Elevator pitch
Policy evaluation aims at assessing the causal effect of an intervention (for example job-seeker counseling) on a specific outcome (for example employment). Frequently, the causal channels through which an effect materializes can be important when forming policy advice. For instance, it is essential to know whether counseling affects employment through training programs, sanctions, job search assistance, or other dimensions, in order to design an optimal counseling process. So-called “mediation analysis” is concerned with disentangling causal effects into various causal channels to assess their respective importance.
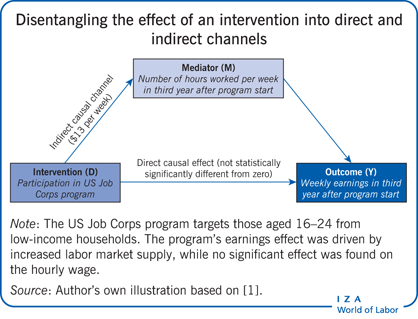
Key findings
Pros
Mediation analysis of a particular policy effect gives a better understanding of why specific policy interventions are effective or ineffective.
Mediation analysis directed at a policy effect’s causal channels is likely to result in better policy advice, particularly with respect to the optimal design of the various components of a policy intervention.
Analyzing causal channels helps to understand the aspects of an intervention whose effectiveness appears particularly interesting (i.e. more relevant than that of other aspects).
Cons
Analyzing causal channels requires stronger behavioral (or identifying) assumptions than evaluating the “conventional” (total) causal effect of a policy intervention.
Sufficiently rich data, which plausibly justify key behavioral assumptions, are needed to analyze causal channels; panel data are typically required in these cases, but are not always available.
Author's main message
Policy evaluations have widely neglected the potential merits of analyzing causal channels to deliver more accurate policy advice. Mediation analysis appears increasingly attractive in a world with growing availability of rich data, even though it relies on non-trivial behavioral assumptions and comparably strong data requirements. The assessment of causal channels by mediation analysis should thus be considered for future policy evaluations. For instance, when investigating the extent to which a labor policy’s effect on earnings comes from increased search effort, increased human capital, or other mediators that are themselves affected by the policy.
Motivation
Policy evaluation typically aims at assessing the causal effect of a policy intervention, often referred to as a “treatment” (e.g. an active labor market policy), on an economic or social outcome of interest (e.g. employment or income). Most evaluations focus on the “total” causal effect of the treatment, rather than the underlying causal channels that drive this effect. That is, these evaluations do not typically investigate the possibility that the total effect may be rooted in distinct causal channels related to intermediate variables that affect the final outcome. These intermediate variables are often referred to as “mediators,” and the investigation of their role is known as “mediation analysis.” If such mediators exist, the total effect can, under particular assumptions, be decomposed into several channels.
As the Illustration shows, the various channels are a direct effect of the treatment on the outcome, and one (or multiple) indirect effect(s) which “run(s)” through the mediator(s). Such decomposition frequently offers a more comprehensive picture about social and economic implications than the total effect alone, and may be important for deriving meaningful policy conclusions [2].
Discussion of pros and cons
Illustrative examples
Three examples are used to emphasize the potential merits of mediation analysis. First, consider the employment effect of job-seeker training followed by additional (human capital increasing) programs that also affect employment. Disentangling the direct and indirect effects (via the additional programs) shows whether the initial training is effective per se, or only together with the later programs. This can be useful for the optimal design of (sequences of) programs for job-seekers [2].
Second, consider disentangling the employment effect of the entire job counseling process provided by employment offices. It is interesting to determine whether the treatment “job counseling” affects employment through placement into training programs, job search assistance, sanctioning (or threat of sanctioning) in the case of noncompliance, personal communication or counseling style of the caseworker, or other dimensions [3]. Knowledge about these channels may help develop guidelines for a more efficient counseling process.
Early childhood interventions represent the third example. These may, for instance, provide access to (high-quality) childcare or increase the teacher-to-children ratio in kindergartens for children from families with a disadvantaged social background. Here, an important issue is whether such interventions affect outcomes later in life (e.g. income, health, and life satisfaction) exclusively through educational decisions (e.g. graduating from high school or college), which are themselves affected by early childhood conditions, or also through other channels, like personality traits. This may provide insights as to whether interventions at a later point in life (e.g. waiving tuition fees for college attendance) can be as effective as early childhood programs in terms of producing socially desirable impacts.
The evaluation of direct and indirect effects is now widespread in social sciences thanks to a key piece of seminal work [4]. However, a significant portion of the earlier literature on causal channels relies on rather rigid behavioral models. One problematic restriction is that the effects of (i) the treatment on the outcome; (ii) the treatment on the mediator; and (iii) the mediator on the outcome are commonly characterized by (linear) models which assume the respective effects to be the same for everyone in the population of interest (irrespective of differences in individual characteristics). Even though this makes mediation analysis very convenient, it imposes severe rigidities on the nature of human behavior. A second unrealistic restriction, which is often imposed implicitly, is the quasi-randomness of the mediator with respect to the outcome. In other words, it is assumed that, apart from the treatment itself, there are no further characteristics that jointly influence the mediator and the outcome. Mediation analysis is not straightforward, even when the treatment is randomized, for instance in an experiment. The randomness of the treatment does not imply randomness of the mediator, because the mediator is itself a post-treatment variable (and can, thus, be interpreted as an intermediate outcome) [5].
An intuitive example that demonstrates how the careless handling of a mediator likely leads to flawed results can be found when assessing the effect of a mother’s smoking during pregnancy on post-natal infant mortality. In general, the empirical literature finds a positive relationship between smoking and infant mortality. However, several studies point out that, among those children with the lowest birth weight (i.e. conditional on the mediator “low birth weight”), smoking appears to decrease mortality. This paradox is most likely a result of the researchers having failed to consider (important) characteristics related to both birth weight and mortality [6]. Consider, infants who have a low birth weight because of their mothers’ smoking have a lower mortality rate than other infants with a low birth weight whose mothers did not smoke; this may be true if the low weight of the latter group is due to characteristics that entail a higher mortality rate than is associated with smoking (such as birth defects).
Strategies to assess direct and indirect effects: Selection on observables
To prevent these kinds of issues, two types of strategies or sets of statistical restrictions have primarily been used to plausibly assess direct and indirect effects. The first relies on the assumption that researchers observe all characteristics that jointly affect the treatment and the outcome, the treatment and the mediator, or mediator and the outcome, which is known as the “selection on observables” assumption. This implies that one can measure the direct and indirect effects on the outcome by comparing groups in different treatments and mediator states that are comparable in terms of such (observable) characteristics. Figure 1 illustrates such a set-up, in which the observed characteristics (or covariates) are denoted by X, and may have an impact on the treatment D, the mediator M, and the outcome Y [2]. As an illustration, consider the first example presented above and let D represent a training program, M a further program later in time, and Y employment. X reflects factors affecting all three variables, for instance, education, work experience, age, profession, and other factors that likely affect placement into training (D), further programs (M), and employment (Y).
As in the Illustration, each arrow in Figure 1 represents the causal effect of one variable on another. This selection on observables assumption is stronger than that invoked in the “conventional” analysis of a (total) treatment effect. The latter concept merely requires that one must observe all characteristics that jointly affect the treatment and the outcome, but does not consider their impacts on the mediator.
Application opportunities for mediation analysis
Several studies discuss more or less flexible estimation approaches for the causal framework shown in Figure 1, and predominantly consider applications for this framework in biometrics, epidemiology, and political science. Similarly, contributions to mediation analysis are on the rise in labor economics and policy evaluation. For instance, one study decomposes the effects of the Perry Preschool program (an experimental intervention targeting disadvantaged African American children in the US) on later life outcomes into causal channels related to cognitive skills and personality traits [8]. An investigation into the direct impact of the Job Corps program for disadvantaged youth in the US on earnings considers “work experience” as a mediator to account for potentially reduced job search effort during program participation, so called “locking-in effects” [9]. The direct health effects from Job Corps are assessed in another study, as well as the indirect effects via employment [7]. A different study applies the mediation framework to the (widespread) decomposition of wage gaps (e.g. between males and females or natives and migrants) into an explained component (due to differences in mediators such as education, work experience, profession) and an unexplained component (possibly due to discrimination and other unobserved factors) [10].
These examples show that causal mechanisms play a role for a range of questions that are interesting to policymakers, even though this is not typically recognized in most modern policy evaluations. It is also worth mentioning that user-friendly software packages have been developed, making the methods more and more accessible to a broader audience [11]. This might pave the way to increased use of mediation analysis in the context of policy evaluation with potential gains in the quality and comprehensiveness of the derived results, which would lead to more effective policy advice.
It is noteworthy that in Figure 1 the same set of covariates (observable characteristics) is assumed to affect the treatment and the mediator (and the outcome). However, the complexity of assessing causal channels increases if some covariates that affect the mediator and the outcome are themselves influenced by the treatment; this is known as “dynamic confounding.” In this case, the selection on observables assumption needs to be augmented by further assumptions with respect to the treatment–mediator, the covariates–mediator, or the mediator–outcome association. Thus, a trade-off exists between the flexibility needed to handle confounding issues and the flexibility allowed in the model specification. In other words, dynamic confounding issues require a more rigidly designed evaluation model, which might limit the potential general application of the model.
To date, mediation analysis with dynamic confounding has rarely been considered in real-world applications, even though it represents an empirically relevant case. As an illustration, consider the third example presented above and let D represent childcare quality, M college graduation, and Y earnings later in life. Childcare quality (D) may affect personality traits like self-confidence or motivation, which may affect both the decision to go to college (M) and earnings (Y) later in life (through work-related behavior).
Alternative strategies: Instrumental variable approach
In many empirical problems, the selection on observables assumption (i.e. the assumption that all factors that jointly affect the treatment, the mediator, and the outcome are observed) might not be plausible. This is the case if for some characteristics that arguably influence the treatment decision, the mediator, and/or the outcome, there are no or no appropriate measures available in the data. For instance, if motivation, ambition, or innate ability drives participation in job-seeker training (treatment) and further programs (mediator) as well as earnings (outcome), but cannot be (adequately) measured, the selection on observables assumption fails. An alternative strategy consists of using “instrumental variables” (IV), which must affect the treatment and/or the mediator, but at the same time must not directly influence the outcome (other than through the potentially altered treatment and/or mediator).
Relatively few papers consider the evaluation of causal channels based on IV. One such study assumes a randomly assigned treatment and a “perfect” instrument for the mediator, which forces the latter to take a particular (and desired) value [5]. Such perfect instruments are, however, hard to find in real-world applications. Considering, for instance, the third example from above with college graduation as the mediator, the random assignment of waivers for college tuition fees could serve as a plausible instrument: waivers likely influence the decision to go to college while not having a direct effect on outcomes later in life, such as earnings. However, waivers most likely do not represent a perfect instrument, as they probably do not affect the college decision of all individuals. That is, the mediator of some individuals may not react to its prescribed instrument.
There is some discussion in the literature about the assessment of causal channels in experiments based on “imperfect” instruments for the mediator (i.e. allowing for the “non-reaction” of some individuals), while the treatment is assumed to be random [12]. Under a particular experimental design, the causal channels can be measured for those individuals whose mediator reacts to its instrument. This implies that direct and indirect effects can be evaluated with double randomization of the treatment (e.g. random assignment of high-quality childcare places) and an instrument for the mediator (e.g. tuition waivers for school or college). This appears to be a promising strategy for the future design of randomized trials aiming at evaluating policy interventions.
Further research considers two distinct instruments for both the treatment and the mediator for various models and assumptions on the behavior of the mediator and its instrument [1]. One interesting contribution is the evaluation of causal mechanisms in cases where the instrument for the mediator may take many different values. In practice, this may be implemented by randomizing a financial incentive (for instance, for the mediator, “college attendance”) whose amount (randomly) varies among study participants. An illustration of the framework is provided in Figure 2, where double-edged arrows imply that causality may run in either direction. The first instrument Z₁ affects D, but not directly Y, i.e. it may only impact Y through its effect on D. The second instrument Z₁ affects M, but similarly, not directly Y. In such a setup, direct and indirect effects may be measured despite the presence of the presumably unobserved characteristics denoted by U, V, and W, which affect each other and D, M, and Y [2]. As an example, assume that Z₁ represents the random assignment to a childcare facility and D the actual use of the childcare facility, which may differ from the assignment if some families do not comply with the assignment. M is college attendance while Z₁ is a tuition waiver, whose amount may randomly vary such that the tuition fees of some individuals are fully covered, while those of others are only partly or not at all compensated. Y is income while U, V, and W are unobserved characteristics like family background, ability, motivation, etc. In this framework, the unobserved variables are likely associated with placement into early childcare facilities; for instance, more highly versus less educated parents may systematically differ with respect to such decisions. Family background (W) is also expected to affect the motivation to go to college (V) and the motivation at work (U), the latter being a determinant of income (Y). The causal mechanisms can nevertheless be evaluated if childcare slots (Z₁) are randomly assigned and actually affect the decision to use childcare, at least among a subset of families. Similarly, the tuition waivers (Z₂) must induce some individuals to go to college when being offered a waiver. Neither of the randomized variables Z₁ or Z₂ may, however, have a direct effect on income later in life. That is, they may affect Y only through the treatment and the mediator, respectively.
Note that the causal framework depicted in Figure 2 could be augmented by observed covariates that affect the instruments, the treatment, the mediator, the unobservables, and the outcome, but this has been neglected here for the sake of simplicity. As an example, assume that family wealth is observed in the data and affects education decisions and later life earnings, as well as the chances to be assigned a childcare slot or a tuition waiver for college. Therefore, childcare slots and waivers are randomly assigned among families with the same wealth, but not across different wealth levels (e.g. families with lower wealth might have higher chances to obtain a slot or waiver than those with a higher wealth). Similarly to the selection on observables framework, the IV assumptions necessary to measure causal channels are generally stronger than those required for the IV-based assessment of (total) treatment effects.
Data requirements and availability
The appropriate strategy, considering either the IV or selection on observables approaches (or neither), can vary from one empirical problem to another, and must be assessed individually, considering the available data. First, the statistical approaches generally only appear credible in the presence of panel data, which permit measurement of the treatment, mediator, and outcome variables at different points in time in order to match the causal framework considered in the Illustration.
Second, the assumptions may only be plausible when a large number of variables are observed, such that unobserved factors that jointly affect the treatment, the mediator, and the outcome (in the case of selection on observables) or the instruments and the outcome (in the case of IV) can be ruled out. For instance, if parental income affects both the probability to be assigned to high-quality childcare or to obtain a college tuition waiver and outcomes later in life (such as earnings), then parental income needs to be observed by the researcher to avoid blurring the effects of childcare or college attendance with the impact of parental income. This requires collecting rather comprehensive data in terms of information on study participants.
Concerning the IV approach, a further caveat is that credible instruments (that do not directly affect the outcome) may be hard to find in empirical data.
An alternative to the approaches outlined so far is the definition of a strict, so-called “structural dynamic model,” which is explicit about any possible choices and channels that are formally permitted within the assumed causal framework. An example is found in a study that estimates schooling, work, and occupational choice decisions based on a structural model [13]. Bluntly speaking, economic theory replaces the need for selection on observables or IV assumptions. While this approach allows for explicit focus on the causal channels of interest, its usefulness crucially depends on the appropriateness of the narrowly specified theoretical model, which may not hold up in reality.
Limitations and gaps
A survey of previous literature shows that the analysis of causal channels generally requires stronger behavioral (or identifying) assumptions than merely evaluating the (total) causal effect of a policy intervention. Partly related to that, data requirements are also higher when evaluating causal channels. First, a comparably rich set of characteristics needs to be observed, to the extent that selection on observables and IV assumptions appears credible in the context of mediation analysis. However, whether a data set is “rich enough” is ultimately not directly testable by means of statistical methods, but needs to be judged on a case-by-case basis, in light of the theoretical considerations and empirical evidence. Second, a panel data set is typically required to measure the various factors at play at different times for the causal framework under consideration (such framework consisting of an initial treatment, an intermediate mediator, a final outcome, and possibly observed covariates that need to be controlled for).
Furthermore, not all methods that are commonly applied in policy evaluation have yet been transferred to the context of mediation analysis. For instance, the feasibility and merits of approaches used in the context of “natural experiments” (interventions that are not experimentally assigned by the researcher but nevertheless contain a quasi-random element) have not yet been considered for analyzing causal channels. This represents a substantial area for the development of further evaluation methods to address and analyze causal channels in future research.
Summary and policy advice
Mediation analysis comes with the caveat that it generally implies higher behavioral restrictions and data requirements than analysis of the (total) causal effect of some policy intervention. However, if these concerns can be satisfactorily addressed, then mediation analysis can allow for a better understanding of particular causal effects through the evaluation of specific causal channels. This may result in more accurate policy advice, particularly when it comes to the optimal design of (the various components of) a policy intervention. For instance, mediation analysis permits investigating specific aspects of an intervention that are of particularly high interest, such as how important placement into training programs is as part of a counseling process aimed at the reemployment of job-seekers.
The feasibility and attractiveness of mediation analysis in policy evaluation will likely increase in a world with growing availability of rich data and appropriate software packages. It is therefore recommended to take the assessment of causal channels into consideration in (the design of) future policy evaluations. This can be most credibly achieved by designing policy experiments with double (or two-step) randomization of both the policy intervention (e.g. job-seeker counseling) and the mediating factors (e.g. training participation). This will imply that several of the behavioral assumptions discussed above are valid according to the chosen experimental design. Policymakers may therefore want to engage or collaborate with researchers to run such experiments in order to improve on “classical” policy evaluations of the total causal effect of an intervention.
Acknowledgments
The author thanks two anonymous referees and the IZA World of Labor editors for many helpful suggestions on earlier drafts. Previous work of the author (together with Markus Frölich) contains a larger number of background references for the material presented here and has been used intensively in all major parts of this article [1].
Competing interests
The IZA World of Labor project is committed to the IZA Guiding Principles of Research Integrity. The author declares to have observed these principles.
© Martin Huber