Elevator pitch
The open science and research transparency movement aims to make the research process more visible and to strengthen the credibility of results. Examples of open research practices include open data, pre-registration, and replication. Open science proponents argue that making data and codes publicly available enables researchers to evaluate the truth of a claim and improve its credibility. Opponents often counter that replications are costly and that open science efforts are not always rewarded with publication of results.
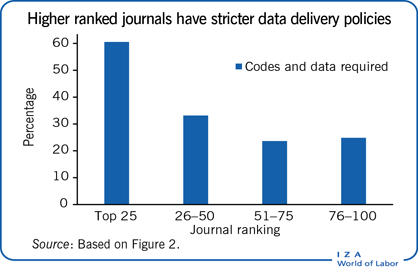
Key findings
Pros
Open science and research transparency offer the potential to improve empirical economic research’s credibility.
Sharing data and codes may allow other researchers to detect false-positive findings and increase the visibility and prominence of academic publications.
A growing number of free data repositories allow researchers to share information more effectively, thus eliminating the monetary cost of storing data and code.
A growing number of simple, low-cost, editorial policies may easily decrease the extent of publication bias.
Cons
Sharing data in a usable format requires considerable time and effort by knowledgeable people.
There is a lack of funding for, and to some extent interest in, replication studies.
Transparent practices such as pre-analysis plans may stifle researchers’ creativity and possibly prevent important breakthroughs arising from exploratory analysis.
There may be substantial upfront costs to transparency and openness and open science efforts are often not rewarded with publication of results.
Author's main message
Open science and research transparency can lead to improved credibility within empirical economic research, which represents a key input in economic policy design. Nonetheless, there remain concerns surrounding the costs associated with open science and the lack of incentives for transparent research. Despite these concerns, the potential benefits justify the efforts. Researchers and policymakers should thus pay close attention to recent developments in open research that may alleviate some of the main drawbacks, such as encouraging registered reports and editorial policies to promote transparent practices.
Motivation
There is an increasing demand for evidence-based policy in many countries. Economists are well suited to this endeavor, as they regularly work with large data sets and sophisticated methods to estimate causal effects. However, there is also growing concern that limited transparency may weaken the credibility and reproducibility of results. An increasing amount of evidence across economics suggests that scientific journals often publish only a subset of results, and that these may not be representative of the entire set of findings. For instance, results that find a significant effect of a particular program or policy may be more likely to end up published than null results.
In addition, a growing body of research shows that findings published in scientific journals may not be reliable or replicable. These issues cast doubt upon the credibility of published research in the eyes of policymakers and citizens. This is particularly relevant today, as, for instance, opinion poll data from the General Social Survey suggest that about 58% of Americans have only some confidence or hardly any confidence at all in the scientific community.
Discussion of pros and cons
Shift toward research transparency
When it comes to bias in published research, two fundamental problems concern cherry-picking of test statistics by authors and the lack of reproducibility of results. This is not a new issue, as it was already highlighted in a seminal study back in 1983 [1]. However, renewed critical scrutiny has led to a growing credibility problem within empirical economic research. Recent studies point out that up to 20% of marginally significant results may be false-positives, and that about half of the published papers in certain fields cannot be replicated [2], [3].
Perhaps in response to this credibility problem, research norms are changing quickly and largely for the better. Journal editors have proposed and implemented a number of promising solutions. For instance, many leading journals now require authors to post their data and codes for replication (Illustration). Researchers are also taking additional steps to make their research more transparent and reliable. A growing number of economists write down their hypotheses before conducting their analysis, as part of a so-called pre-analysis plan. This practice is intended to increase the level of confidence in a study's findings by minimizing researchers’ ability to cherry-pick results.
This recent shift toward open science and enhanced research transparency has not encountered much resistance in economics. A recent worldwide survey of PhD students in top programs and researchers who recently published in the top ten economics journals indicates that most economists think open science is important. Moreover, about 80% of the economists interviewed believe that publicly posting study instruments online is important for progress in the discipline [4]. This belief is equally important for both PhD students and professors.
However, while most economists seem to support the trend, there may be substantial upfront costs to enhanced transparency and openness. For example, sharing data in a usable format requires considerable time and effort from researchers [5]. In addition, there are at present no strong incentives to share such data. There is also a clear lack of funding for, and to some extent interest in, replication studies.
Though there may well be upfront costs, research transparency is also likely to incur considerable benefits later on. Many economists now use transparency tools like pre-analysis plans to conduct better causal inference. Moreover, sharing data and research programs may increase the visibility and prominence of academic publications.
Publication bias and cherry-picking (p-hacking) in economics
The recent shift toward research transparency has many causes. One of the key reasons is the growing number of studies documenting the extent of publication bias and p-hacking (i.e. cherry-picking) in economics. Publication bias in academic research occurs if the outcome of a study is related to the decision to publish. For instance, publication bias can be claimed if a study finding a statistically significant effect (or a surprising result) is more likely to get published than a study not finding a statistically significant effect (or unsurprising results), even if the two studies’ research design and execution are of the same quality. In other words, publication bias means that studies with a null result are less likely to be published than those with significant results, conditional on quality.
This is a big deal because policymakers and citizens use empirical evidence as an important input when making policy decisions and designing programs. If policymakers and citizens only see a subset of research, that is, findings showing a significant effect or surprising result, then it is unclear how much faith they should have in said research. In other words, if studies finding a significant effect of a given policy are the only ones getting published, then this would lead to a misrepresentation of the policy's real effect in the published literature.
A related issue is p-hacking (also known as cherry-picking or specification searching). While publication bias implies distortion or false representation within a given body of literature, p-hacking implies distortion within a given study. Imagine a researcher is interested in the effect of immigration on wages. The researcher will likely have access to a large data set and may estimate many different models. P-hacking would occur if the researcher restricts the sample to only a subset of the population or selects different covariates with the purpose of moving a test statistic across a statistical threshold. In other words, there is a large set of specifications available to the researcher, who then chooses to present only a subset of the results that he or she finds. By selecting those specifications that are statistically significant, the researcher paints an incomplete picture, in this example concerning the impact of immigration on wages, when in fact there might be no real underlying effect.
Different techniques have been developed to measure the extent of publication bias and p-hacking. The most well-known is probably the caliper test [6], which examines the number of published test statistics that are critical values lying just above and below a statistical significance threshold. This method argues that there should not be bunching on either side of the threshold since sampling distributions should reflect continuous probability distributions. Simply put, the likelihood of observing a finding just above a significance threshold should be about the same as the likelihood of observing a finding just below it. The extent of bias is measured as the excess number of marginally significant results.
Another method to measure p-hacking is to compare the distribution of test statistics in published articles to a range of other possible distributions such as a “student's t-distribution” [2]. The extent of p-hacking is defined as the excess number of test statistics in published articles in comparison to these other distributions around conventional significance thresholds. In the presence of p-hacking, the distribution of test statistics would have a humped shape around conventional significance thresholds, that is, a p-value of 0.05 or 0.01.
To give a sense of the scope of the problem, a 2016 study finds that 10–20% of tests were p-hacked [2]. This study collected data from three of the most prestigious economic journals over the period 2005–2011 and showed that the extent of p-hacking was larger for single-authored articles and papers by non-tenured researchers. In contrast, the presence of a theoretical framework was negatively related to the extent of p-hacking. The main results of this study are reproduced in Figure 1. Intuitively, the distribution of test statistics, in the absence of p-hacking, should have a decreasing pattern over the whole interval.
Figure 1 illustrates a (two-humped) density function of test statistics, with missing p-values between 0.10 and 0.25 and a surplus of marginally rejected tests. As mentioned, the distribution of test statistics, in the absence of p-hacking, should have a decreasing pattern over the whole interval, though this is not observed. As a reference distribution to identify p-hacking, the figure illustrates a student's t-distribution with one degree of freedom. In this sample, approximately 54% of test statistics were statistically significant at the 5% level. The local maximum around 1.96, p-value of 0.05, thus suggests that some test statistics were p-hacked.
Figure 1 also plots the distribution of test statistics, but only for studies whose method is a randomized control trial. This method is experimental and is considered by most as the gold standard for causal inference. For this subsample, about 37% of test statistics were statistically significant at the 5% level. This finding provides suggestive evidence that the extent of publication bias and p-hacking may be greater/smaller for some methods.
Another recent study documents the extent of p-hacking and publication bias for 25 top economics journals [7]. This study confirms the presence of p-hacking also in less prestigious journals and confirms that some methods are more prone to marginally rejecting the null hypothesis. For instance, field experiments and regression discontinuity design exhibit much less p-hacking than papers relying on instrumental variables. These results suggest that a well-executed experimental evaluation with an adequately sized sample may thus dispel (to some extent) concerns of p-hacking and support strong claims regarding the impact of a program or a policy [2], [7].
To sum up, there are now many studies that have documented issues related to publication bias and p-hacking in top and non-top economic journals. However, the fact that certain methods such as randomized control trials (RCT) are less subject to p-hacking provides suggestive evidence that improved research design may help improve the credibility of empirical economics research.
Replications and data availability policies
The findings of publication bias and p-hacking in economics literature suggest the need for fundamental changes in the way economics research is conducted and published. On the positive side, progress is being made; new initiatives have been implemented over the past 15 years to improve the field's research credibility. Possibly the most important initiative is requesting replication data and codes for published articles. The first general interest journal to systematically request data and codes was the American Economic Review. The practice became mandatory in 2004 and the policy states that the journal will only publish papers if “the data used in the analysis are clearly and precisely documented and are readily available to any researcher for purposes of replication.” Researchers must notify the editor at the time of submission if they cannot comply with this rule.
Many other economics journals have since implemented data and code availability policies. Figure 2 plots the percentage of top 100 economics journals with required and/or encouraged data availability policies. The x-axis plots journal rank in bins of 25 journals from higher rank journals to lower rank journals (based on RePEc's Aggregate Rankings) and the y-axis plots the percentage of journals that explicitly encourage data sharing on their website or have a data and code availability policy. Top-ranked journals are more likely than lower-ranked journals to have a data availability policy and it is more likely to be mandatory. Over 90% of journals in the top 50 have a data availability policy (required or encouraged), falling to just over 70% for journals ranked 51–75, and around 65% for journals ranked 76–100. Furthermore, about 60% of data policies in the top 25 journals are required, while just over 30% of journals ranked 26–50 are, and under 30% for journals ranked 51–100.
One issue often mentioned with data sharing is that it requires considerable time and money. Data storage used to be expensive and this may explain the lack of data sharing requirements by many journals. But it is now quite easy to store data. Many online sites offer free file storage space for researchers, thus eliminating the monetary cost of storing data and code. Journals could also use these free external data repositories.
While many leading journals now mandate authors to post their codes and data, there is unfortunately very little verification taking place to ensure that the provided data and code were in fact used to legitimately generate the published results. Moreover, very few replication studies are published in top economics journals. A recent study finds that from 1974 to 2014 only 130 replication studies were published in the top 50 economics journals [8]. This translates to a share of replications over the total number of published studies of about 0.1%.
One major issue with replications is the selection bias. It was recently estimated that only about half of the papers published in leading economics journals provide the data and code necessary for replication. This is due to many reasons, including the use of proprietary data, which makes replication impossible (or very costly). Another selection issue is that replications often end up going unpublished. Currently, researchers do not have (m)any incentives to do replication studies. Replications are rarely published, and publications are the “academic currency.” Furthermore, if only replications that find the opposite result from the original study are published, then replications would also suffer from selection bias.
A small number of large-scale replication efforts have been made in recent decades. One well-known large-scale replication is the Experimental Economics Replication Project, which attempted to replicate 18 studies published in two leading economics journals [9]. About 60% of the replications yield a significant effect in the same direction as the original study, although the effect size of the replication is often smaller.
Another large-scale replication was recently conducted in macro-economics [3]. The researchers attempted to replicate 67 papers in 13 economics journals. They obtained data and code for 40 of the 61 papers that did not rely on confidential data and successfully replicated about half of the 61 papers. Replication success is thereby defined as the ability to reproduce the original study's key qualitative conclusions.
The very small number of replications across the field may be related to a lack of funding. A number of studies point out that funding agencies should provide more money for replication studies and should consider introducing explicit replication policies [8]. Another way to increase the number of replications is to value and reward the work of researchers who conduct replication studies with publication of their studies.
Ways to make research findings more credible
In addition to the above, journal editors have recently implemented other practices to enhance the credibility and reproducibility of published results. For instance, eight health economics journal editors sent out an editorial statement which was aimed at reducing authors’ incentives to p-hack and to remind referees to avoid bias against studies that “have potential scientific and publication merit regardless of whether such studies’ empirical findings do or do not reject null hypotheses that may be specified.” A recent study shows that this simple, low-cost practice increased the number of published studies that do not reject the null hypothesis (i.e. find no significant effect) [10]. Importantly, the impact factor of these journals was not affected by this increase.
Another approach was recently implemented by the journal Psychological Science, which started offering badges to authors who reported open data and codes. The initiative has proven very successful, as it is estimated to have increased data reporting by about 35 percentage points [11]. This is an interesting example of a simple reward that could incentivize researchers to share their data.
Pre-analysis plans
Pre-analysis plans are typically written and registered before an intervention begins or before researchers gain access to the resulting data. Such plans outline the hypotheses to be tested, the sources of data, and the model specifications. They offer both advantages and costs [12].
One key advantage is that by pre-registering the analysis to be carried out before examining the data, p-hacking becomes less of an issue. Pre-analysis plans may thus reduce the likelihood that referees and editors suspect authors of cherry-picking their estimates. Moreover, pre-analysis plans help researchers to think through the data that they need and the hypotheses they will test. This is particularly important for field experiments, which often end up being very costly.
A study on a governance program in Sierra Leone demonstrates the usefulness of pre-analysis plans [13]. The authors show how the results of their field experiment could have been easily manipulated and erroneously interpreted. Fortunately, the authors had written down their hypotheses and their statistical code in advance. The use of a pre-analysis plan allowed them to bind their hands against p-hacking and to protect themselves against pressure from potentially non-neutral partners, for example, governments or non-governmental organizations.
The use of pre-analysis plans and pre-registration for field experiments is now common practice in (development) economics. In 2012, the American Economic Association's executive committee established a registry for posting pre-analysis plans. This registry currently lists over 2,000 studies across more than 120 countries.
The Journal of Development Economics, in collaboration with the Berkeley Initiative for Transparency in the Social Sciences, recently launched an initiative that offers authors the opportunity to submit a pre-analysis plan for review. The pre-analysis plan may then be accepted for publication before the results are known. This approach, known as “Registered Reports,” allows the author(s) to get an acceptance based solely on the research plan. One of the goals of this initiative is to directly deal with publication bias. Referees and editors (and the authors) have not yet seen the outcome of the study and are thus forced to make a publication decision based solely on the study's design, statistical power, and potential contribution to the literature.
Despite the clear advantages, pre-analysis plans also involve some significant challenges. One such challenge is that pre-specifying all the hypotheses to be tested in advance is nearly impossible. Another issue involves serendipity, or the lack thereof, which is an important part of research. Unexpected findings often lead to the development of new hypotheses and exploratory analysis can induce important breakthroughs. Both of these become less likely when using pre-analysis plans, as they inherently narrow the scope of research prior to the data-analysis phase. Similarly, by reducing the potential of exploratory learning, research questions with many unknowns become risky endeavors, which further reduces the possibility for unexpected outcomes.
Limitations and gaps
The large number of journals not requesting authors to post their data and codes combined with the small number of replication studies being conducted (or published) means that open science faces several considerable obstacles. A primary concern is that open science efforts are often not sufficiently rewarded. Established researchers are less accustomed to following open science practices, which often require increased time and effort, and may thus struggle to change their habits without the presence of strong incentives.
Another concern is that the increasing use of proprietary government or corporate data may stall the movement toward data sharing. The use of confidential data combined with growing concerns about data sensitivity present limiting factors in this regard. Considerable efforts to provide instructions on how to obtain such data and codes so that other researchers may replicate findings will be necessary.
Finally, some economists may fear the risk of peer appropriation if they share their data and codes. Building a data set takes a lot of time and effort and they may feel that other researchers will unfairly benefit from using their data set.
Summary and policy advice
The ongoing open science and research transparency movement represents a challenging time for economists and policymakers. The key question for proponents is how to transform these challenges into opportunities. How can researchers be incentivized to share their data sets and codes and to review and re-test the conclusions of previous studies? And how can incentive structures be altered to decrease the extent of publication bias in published literatures?
At least four major actions could increase transparency and credibility within economic research. First, while much progress has been made, it is still surprising that many scientific economic journals do not require (or allow) researchers to share their codes and data sets. The growing number of free and open source data repositories such as the Open Science Framework facilitate data sharing for preprints and working papers, but could also be used for published articles. Second, simple, low-cost, editorial policies could easily decrease the extent of publication bias. Third, replication grants and a greater recognition of the value of replications are necessary. And fourth, there is growing evidence that improved research design may make empirical economics research more credible, suggesting that authors themselves hold a key role in promoting the open science movement.
Acknowledgments
The authors thank anonymous referees and the IZA World of Labor editors for many helpful suggestions on earlier drafts. The authors also thank Taylor Wright for research assistance. Previous work of the authors contains a larger number of background references for the material presented here and has been used intensively in all major parts of this article [2], [7], [10].
Competing interests
The IZA World of Labor project is committed to the IZA Code of Conduct. The authors declare to have observed the principles outlined in the code.
© Cristina Blanco-Perez and Abel Brodeur