Elevator pitch
The information technology revolution has had dramatic effects on jobs and the labor market. Many routine and manual tasks have been automated, replacing workers. By contrast, new technologies complement non-routine, cognitive, and social tasks, making work in such tasks more productive. These effects have polarized labor markets: While low-skill jobs have stagnated, there are fewer and lower paid jobs for middle-skill workers, and higher pay for high-skill workers, increasing wage inequality. Advances in artificial intelligence may be accelerating computers’ ability to perform cognitive tasks, heightening concerns about automation of even high-skill jobs.
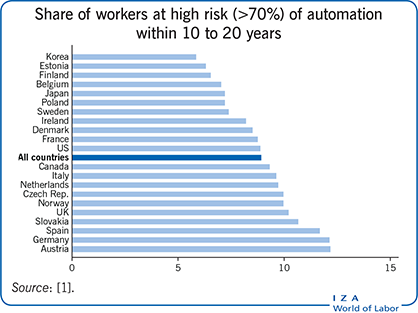
Key findings
Pros
Technology complements many tasks, increasing productivity, quality, and innovation.
Big data and machine learning are increasing machines’ ability to perform cognitive, physical, and even some social (language) tasks.
Greater access to data, analysis tools, and telecommunications allows many workers to focus more on social interactions, collaboration, continuous improvement, and innovation.
Technology makes many high-skill jobs more intrinsically motivating, enabling more tasks, skills, and decentralization.
Cons
Machines substitute for humans in many manual and routine jobs.
The pace at which machines gain the ability to perform cognitive tasks is faster than in the past, making adaptation by workers more difficult.
Labor markets have polarized and inequality has risen, with relatively less demand for mid-skill workers and increased value for high-skill workers.
Technology makes many middle-skill jobs less intrinsically motivating, with fewer tasks and skills, and more centralization and monitoring.
Author's main message
Technology has opposing effects on jobs. It facilitates automation, creating fewer and less motivating middle-skill jobs. Conversely, it complements social and innovation tasks, creating more interesting low- and high-skill jobs. This causes labor market polarization, “hollowing out” demand for middle-skill jobs, and increasing wage inequality. Some claim that computers will soon replace many workers as artificial intelligence advances. Others are skeptical, as previous technological advances did not lead to mass unemployment. Policymakers should encourage technology that complements employees’ work, and should foster education and training that help workers adapt to change.
Motivation
Since at least the Luddite movement of 1811–1816, the effects of new technology on jobs and employment have generated great controversy. In the last few decades, enormous improvements in information and telecommunications technologies (ICT) have had dramatic effects, benefiting some workers, but eliminating others’ jobs.
The current debate focuses on two issues: How far, and how fast, will job automation proceed? Recent advances in artificial intelligence methods are pushing past previous limits on the types of tasks that can be automated. Machines increasingly perform cognitive tasks, use natural language, and have greater dexterity and mobility. Some observers claim a high fraction of jobs are at risk of being automated, including high-skill jobs for the first time, potentially leading to large-scale unemployment. Others are more skeptical, noting barriers to technological advancement and implementation, and pointing out that labor markets have always managed to absorb new technologies in the past.
Discussion of pros and cons
How does technological change affect job design? Think of a job as a set of tasks that require various types of employee skills. New technology raises relative employee productivity in some tasks, and replaces employees in other tasks. Firms respond by changing job design—the mix of tasks assigned to workers—and subsequently their demand for workers with different skills.
Early technology tended to increase productivity of low-skill manual laborers by providing better tools, machinery, and cheaper raw materials. This was reflected in gradual mechanization of agriculture, and the movement from artisan to factory manufacturing in the late 1800s [2]. However, by about 1910, new technology began favoring middle- and high-skill workers. Factories shifted to electric power, which facilitated batch or continuous production methods, and assembly lines. Factory foremen, machinists, and managers became more productive, overseeing more resources and output. Meanwhile, many manual jobs were mechanized.
This is an early example of a general point. Technology sometimes complements employees by increasing their ability to perform certain tasks, and sometimes substitutes for employees by automating some or all of their tasks. It thus changes job design by refocusing the employee on tasks that are difficult to automate, and eliminating tasks that are easy to automate. Additionally, the effect of new technology can change over time. Initially, it complemented low-skill work. Later, it substituted for it while complementing middle- and high-skill work. Today it complements high-skill work but often substitutes for middle-skill work. It is reasonable to expect that ICT’s effects may change again in the future.
Automating tasks (in machinery or software) has several advantages. It reduces variation since machines tend to perform identically every time. This lowers uncertainty and helps improve the quality of decisions, products, or services. Machines, and particularly computers, often generate large economies of scale. Firms can avoid the complexities of managing employees, including conflict, incentive problems, and absenteeism. Therefore, if the cost of automating a task falls far enough, firms are likely to automate that task.
Which tasks are easiest to automate? Those that are most easily understood, optimized, and codified in advance. Thus, routine, simple tasks have been most susceptible to mechanization and computerization [3]. As noted above, initially, automation was of manual tasks in manufacturing. Experts such as Frederick Taylor devised methods to break production into specific steps, and then optimize each step. Doing so codified the task, which facilitated mechanization. From the 1970s onward, the ICT revolution enabled similar automation of many routine, predictable tasks in clerical and white-collar jobs. Work involving information processing, producing financial forms, making routine calculations, etc., was easily taken over by computers. This “re-engineering” eliminated many middle-skill jobs (e.g. clerical work, data entry, book-keeping), and reduced the number of layers in corporate hierarchies.
Simpler, more stable, and predictable environments favor automation for two reasons: ease of optimization and technological longevity [4]. For tasks to be automated, the firm must invest resources in analyzing and optimizing that part of the process. Perfecting part of a process takes resources (e.g. consultants, total quality management methods). This investment will be more profitable if the optimization problem is easier, as is the case with simpler products and product lines. It will also be more profitable if the new knowledge can be deployed longer in the future, as is the case with stable and predictable environments. For example, UPS (a worldwide package delivery company) famously optimized the job of delivery truck drivers, even to the extent of teaching them how to step into the truck in the quickest possible way. Its business was very simple (deliver a package from one place to another), as well as stable and predictable (methods evolved little over more than 100 years, from bicycles to motorcycles to trucks, then to airplanes for long distances).
Which tasks are more difficult to automate? First, not all manual tasks have proven easy to automate. Physical tasks sometimes involve fine motor coordination and dexterity, which machines have not been able to replicate. They also often involve observing and interpreting the worker’s physical environment, as well as moving within random physical spaces. Computers and machines have historically lacked these capabilities, including vision and image recognition (Figure 1).
Cognitive tasks have also been difficult to automate. They require higher-order thinking skills, while computers have tended to only perform specific, programmed operations. Instead of being automated, jobs involving analysis, decision making, abstract thinking, learning, innovation, and creativity are often complemented by new technology. For example, the job of an aircraft design engineer has changed dramatically. In the past, it involved substantial tedious work, producing complex blueprints by hand calculation and drawing. Now engineers have computers that perform these tasks, freeing them up to focus more on design and complex configuration options [5].
Social tasks have also proven difficult to automate. Computers and robots do not have the ability to empathize with colleagues and customers, inspire employees, use intuition, or listen and communicate with subtlety. Tasks involving social interactions, often in low-skill service jobs and high-skill management jobs, have largely avoided automation. Social skills have become increasingly valuable in the labor market, and employment growth has been largest in jobs that are high in both cognitive and social skill requirements [6]. That is, social and cognitive skills appear to be complementary.
Summing up, a job is a bundle of manual, cognitive, and social tasks. New technology allows firms to automate some tasks, taking them from workers and performing them instead with machines and computers. It also allows firms to provide workers with information, data, analysis, and communication tools that increase their ability to perform other tasks. Thus, the effect of technology on job design rests on a substitute–complement continuum. For some jobs, most or all tasks can be automated. For some jobs, few tasks can be automated, but many can be complemented by technology. Other jobs lie in between, with some tasks automated, some unaffected, and some complemented.
For example, some medical diagnostic tests have been automated, eliminating many medical technician jobs. Some nursing tasks have been replaced by bedside machines that monitor patients and dispense medicine, but the nurse’s interaction with the patient is largely impossible to automate. Finally, virtually all surgeries are still performed by humans, but surgeons have advanced tools that allow them to perform these surgeries more quickly, safely, and effectively.
This process can lead to dramatic differences in employees’ work [7]. For jobs that are mostly automated, managers tend to make most or all decisions and workers simply perform their prescribed tasks. This is because much of the process has already been optimized, so the worker can add little new knowledge to the job, and few decisions or changes need to be made. These jobs usually require few skills, involve only a few repetitive tasks, require little thinking by the worker, and therefore tend to have low intrinsic motivation. By contrast, jobs that are complemented by technology tend to require more skills, including problem-solving and social skills. They tend to make more use of decentralization so that employees learn, and then develop, test, and implement ideas and solutions [4]. As a result, such jobs have high intrinsic motivation [8]. Consistent with these ideas, investment in ICT and research and development are positively associated with more enriched job designs, large-scale organizational change, continuous improvement, and greater competition.
Other effects of technological change
Technology is changing the employer–employee relationship, and even what it means to be an “employee”; however, such effects are only briefly touched upon here as they are not the main scope of this article. It is now easy to collaborate remotely by file and data sharing, email, and videoconference. Except when joint work needs to be synchronous and face-to-face, this can change traditional modes of work, employment, and firm structures. These developments facilitate the globalization of firms, but also enable the outsourcing and offshoring of jobs beyond firm boundaries. They enable new arrangements, with more flexibility in tasks, total hours, timing, and location of work. Recently, this has manifested in the “gig economy,” in which an increasing fraction of the labor force is employed in short-term, part-time arrangements without attachment to a traditional employer. The net effect of these changes on workers and firms are not well understood; society should expect to see further evolution in these areas.
CIT may further change how firms motivate employees. On the one hand, many jobs have become more decentralized. Employees with greater discretion are usually given stronger pay for performance in order to align their goals and decisions with firm objectives. On the other hand, technology leads to greater centralization in some other jobs, and makes it possible to monitor and assess employees in new ways. For example, identification badges can be designed to track an employee’s location, note which colleagues they spend time with, monitor the tone of employee conversations, and note how they stand relative to each other during those conversations. Machine-learning algorithms can then, for instance, analyze such data to evaluate employee “leadership potential.”
Labor market polarization
As new technology substitutes or complements different types of tasks, it changes the relative demand for skills needed to perform those tasks. Skills associated with tasks that machines can now perform tend to see a relative decline in demand, while those associated with tasks that are complemented by new technology see a relative rise in demand. Thus, technological change affects the relative compensation of workers with different skill types. The supply of workers with different skill types will also change. However, labor supply tends to change slowly since it requires changes in education and training. This means that skills and wages tend to be highly correlated. For that reason, labor economics researchers often proxy “skills” by the level of pay.
Automation in modern times has tended to focus on middle-skill jobs. High-skill jobs comprise cognitive tasks, social skills (management and leadership), and creativity. While some low-skill jobs have been automated, those requiring greater dexterity, teamwork, or interactions with customers have not been widely automated. By contrast, middle-skill jobs tend to involve routine information processing, calculation, and decision making. They have therefore been hardest hit by automation with the advent of cheap, powerful computers, and greater access to data.
This pattern is often termed labor market polarization [9]. Polarization has two aspects. First, the relative share of low- and high-skill jobs has increased, with a “hollowing out” of the share of middle-skill jobs. Second, this has increased wage inequality, since middle-skill jobs have fallen in prominence, while complementarity with technology has increased relative compensation for high-skilled workers; Figure 2 illustrates this for OECD countries.
Polarization is a relatively new phenomenon. Until recently, labor markets reflected skill-biased technological change in which technology favored workers with more skill relative to those with less. What is different now is that computers can perform analysis and, to some extent, cognitive tasks; hence, in the last three decades, routine-biased technological change has emerged.
How far and how fast is automation of tasks proceeding?
How technology affects job design has recently changed. Initially, computers had largely automated tasks that could be well-defined and guided by humans, either via traditional computer programs that specify what the computer should do, or expert systems designed to categorize and replicate human decision making. Recently, however, computer scientists have made strides in machine learning, in which computers develop, evaluate, and refine their own algorithms, with little or no human intervention. This presents a new approach: automation of cognitive tasks. Moreover, such algorithms have improved mobility, dexterity, vision, and object recognition in robotics. For example, one influential paper from 2003 describes deciphering the signature on a check and driving a car as tasks that were difficult to automate [3]. However, checks are now routinely processed by computers that decipher handwriting, and driverless cars are being tested in several cities.
The development of computers that can learn is a potentially dramatic change in task automation. How far these developments are likely to proceed, and how quickly, is the subject of great debate. Some argue that the pace of automation has accelerated, including for the first time in high-skilled jobs [10]. One study analyzed the task content of 7,000 jobs and concluded that nearly half, including many high-skilled jobs, are at high risk of automation in the next 10 to 20 years [11]. If that prediction proves true, the implications for labor markets could be dramatic.
However, the study provoked controversy. For example, it analyzed risk of automation at the job level, but jobs comprise a set of tasks, some of which might be automated, while others might not. The illustration on page 1 comes from a work that refined the previous study; the authors concluded that the fraction of jobs at high risk for automation is not 50%, but closer to 5–10% [1]. Jobs at least risk of automation are estimated to involve greater use of deductive reasoning, originality, communication, training, problem solving, and reading and writing. They also have greater requirements for pre-job education or training [1], [12]. However, considerably more work needs to be done before researchers will be confident answering these questions.
Limitations and gaps
A significant limitation of the current debate on technological change is that it is difficult to predict future advancements, effects on job design, and labor market responses. Computer scientists are uncertain of how much progress will be made, and at what pace. Artificial intelligence has proceeded in spurts, with occasional advancements followed by slower periods in which obstacles have proven difficult to overcome. Furthermore, experts may overestimate likely progress in their own field.
Even if one knew the extent of future change, research on potential task automation is speculative and at an early stage. Equally important, but understudied, is the likelihood that ICT and machine learning might further complement tasks rather than automate them. Mechanisms by which technology complements work are not as well understood as those by which it substitutes. More evidence is needed on how machine learning and other technologies are implemented, and on how they substitute or complement different tasks, as well as the ultimate effect on job designs.
The speed at which new technology will be implemented is uncertain. Past experience, including the personal computer revolution of the 1980s, suggests adoption can be slow and difficult. It takes time for organizations to learn practical implementation. Change is slow, complex, and may require high pressure to succeed. Indeed, research indicates that changes in jobs to exploit routine-biased technical change is more significant during recessions. Finally, new technology often faces regulatory hurdles, as well as resistance from political and special interest groups.
The future extent of labor market polarization is likewise unclear. New technology has not always complemented high-skill jobs, and may not in the future. Labor market effects of new technology will depend on complex interactions between skill demand and supply, on how technology is deployed, as well as on trade. Job design and work-focused research and design are endogenous. As middle-skill workers become relatively cheap, firms are motivated to find ways in which technology can complement their work. For example, health care providers are investing in technology that would allow nurses, home health aides, and other middle-skill workers (who are relatively inexpensive compared to doctors) to perform some diagnostics and provide limited patient treatment. Some developments are likely to lead students, employees, and firms to change the type of skills they invest in. International trade allows some types of tasks to be offshored more than others, affecting relative demand for different types of skills [13]. Trade also affects job design, as ICT eliminates geographical barriers to collaboration or offshoring. These interactions are not yet fully understood, and are likely to change in the future.
Summary and policy advice
Some believe the pace of automation, including of high-skill cognitive and social tasks, is accelerating. However, one should not overreact. New technology has always generated dire labor market predictions that have never come to fruition. Technological change has not always complemented high-skill work while replacing low-skill jobs, and may not in the future either. Humans are capable of much that is hard to automate, even with the advent of artificial intelligence. Technology can be used to help people focus on customer service, artisanal and craft work, innovation, education, and more. Furthermore, ICT improves productivity and quality, and generates new products and services. These generate growth, which can improve labor demand [13].
Much of the research on robotics and artificial intelligence is aimed at mimicking humans, which biases toward automation. Policymakers should encourage research into how technology can instead augment human creativity and collaboration, particularly in middle- and low-skill jobs.
What skills are most likely to be valuable with future technological change? First, abstract thinking, analytical, and problem solving skills. For this reason, mathematics, statistics, science, engineering, and economics have risen in prominence. Second, creativity, and social and communication skills. Educational institutions need to teach this combination of skills.
Many laws and regulations were established when unions and long careers in a single large corporation were the norm. These often impose detailed, inflexible arrangements that may no longer fit the current and future labor market. Policies that loosen the formal definition of an “employee” would enable people and firms to more flexibly and creatively realize ICT’s potential to enrich careers and improve work–life balance. They would increase productivity and should encourage innovation.
Acknowledgments
The author thanks two anonymous referees and the IZA World of Labor editors for many helpful suggestions on earlier drafts. He also thanks Alec Levenson and Cindy Zoghi for discussions on this topic during their collaboration, Geoffrey Gibbs and Kathryn Ierulli for valuable comments, and Hieu Nguyen for research assistance. Financial support from the University of Chicago Booth School of Business is gratefully acknowledged.
Competing interests
The IZA World of Labor project is committed to the IZA Guiding Principles of Research Integrity. The author declares to have observed these principles.
© Michael Gibbs