Elevator pitch
Both the availability and sheer volume of data sets containing individual molecular genetic information are growing at a rapid pace. Many argue that these data can facilitate the identification of genes underlying important socio-economic outcomes, such as educational attainment and fertility. Opponents often counter that the benefits are as yet unclear, and that the threat to individual privacy is a serious one. The initial exploration presented herein suggests that significant benefits to the understanding of socio-economic outcomes and the design of both social and education policy may be gained by effectively and safely utilizing genetic data.
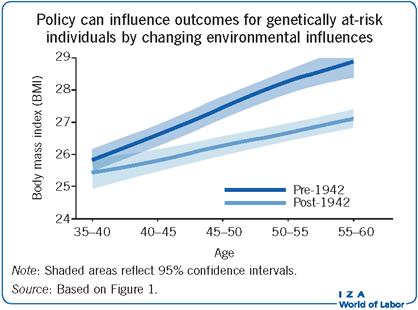
Key findings
Pros
Genetic data provide a useful way to understand individual differences in socio-economic outcomes.
By understanding the genetic basis of specific outcomes, policies and treatments could be more efficiently targeted.
Recent research indicates that genetic associations with outcomes such as obesity may vary across birth cohorts due to different prevailing environmental contexts.
Differences in genetic inheritance between full biological siblings offer a new source of information to estimate causal effects.
Cons
The majority of evidence so far reflects only simple associations between individual genetic factors and socio-economic outcomes, in contrast to causal relationships.
The effect sizes for most genetic factors are very small in magnitude.
Use of genetic data causes concerns for infringement of individual privacy and human rights.
The availability of genetic data may influence decision making and potentially lead to discrimination based on one’s genotype.
Author's main message
Molecular genetic data offer the potential to design new, effective approaches to improving societal outcomes. For example, existing research has significantly advanced knowledge of how mental health influences education and labor market outcomes. Yet there remain concerns surrounding the potential for data on inherited predispositions to be abused in either the workplace or in insurance coverage. Given the complexities of the new data, it is critical that researchers educate the public on the true promise it offers, so that society can become comfortable with genetics playing a role in policy design.
Motivation
In 2001, scientists working collaboratively from around the world completed the sequencing of the human genome. Since that feat, medical research has increasingly focused on disease mechanisms at the cell and molecular levels, helping to generate significant interest in the development of “personalized medicine.” Research has even begun to shed light on how molecular genetics influences many commonly studied individual socio-economic outcomes, such as educational attainment and fertility. However, societies now face some critical questions as gene-based research continues to progress: Should molecular genetic information be considered in the design of social and economic policies? Should genes come to play a central role in society’s thinking about socio-economic issues?
For many decades economists have generally ignored the role of genetic factors. For example, many researchers investigated the intergenerational transmission of traits and socio-economic outcomes such as inequality, but were hesitant to identify the source of intergenerational correlations. Those that attempted to ascertain the role of genetic factors used samples of biological siblings in a bid to understand heritability in various traits and outcomes, ranging from educational attainment to smoking to risk attitudes. Heritability is generally defined as the proportion of variation in a population that is accounted for by genetic factors. Many social scientists may have refrained from undertaking these exercises, in part due to concerns that the results could be distorted to suggest they are promoting social eugenics.
With molecular genetic data, researchers can examine if differences in the genetic code at specific locations of the DNA chromosome pair are linked with these specific differences in socio-economic outcomes. Variation among individuals’ DNA sequences may directly or indirectly (through environmental channels) influence many socio-economic outcomes. While early studies by economists typically explored variation in a specific genetic marker, called a candidate gene, recent work considers either a larger number of genetic factors simultaneously or a summary measure of individual genetic variation.
Perhaps it is not surprising that both social scientists and policymakers have serious concerns about the idea of using molecular genetic data. The major reason social scientists resist examining how genetic factors relate to individual behaviors is possibly that genes are purely predetermined. Hence, any impact genes have on socio-economic outcomes would be innate, which leaves no role for policy interventions. This simplistic reasoning is incorrect [1], an issue that will be elaborated on below.
Discussion of pros and cons
Individual specific, but no longer unobserved, heterogeneity
In countless studies labor economists attempt to isolate the influence of a single explanatory variable (e.g. education) on an outcome of interest (e.g. earnings). To convince readers of their principal finding, researchers often assess whether their estimates are sensitive to the inclusion of additional observable characteristics, such as experience, age, height, and health. Until recently, genetic data at the molecular level were not available. This may represent a serious limitation to prior research, since studies on heredity suggest that genetic factors could explain up to 65–80% of the variation in height and 20–40% of the variation in educational attainment.
Without data on genetic characteristics, researchers could only use longitudinal data to create a proxy to capture all unobserved (time-invariant) individual specific heterogeneity. This approach, generally referred to as a fixed-effects analysis, would allow to control for both unmeasured individual specific factors, such as one’s genetic code, and unmeasured skills, such as innate ability, perseverance, industriousness, or motivation. This approach assumes that the unobserved factors have a constant effect for individuals as they age, which is a strong (and not always realistic) assumption. As shown in the Illustration, a variant of the well-studied FTO gene may have different impacts on the body mass index (BMI) as individuals age, implying that the effect actually changes over time.
Genetic data may be what is truly meant by unobserved individual specific heterogeneity. Genetic markers and the structure of one’s DNA are fixed at conception and do not change over the lifecycle, unlike one’s skills, which an increasing body of evidence shows can be developed via education and training investments. In other words, how genes are expressed may change, but the structure of DNA itself cannot.
Data on genetic markers may permit researchers to enter the black box of individual specific unobserved heterogeneity. If genetic markers are linked to traits that are both accounted for and explained by regression models, then failing to control for genetic differences may generate biased estimates and distort research findings. Yet one of the challenges is how to incorporate genetic diversity across individuals in an efficient and meaningful way. After all, there are millions of locations in one’s DNA where genetic differences emerge. An early literature explored links between economic outcomes and specific locations on the chromosome pair (referred to as candidate genes). However, since the findings on the strength of these associations were not robust across samples and may have been due to data snooping, the research community has proposed investigating links between outcomes and a sufficient statistic, called a polygenic risk score, which is often calculated as the cumulative weighted sum of the variation in multiple genetic locations.
However, to determine these weights and the genetic locations to be included in the calculation of a polygenic risk score, evidence from a genome-wide association study (GWAS) is needed. A GWAS can be thought of as a hypothesis-free scan for associations between a specific outcome and subsets of the millions of genetic variants. Looking at the predictive powers of polygenic scores derived from different GWASs over the last five years, a recent study summarizes how the score’s ability to explain variations in educational attainment has increased from 3% to 7% (in this study, educational attainment is taken as years of completed schooling) [2]. This research points out that very large data sets are needed to undertake GWASs since most of the statistically significant effects are very small. Further, larger sample sizes also permit the possibility of identifying rare genetic effects that may not be measured by the underlying genotyping arrays currently used in GWASs.
In sum, while the amount of variation explained by these genes is significantly smaller than estimates from heritability studies that exploited variation in families, recent research shows that the significant markers identified are also associated with neurocognitive disorders and brain function [3]. Hence, this type of research not only identifies the individual genes, but also suggests the biological pathways by pointing out the commonalities in correlations of specific genes with different outcomes (i.e. brain function and educational attainment).
Genetic factors can identify new challenges and diagnoses
When economists use genetic data in the empirical microeconomics literature, they must often overcome empirical challenges, for example when estimating the effects of poor health on outcomes such as years of schooling or wages. The challenge arises since poor health is often measured with error, while also being systematically related to unobserved determinants of the outcome variable. In these situations, instrumental variables regression can be used. Here an additional variable (an “instrument”) is used to replace the problematic independent variable with a proxy that is unrelated to both the unobserved factors that affect the outcome and the measurement error. A study from 2009 provides the first application of genetic markers in an instrumental variables application to identify causal estimates of how physical and mental health conditions in adolescence affect academic performance [4]. Thereby, the health variables are isolated from other explanatory factors such as nurture inputs, which in this case might include the neighborhood in which the families reside and the peers with which the adolescents associate. In using this approach, the researchers assume that the genetic instruments are not only correlated with the poor health measures, but that they only influence academic outcomes through these health variables. The main findings from this study are that depression and obesity both lead to an approximate one standard deviation reduction in academic performance. But this deterioration is shown to differ by student gender: young women are found to be more adversely affected by negative physical and mental health conditions. Lastly, the separate impacts of inattention and hyperactivity on academic performance differ sharply in magnitude and sign.
While this investigation and subsequent work have led to significant debate about which genetic markers are valid instruments, such work has also highlighted that measuring an individual’s health status is challenging due to comorbid health conditions, that is, situations where two or more disorders or illnesses occur in the same person (whether simultaneously or sequentially). In its empirical application, the 2009 study shows that omitting comorbid diagnoses would result in biased estimates of the causal effect of specific health diagnoses on socio-economic outcomes [4].
Many current definitions of health are based strictly on symptoms. Multiple disorders can share symptoms, but confusion in reaching a diagnosis can occasionally arise from how doctors decide to classify disorders. For example, beginning in 1994 all forms of attention-deficit disorder would be called “attention-deficit/hyperactivity disorder,” even if the person was not hyperactive. With molecular genetic data, it is likely possible to define the genetic etiologies of specific disorders. That is, conditions that are observationally difficult to distinguish may be composed of a small number of distinct conditions that differ based on their genetic makeup, thereby permitting new classifications of disorders [5]. Doing so and subsequently using genetic data would lead not just to a more accurate diagnosis, but also to benefits from more effective treatments [6]. Further, as knowledge about one’s genetic makeup increases, associated risk scores will provide individuals with an opportunity to respond to a condition more rapidly, and at lower costs than having an expert reach a diagnosis, before any symptoms manifest. In summary, the use of genetic markers has clarified the challenges researchers face in precisely measuring health in any empirical study, and may assist medical professionals in both diagnosing and treating health conditions.
Genetic lotteries and research design
Genetic markers are inherited from one’s biological parents, whose own markers are, in turn, inherited from their own biological parents. Dynastic effects relating to the line of heredity present obvious challenges in the use of genetic information as either control or instrumental variables, since, where unobserved, such effects may confound the estimates. Consider estimating the link between one’s genes and educational attainment. If there is a history of positive assortative mating in the family on the relevant genetic loci that affect educational outcomes—which would mean that these genes are positively correlated with educational achievement across generations—researchers may be led to overstate the relationship between an individual’s attainment and their own genes. While control variables could be added to capture parents’ or grandparents’ education, there remains a possibility of bias from omitting prior generations in the dynasty.
To overcome this challenge and obtain causal estimates, two researchers have introduced a new empirical strategy—coined the genetic lottery—which exploits genetic inheritance within full biological siblings in a bid to remove dynastic effects [7], [8]. While economists are familiar with natural experiments, the authors point out that within families, differences in the inheritance of specific genetic markers present the opportunity for additional experiments in “nature” [8]. The academically important contribution of the two studies is that the genetic lottery approach can be used to test whether family fixed-effect estimators on their own solve endogeneity problems.
The main finding in the first of the above studies is that inattentive symptoms in early childhood, assessed via a screening questionnaire, have large and lasting effects in reducing completed schooling [7]. The study also finds little consistent evidence that the overweight status of adolescents influences years of completed schooling. The authors argue that there are policy implications for the timing of interventions since health and productivity are often speculated to have a complex interdependence in the workplace. That is, health measures targeted at youth may affect future education and career choices, so there may be large benefits to individuals’ adult labor market outcomes from school-based programs that target childhood and adolescent health measures.
The availability of genetic data thus provides a way to test key identifying assumptions in this common research design, which has been applied in almost every branch of empirical economics as well as behavioral genetics. The genetic lottery provides a new research design for social scientists, and in many GWASs researchers now examine if the results are robust to controlling for family fixed effects.
The importance of genetic factors in social inequalities
Studies that use genetic markers as an instrumental variable are not suggesting that heredity is destiny. To illustrate this point, consider the rapid spread of obesity around the globe in the last 50 years. Researchers in the biological sciences have conducted searches for genetic variants that play a role in obesity, and have developed a rich evidence base for genetic and epigenetic mechanisms involved in the susceptibility and development of obesity. However, genetic changes remain largely stable across many generations of a population, so this is unlikely to explain the drastic rise in obesity rates over the last 50 years.
Many economists and social scientists have hence conducted a parallel program that explores whether changes in the environment that make it easier for people to overeat and harder for people to get enough physical activity can explain the rise in obesity. This research has influenced policy discussions related to taxes on sugar sweetened beverages, labeling of fast food in terms of calories, and the presence of soda machines in schools, among other proposals. Evidence underlying these policy debates often comes from studies that ignore genetic marker confounders, which may be an important consideration, particularly if identical policies or treatments have heterogeneous effects across individuals with different genetic makeups. These differential responses could be significant in cost–benefit exercises if a given policy is discovered to influence one group (defined here on genetic characteristics) substantially more than others. After all, just because genetic characteristics have historically been unobserved, this is not an excuse to engage in potentially discriminatory treatment now that genetic information is becoming available.
Obesity is a complex disorder with both genetic and environmental causes, and this requires researchers to use data and estimate models that allow environmental and genetic effects to interact in complicated ways. Further, to develop credible evidence on how genetic and environmental factors contribute to obesity requires exogenous variation in environmental conditions. A study from 2015 considers an alternative strategy which uses data from different birth cohorts in the offspring sample of the Framingham Heart Study (a US project of the National Heart, Lung, and Blood Institute and Boston University), and econometric models that can identify unknown breakpoints in relationships between a specific genetic factor and obesity (as measured by BMI) [9]. This genetic variant is of one well-studied obesity-promoting gene, commonly referred to as the FTO gene. The study tries to sort between differences in BMI within individuals over time (age effect), population-wide differences in BMI over time (period effect), and differences in the experienced period effect across individuals of varying ages (cohort effect). A robust relationship is found between birth cohort and the FTO risk allele with BMI, with an observed inflection point for those born after 1942 [9]. Figure 1 presents smoothed means of BMI for individuals sampled in the Framingham Heart Study with three unique genotypes and separated by birth cohort (before and during/after 1942). Individuals with two of the genotypes (AA and AT) in the later birth cohort have consistently higher BMIs over time, while those with the more common TT genotype do not. The results show that the environment one grows up in plays a large role in BMI, and that BMI also increases on average as one ages.
The above study also has important implications for the scientific literature focused on gene discovery since it raises the possibility that genetic associations may differ across birth cohorts due to variation in prevailing environmental contexts [9]. Many GWASs pool different data sources in order to increase the sample size to help identify small effects and increase their statistical power. However, doing so may introduce bias if information on differences across subjects’ birthdates and when and where the data were collected are not accounted for. These lessons hold for any study using genetic data to explain complex outcomes that result in social inequality and demonstrate the need for careful (econometric) modeling.
Genetics and public policy
Heritability plays a role in nearly every socio-economic and health outcome. This feature has long been ignored by social scientists and policymakers. However, heredity is not destiny and much work is needed to translate the revolutionary advances in genetics and genomics to reach both policy audiences and the broader academic community. The idea that a benevolent social planner can induce greater success by personalizing policy to individuals based on their genetic code has clear appeal to many, as does the concept of personalized medicine. Yet, as discussed earlier, issues related to privacy and discriminatory treatment based on genetic characteristics open a new set of challenges to designing effective policy.
The speed at which molecular genetic data can be effectively integrated within policy design is directly tied to improvements in understanding how genetic markers operate. For example, huge advantages may be gained if genetic screening can reliably predict complex learning disorders. That is, even if a disorder is a function of many genes, each with very small effects, researchers can calculate a single summary score from an ensemble of markers that have well-validated significant effects. The summary score provides a measure of an individual’s risk for a specific disorder or trait, which, in many situations, may take psychologists years to diagnose. Armed with knowledge of whether their child or employee is at an elevated risk for a given poor outcome, parents and employers will be able to make different investments, years prior to receiving a formal diagnosis. These investments may affect how the underlying genes are expressed and therefore reduce the risk for future poor outcomes. Moreover, as knowledge advances the predictive accuracy of these summary scores will increase. This could improve policy evaluations, since researchers could net out the contribution of genetic factors on the individual outcomes to provide cleaner evidence on the effectiveness of environmental inputs.
An important insight worth stressing is that even when a problem has its root in genetic factors, there is still a role for public policy [1]. Take the example of poor eyesight. Even if poor eyesight was strictly a result of genetic inheritance, policymakers could provide glasses to those afflicted. In other words, when discussing genetics and public policy, attention should not be focused upon the question of whether a specific outcome or trait is primarily a function of genes. Instead, policymakers must ask whether the available evidence suggests that a policy would pass a cost–benefit test. Any cost–benefit test could further consider the consequences of issuing a broad mandate versus targeting policy to those with specific characteristics. In summary, the success of integrating molecular genetics within a policy making environment does not require a wholesale transformation of how policies are developed.
Caution and care are needed when using and interpreting genetic data
Evidence from GWASs has been very influential and garnered significant press coverage in recent years. A strength of GWASs is that they investigate the full genetic code, in contrast to methods that specifically test a small number of prespecified genetic regions. Hence, GWASs represent a non-candidate-driven approach. Often, the popular press reports the results from GWASs as being causal, but they are only simple links between genetic variants at a specific location; the outcome under investigation and the mechanism by which a given genetic variant impacts a given outcome generally remains unknown. The effect size of any significant location is typically quite low, and it is rare to find a genetic variant that can account for more than 0.001% of the variation in traits of interest to labor economists. For example, evidence from a recent GWAS concludes that the effects of any single difference in genetic markers on self-employment are likely to be a very small proportion (<0.002%) of the variance in self-employment [10].
Most complex disorders are the result of many genetic variants, each with small effects. However, caution is required if one decides to change their behavior in response to this new information. In April 2017 the US Food and Drug Administration allowed the genetic testing firm 23andMe to sell reports with qualifiers showing customers whether they have an increased genetic risk of developing certain diseases and conditions. The number of conditions are limited and this reversed a decision in 2013 that forced 23andMe to stop the delivery of results on health-related traits.
An example where care is taken with reporting genetic information is the Stanford Cancer Institute tool for individuals with known mutations in the BRCA1 or BRCA2 genes, which put women at higher risk for cancer (available at http://brcatool.stanford.edu/). This calculator provides information on how the chances of survival change in response to different preventive measures at different ages. Yet this type of calculator is available only for a handful of genetic variants, suggesting that there may be unintended consequences from responding (especially over-responding) to a predisposition (that is correlational), as well as pointing to the possibility of patient-demanded medical care that may prove to be ineffective. Given the limited understanding of how genetic markers operate, the provision of this information without appropriate context and qualifiers could worsen outcomes.
The moral and ethical questions of how molecular genetic data should be utilized are heavily debated. These data may characterize an invasion of privacy and violate human rights. Concerns are emerging in multiple venues that the presence of molecular genetic data may require careful regulation on the part of governments. The principal concern is with regard to the potential for genetic data on inherited predispositions to influence decisions in either the workplace or on insurance coverage. For example, over 60 countries presently have a DNA database of convicted criminals, and there is debate about whether the benefits to society trump an individual’s right to privacy. Further, since these data can be linked to arrest records, they can also potentially be used by employers when deciding to hire, promote, or terminate workers. Similarly, these data may lead to disparate treatment or genetic discrimination by health insurers, who might refuse to give coverage to an individual who has a genetic difference that raises their odds of a specific health disorder. The rapid availability of molecular genetic data for use by others, for reasons that were not apparent at the time of data collection, may additionally constitute a violation of individual privacy, on top of the usual data security concerns. In summary, genetic data sets provide information on factors that were previously unobserved or ignored, but since this information is poorly understood, great care must be given to ensure it is used appropriately.
Limitations and gaps
Knowledge on how genetic factors influence socio-economic outcomes is still in its infancy but is quickly evolving. Many of the advances over the last decade have been due to the availability of larger data sets and increased computational power. A companion study provides a much more critical and technical assessment of a subset of research to date in this area [11]. In a nutshell, the study speculates that advances in the statistical and econometric tools used to measure both genetic effects and gene–environment interactions will determine the speed by which future progress can be made.
Looking forward, careful research design may address many existing limitations. There appear to be substantial benefits from building on the same set of ideas that have transformed modern labor economics research when estimating relationships between outcomes and explanatory variables, including genetic factors. For example, studies that exploit variation in genetic factors between full biological siblings living in the same household will likely present more credible evidence on the role of specific genetic markers. Similarly, studies that ensure environmental factors are credibly exogenous will likely prove to be more convincing. Across all types of studies using genetic information, attention needs to be paid toward the way in which data were collected to reduce spurious associations related to differences across human subpopulations in genetic frequencies or differences in environmental conditions across birth eras. On the former, for instance, when analyzing data from a sample consisting of individuals from multiple ethnicities some genetic markers may be related to the outcome just because there is both a different outcome and different genetic prevalence due to ethnicity. Thus, there are benefits from potential interdisciplinary collaboration in research design for studies using genetic data.
Summary and policy advice
The mix of enthusiasm and trepidation regarding the potential social impact of genetic data is not unique to any government or individual. The enthusiasm is well-placed. For many health problems, there are enormous gains to be made from incorporating these data. For example, tools have been developed to better educate individuals on the trade-offs that different medical treatments could provide based on inheriting a genetic mutation that predisposes an individual to a specific disease. Similarly, for many social and educational outcomes, the behaviors under study exhibit a large degree of heritability. Research can provide guidance on one’s risk of certain poor outcomes based on their genetic makeup.
As an example, suppose that certain skills have a large genetic component. In an era with “skill-biased technological change,” individuals endowed with these genetically-linked skills may disproportionately complement new technologies, thereby increasing labor productivity. If research finds that genetic variation operates differently for men and women, then this may provide a new explanation for gender disparities in wages and occupation choice.
To respond to knowledge of one’s genetic makeup requires a rich understanding of the uncertainty inherent in these estimates as well as how these genes respond to different policies and interventions. Indeed, there is evidence that individuals respond in a heterogeneous manner to the same environmental influences based on their genetic makeup. Thus, one of the main policy challenges will be to understand how to utilize genetic data to remediate gaps in socio-economic outcomes. That said, an advantage of genetic information is that it is fixed at conception. This allows researchers to draw conclusions on the role of environments and policies, since one can see how individuals with similar endowments respond to different policies.
However, the trepidation is also justified. Like many other new sources of “big data” or artificial intelligence, there is the potential for misuse. Possible sources of abuse include not just the potential promotion of eugenics-style initiatives, but also discriminations by insurers or employers. However, given the significant potential benefits of incorporating these data with appropriate safeguards, it is hoped that policymakers can become more confident that the question to ask will shift from “Whether we should use data on molecular genetic factors?” to “How can we maximize the benefits while minimizing the harm?”
Acknowledgments
The authors thank an anonymous referee and the IZA World of Labor editors for many helpful suggestions on earlier drafts. Previous work of the authors contains a larger number of background references for the material presented here and has been used intensively in all major parts of this article [3], [4], [7], [8], [9], and [11]. Support from David Cesarini, Jason Fletcher, and J. Niels Rosenquist, among many others, as well as financial support from SSHRC is gratefully acknowledged.
Competing interests
The IZA World of Labor project is committed to the IZA Guiding Principles of Research Integrity. The author declares to have observed these principles.
© Weili Ding and Steven F. Lehrer
A quick introduction to the human genetic code
Source: Rosenquist, J. N., S. F. Lehrer, A. J. O’ Malley, A. M. Zaslavsky, J. W. Smoller, and N. A. Christakis. ‘Cohort of birth modifies the association between FTO genotype and BMI.’ Proceedings of the National Academy of Sciences of the United States of America 112:2 (2015): 354–359.