in objective language proficiency explain most of the immigrant/native-born earnings gaps.
Self-assessed measures of language proficiency are more readily available and contain a large amount of information related to earnings.
Skills can be used to compare human capital across individuals, avoiding issues related to education quality and work experience.
Cons
Objective measures of language proficiency are uncommon; more common subjective measures contain measurement error and/or capture different skills.
Test scores reflect a mix of cognitive ability and language proficiency since many immigrants do not take tests in their mother tongue.
Available measures of literacy and numeracy are highly correlated, implying they may not capture the skills they are intended to test.
Objective tests are often not challenging enough for high-skilled respondents.
Literacy and numeracy do not capture the full range of skills needed to succeed in the labor market.
Author's main message
Language proficiency is key to the economic integration of immigrants. Most research relies on self-assessed measures because objective test scores are not available in widely used data sets. However, while there is a great deal of overlap between these measures, self-assessments may contain measurement error and/or capture different skills than objective test scores. Current measures therefore do not capture the full spectrum of language-related human capital. Better tests are thus needed, and should be better integrated into immigrant selection criteria.
Motivation
Over the past several decades, Western countries have been admitting a large number of immigrants from developing countries and, in many cases, they have experienced poor economic integration. For countries like the US and Canada, which have a comparatively long history of immigration, recent cohorts have experienced worse labor market outcomes at entry relative to earlier cohorts, as well as an increase in the number of years needed to reach parity with the native born, if ever. In the 1990s, Canada increased its emphasis on education when selecting economic immigrants. This resulted in the portion of new immigrants with a university degree growing from around 25% to over 50% [1]. Despite these efforts, recent immigrants still struggle in the labor market. Empirical evidence has pointed to language proficiency as a key factor in the lack of economic integration. Therefore, it is important to establish what is currently known about the language proficiency of immigrants and its role in economic integration, as well as the relevant limitations. This knowledge is central to designing data, research, and policies to better choose immigrants and assist in their economic integration.
Discussion of pros and cons
Economic integration over time
A growing number of immigrants from developing countries has coincided with poor economic integration in Western nations. For example, Figure 1 outlines the case of male immigrants in Canada [1]. It shows the relationship between years since immigration and weekly earnings for different cohorts of immigrants (calculated in terms of percentage differences). The line at zero indicates earnings equality with the Canadian born. Points above the line indicate an earnings advantage for immigrants, and points below indicate an earnings disadvantage. Each successive cohort generally has lower earnings at entry than the previous one, and the time needed to catch up to the Canadian born has greatly increased. The authors also show estimates in which they condition on educational attainment, then add controls for source country and self-assessed language proficiency. There have been greater levels of educational attainment among recent cohorts, as well as lower returns to it, so conditioning on education further increases the earnings differential and shifts the curves downward. When adding controls for source country and language proficiency, within-cohort differences are greatly compressed [1]. This likely occurs because recent cohorts’ source countries are more often from non-traditional regions (e.g. the Philippines, India, and China), which is highly correlated with language proficiency. Indeed, the mother tongue of many immigrants is different than that of the host country, making it difficult to transfer the human capital they acquired prior to arrival.
While this article focuses on language proficiency, the poor economic outcomes outlined in Figure 1 are driven by a host of factors, including the transferability of human capital. Past research has relied on variants of the so-called Mincer equation, using education and work experience as measures of human capital, to explain differences in earnings. Although educational attainment and years of schooling are available in most data sets, there are important quality differences that reduce the accuracy of these measures in capturing an individual's human capital. Some argue it is unreasonable to assume that a year of schooling will yield the same skills across countries [2]. This is especially important when considering the foreign-acquired education of immigrants. Past work has tried to address this issue by including source country quality of education in the Mincer equation. For example, there is evidence that quality of education, as proxied by international test scores, is important in explaining the Canadian rate of return on foreign years of schooling [3]. Though source country quality of education captures some of the differences in human capital across immigrants, there remains variation in the quality of education within each source country. This is true even when restricting the sample to immigrants from a specific region; students develop different skills even within a given classroom and institution. Therefore, educational attainment and years of schooling do not capture the vast heterogeneity in skills. Similarly, work experience is intended to capture on-the-job human capital accumulation. However, in addition to the lack of direct measures of work experience in most data sets, there is variation in the human capital contained therein. Different jobs do not provide the same human capital accumulation and, even in a given job, individuals develop different skills.
Objective tests to measure skills
It is argued that objective tests “provide a common metric for measuring skill differences across countries, and they provide a method for testing directly the approaches to modeling growth” [2], p. 34. This is true within countries as well; objective tests provide a way to compare the human capital of individuals, avoiding issues related to quality of education and work experience. Some data sets contain objective test scores, such as the 2012 Programme for the International Assessment of Adult Competencies (PIAAC), which includes literacy, numeracy, and technology skills and allows for comparisons across 23 countries. Many researchers interpret literacy and numeracy scores as measures of cognitive ability [4], [5].
However, skill measures are not without limitations. First, literacy and numeracy scores do not capture the multitude of language and quantitative skills that are necessary for labor market success. Likewise, they are only two of the many skills required. It has also been noted that objective test scores are highly correlated across skills. For example, there is a strong correlation between prose, document, and quantitative literacy using the International Adult Literacy Survey [6]. Similarly, using data from the 2012 PIAAC, a strong correlation is found between literacy, numeracy, and technology skills (e.g. the correlation between literacy and numeracy is approximately 0.9—with one being the highest possible value—even when the sample is disaggregated by gender and immigration status). This implies that objective tests are too similar in terms of the skills they assess.
There are also concerns that test scores may not adequately capture the upper part of the skill distributions due to top-coding, which occurs because the tests are not challenging enough to rank people above a certain skill level [7]. It is common in countries like Canada and Australia to select immigrants for high-skill jobs, and top-coding may not allow for assessing differences between them. While insignificant differences in the upper part of the distributions may be due to immigrants having similar skills as the native born, they could instead reflect that tests are not challenging enough to identify differences. On the other hand, top-coding may not be binding because of the variance in language proficiency across immigrants [7]. In other words, objective test scores may still provide information about their labor market potential, despite top-coding, but it will be related to language proficiency and not just the skill being tested.
This suggests another limitation. To the extent that test performance depends on language proficiency, literacy and numeracy scores may not accurately reflect cognitive ability. This is particularly true for immigrants, many of whom do not take the tests in their mother tongue. There is evidence that, for workers with a university degree, test scores do not affect earnings of the native born, but they provide an important return for immigrants [7]. This implies that objective tests likely capture a mix of cognitive ability and language proficiency.
It follows that language, in addition to being a component of human capital, is also key to facilitating the transmission of other components [8]. Indeed, there is evidence that the immigrant/native-born earnings gap is mostly eliminated after controlling for an objective measure of language proficiency [9]. However, objective measures of language proficiency are only observed in specialized data sets; most contain subjective measures, such as the US Census (e.g. “How well do you speak English?” with a handful of options ranging from “not at all” to “mother tongue”). Therefore, to better understand the importance of language proficiency for economic integration using widely available data sets, it is necessary to establish how well these subjective measures capture the full spectrum of language-related human capital.
Subjective versus objective measures
To accomplish the above, data from the 2012 PIAAC for Canada are examined as a case study. First, differences are outlined in an objective measure of language proficiency (literacy test scores) between immigrants and the native born. An examination is then conducted into how this objective measure relates to (subjective) self-assessed speaking proficiency. Finally, the implications for economic integration are assessed by examining the importance of these two variables for earnings.
The top two rows of Figure 2 present the mean objective language proficiency (measured via literacy test scores) for the Canadian born and immigrants, first for the full sample and then for female and male immigrants, respectively. On average, literacy scores are lower for immigrants. Scores for male immigrants tend to be slightly higher than those of female immigrants. This is likely due to Canada's reliance on economic immigrants, whereby male immigrants are more likely to be the principal applicant and assessed based on their human capital at the time of the application [10]. As shown in the literature and examined below with more recent data, these differences in objective language proficiency are key to economic integration [9]. However, only a handful of specialized data sets contain such measures. Instead, the majority of research has relied on self-assessed language proficiency, which is observed in most widely used data sets, such as the US Census.
The bottom panel of Figure 2 presents the mean objective language proficiency of immigrants (measured via literacy test scores), further disaggregated by their self-assessed ability to speak the language in which they took the objective test. Immigrants who self-assess as “very good” have similar literacy test scores as the Canadian born, while immigrants who self-assess as “mother tongue” have scores that are slightly lower. The estimates include immigrants who arrived as children and were educated in Canada, so these individuals are likely included in the “very good” and “mother tongue” categories. Literacy test scores decline monotonically for the remaining three self-reported categories.
However, looking simply at mean differences may hide important information about the similarities and differences between groups. Figure 3 thus presents literacy score densities for immigrants and the Canadian born. Consistent with existing evidence, large differences between groups are found using more recent data; the distribution of objective language proficiency (literacy test scores) for immigrants is to the left of the Canadian born [9]. While most of the distributions have common support, this is not true in the tails. In the upper part of the distribution, the Canadian born are to the right of immigrants, while the bottom part indicates that a moderate proportion of immigrants have literacy scores below the lowest scores of the Canadian born.
The Illustration further examines literacy score densities for immigrants, disaggregated by their self-assessed ability to speak the language in which they took the objective test. Consistent with mean differences shown in Figure 2, densities are shifted to the left for lower self-assessed groups. However, the density analysis indicates a large overlap between groups. This could be capturing a combination of two factors. First, this may point to measurement error in self-assessed language proficiency, since one would not expect overlap between groups. Second, it may indicate that self-assessed speaking proficiency captures different skills than literacy test scores. If the latter is true, then not everyone with strong speaking skills will necessarily have strong literacy skills and vice versa. This would suggest that relying on one dimension of language proficiency may not capture all aspects that are important for human capital.
The data used here do not contain both self-assessed speaking and writing proficiency; however, using another data source, the 2003 US National Assessment of Adult Literacy, it is found that they are highly correlated (with a value of 0.813). Yet, the wide distribution of literacy scores among immigrants who took the test in their mother tongue and the fact that it overlaps with non-mother tongue groups suggests that narrow self-assessed categories do not capture differences in language proficiency.
Earnings differences and self-assessed speaking proficiency
Figure 4 presents earnings differences for immigrants relative to the Canadian born, and then shows those same differences disaggregated by self-assessed speaking proficiency. In the top panel, when controlling for age and region of residence, the immigrant disadvantage is around 17% for female immigrants and 11% for male immigrants. This disadvantage grows if excluding immigrants who arrived as children.
In the second panel, when controlling for objective language proficiency (literacy test scores), the difference for female immigrants is reduced by around 10 percentage points, or more than 60%. For male immigrants, there is no longer a measurable difference relative to the Canadian born.
In the third panel, controls for objective language proficiency (literacy test scores) are removed and are replaced by controls for educational attainment. The earnings gaps increase relative to the first panel; they are around 21% and 17% for female and male immigrants, respectively. This occurs since, on average, the education obtained by immigrants prior to arrival is less valued in the Canadian labor market. This is particularly true for more recent cohorts who, in addition to having higher levels of education, also tend to be from non-traditional regions, making it more difficult to transfer their human capital. These gaps may be exaggerated if there are quality differences between foreign-acquired education and that acquired in Canada. The current analysis does not include controls for quality of education or source country, though both are important for the returns to education and economic integration [3].
The last panel presents results after controlling for both literacy test scores and education. The gaps shrink to 15% and 11% for women and men, respectively.
Figure 4 also shows that when immigrants are disaggregated by self-assessed speaking proficiency, large differences can be seen in earnings across groups. With basic controls (age and region of residence), there are no distinguishable differences in earnings for immigrants who self-assess as speaking “very good” or “mother tongue” versus the Canadian born (except male immigrants who took the test in their mother tongue). Gaps increase monotonically for those who speak “good,” “fair,” and “cannot speak/poor.” The gap for the latter is almost 50% for female immigrants and 40% for male immigrants. These estimates suggest that a large amount of information is contained in self-assessed speaking proficiency. However, it should be cautioned that this measure may be highly correlated with other variables, such as source country. It is hard to disentangle these human capital variables, especially when they are very broad.
When controlling for objective language proficiency (measured via literacy test scores), important reductions in the earnings gaps are observed. Gaps for the lower three language groups shrink by 35% to 70%, although they are still statistically significant for female immigrants and the “fair” group of male immigrants. The fact that statistically significant differences remain indicates measurement error in self-assessed speaking proficiency and/or important differences between self-assessed speaking proficiency and literacy test scores. In the third panel of Figure 4, when controlling for education instead of literacy scores, the earnings gaps again grow compared to the first panel. Controlling for both education and literacy reduces the gaps, but they remain for most of the self-assessed groups.
Overall, it appears clear that objective language proficiency is an important determinant of earnings and key to addressing the immigrant/native-born gaps. Further, important differences emerge between the self-assessed groups that do not fully disappear after conditioning on literacy test scores.
Limitations and gaps
The available objective and subjective measures of language proficiency are not enough. For example, literacy scores are highly correlated with other skills (e.g. numeracy). This may be due to a lack of difficulty in the tests, which limits the extent to which they capture higher-order cognitive ability and language proficiency. Better measures of language proficiency are needed in widely used data sets to advance research and policy related to the economic integration of immigrants.
Moreover, while objective tests are rare, they are almost always cross sectional. This prevents researchers from considering when skills are acquired and how they evolve through education and work experience. For immigrants, in particular, it does not allow researchers to examine how they evolve from the point of arrival.
Summary and policy advice
Language proficiency is key to the economic integration of immigrants, both as a component of human capital and because it facilitates the transmission of other components. Empirically, language proficiency is highly correlated with other skills though, such as numeracy. This suggests that tests are similar in terms of what they assess or that language proficiency affects test performance across skills, making it difficult to assess the relative importance of each for economic integration. Moreover, it could also be that tests are not difficult enough to capture skills and language proficiency in the upper part of the distribution.
To assess the relationship between language proficiency and economic integration, most research has relied on data sets that contain self-assessments, not objective test scores. However, there seems to be a large amount of overlap in literacy test scores for self-assessed speaking groups, which implies measurement error in the latter, or that self-assessments are capturing different skills. While both objective and subjective measures contain information pertinent to earnings, gaps remain for self-assessed groups when controlling for literacy test scores.
Many countries have recognized the importance of language proficiency and have integrated language tests into their immigrant selection criteria. For example, Australia made language tests mandatory for prospective immigrants in the 1990s. This policy improved language proficiency relative to earlier cohorts, as well as relative to Canada and the US, in which rigorous language tests were not in place over the same period [7].
However, Canada, for instance, has recently made efforts to better incorporate language tests into its immigrant selection criteria. Most recently, the Express Entry program requires economic immigrants to undergo tests that assess listening, speaking, reading, and writing proficiency. The level required to pass depends on the program to which the immigrant is applying, as well as the designated occupation. Still, better data are needed to accurately assess the effect of these types of policies, and policymakers should adjust the programs accordingly.
Acknowledgments
The authors thank an anonymous referee and IZA World of Labor editors for many helpful suggestions on earlier drafts. Financial support is gratefully acknowledged from the US Department of Agriculture, National Institute of Food and Agriculture, Hatch project 1016011. This analysis was conducted at the Atlantic Research Data Centre, which is part of the Canadian Research Data Centre Network. The services and activities provided by the Atlantic Research Data Centre are made possible by the financial or in-kind support of the Social Sciences and Humanities Research Council, Canadian Institutes of Health Research, Canada Foundation for Innovation, Statistics Canada and Dalhousie University. The authors further thank Heather Hobson and Daniel Feldman for research assistance.
Competing interests
The IZA World of Labor project is committed to the IZA Code of Conduct. The authors declare to have observed the principles outlined in the code.
© Angela Daley, Min Hu, and Casey Warman