Elevator pitch
Non-experimental evaluations of programs compare individuals who choose to participate in a program to individuals who do not. Such comparisons run the risk of conflating non-random selection into the program with its causal effects. By randomly assigning individuals to participate in the program or not, experimental evaluations remove the potential for non-random selection to bias comparisons of participants and non-participants. In so doing, they provide compelling causal evidence of program effects. At the same time, experiments are not a panacea, and require careful design and interpretation.
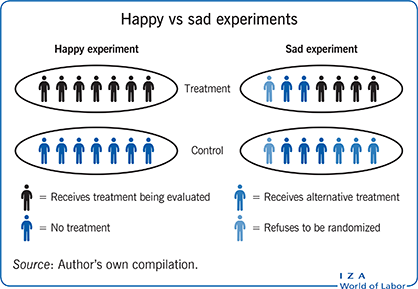
Key findings
Pros
Experiments solve the problem of non-random selection and thus often provide compelling causal evidence of program effectiveness.
Policymakers and other stakeholders find experimental methods easier to understand than many non-experimental evaluation methods.
Experiments are, in general, more difficult for researchers to manipulate than non-experimental evaluations.
Experimental data provide a benchmark for the study of non-experimental approaches.
Cons
In many experiments, interpretation is complicated by the fact that some of those assigned to the program do not participate in it, and, equally, that some of those assigned to not receive it may actually do so (or else receive a similar program).
Many experimental evaluations allow individuals to opt out of random assignment, which reduces the findings’ generalizability.
To fill the control group, experiments may require changes in program scale or that programs serve people they would not otherwise.
Local programs that resist participation in an experimental evaluation may not be representative, thus limiting generalizability.
Author's main message
Experimental evaluations of labor market programs (including evaluations that consider different ways of operating such programs) provide clear, compelling causal answers to policy questions of interest. Experiments require careful design, implementation, and interpretation to avoid potential weaknesses specific to experiments, and they remain subject to all of the usual issues that arise in any empirical study; nevertheless, they represent an extremely valuable tool in the program evaluator’s toolkit and remain underutilized throughout the developed world.
Motivation
What should a policymaker do when multiple non-experimental evaluations of the same active labor market program—all by reputable researchers without obvious bias, and all using the same underlying data source—produce impact estimates that imply very different policy conclusions about the program? Several decades ago, exactly this state of affairs occurred with respect to the US Comprehensive Employment and Training Act (CETA), leading to the first major experimental evaluation of an ongoing program, namely that of CETA’s programmatic successor, the Job Training Partnership Act (JTPA) [1]. In the succeeding decades, social experiments became commonplace in the US, influencing policy on topics as diverse as health insurance, police responses to domestic violence calls, sex education curricula, and teacher training. Experimental methods have also flourished in development economics and, more recently, social experiments have spread to (parts of) Europe. Yet skepticism remains in the academic community, among program administrators and caseworkers, and in the press (and, of less concern, among advocates for policies on the losing end of experimental evaluations). This article considers the broad case for social experiments—there is more to it than just avoiding selection bias—as well as their limitations.
Discussion of pros and cons
Experiments provide many benefits to program evaluators
When it comes to evaluation, the fundamental problem concerns the non-random selection of participants into programs (and jurisdictions into policies, and so on). This selection issue means that comparisons of the outcomes of participants with those of non-participants will combine, in an unknown proportion, both the causal impacts of the program and differences that would have emerged even without it. A well-executed experimental evaluation with an adequately sized sample dispels such concerns about non-random selection and so supports strong causal claims regarding the impact of a program on the randomly assigned population.
Even with a strong non-experimental evaluation that applies state-of-the-art methods to high-quality data, a haze of doubt always remains around causal claims. Put differently, non-experimental evaluations always raise concerns about non-random selection into a program. Each combination of non-experimental method and observational data on a comparison group of non-participants solves the problem of non-random selection under particular assumptions, but those assumptions always remain at least partly untestable.
In contrast, experiments directly solve the problem of non-random selection into treatment by randomly forcing some individuals who would have otherwise participated in a program not to do so. Experiments provide this important causal service whether they seek to estimate an average treatment effect or a “structural” parameter, such as an elasticity of labor supply, as in the US Negative Income Tax experiments. Thus, while experiments require assumptions about some things (as will be discussed below), they do not require assumptions about the process of selection into the program in order to provide a compelling estimate of the causal effect for the randomly assigned population.
Additionally, the conceptual simplicity of experiments serves to make the evidence that they provide easier for non-specialists to understand and, as a result, more convincing to them. As well-known economist Gary Burtless explained: “Because policymakers can easily grasp the findings and significance of a simple experiment, they concentrate on the implications of the results for changing public policy … Politicians are more likely to act on results they find convincing” [2]. Most people understand how randomization leads to a compelling case for causality, especially in experiments that do not embody too many of the limitations discussed below.
Moreover, experiments reduce the potential for conscious or unconscious researcher bias to affect impact estimates. Researchers applying non-experimental methods typically have more degrees of freedom in choosing how to conduct their analysis. For example, in an evaluation using matching methods, the researcher chooses both the set of matching variables and details of the matching procedure. Choices that lead to substantively meaningful differences in the estimated impacts may appear equally plausible to even expert readers, as in the CETA evaluations mentioned above. Experiments do not make manipulation impossible, but they typically reduce the potential for it.
Finally, experiments have important knowledge spillovers. One large and growing literature uses experimental impacts as benchmarks for examining the performance of alternative combinations of non-experimental methods and data. For example, a set of papers uses the experimental findings from the US JTPA experiment to study various aspects of non-experimental evaluation design [3]. These include the value of particular types of conditioning variables, the choice between comparing outcome trends and outcome levels, and the choice of whether or not to locate comparison groups in the same local labor markets as participants. By comparing non-experimental estimates obtained using different econometric methods, different comparison group data, and different sets of conditioning variables to the experimental estimates, these studies provide evidence on what works and what does not, and this evidence has proven valuable in more recent non-experimental evaluations. Because the higher financial and political costs of experiments mean they will never fully displace non-experimental evaluations, using experiments to learn about how to design more compelling non-experimental evaluations represents an important contribution.
Potential drawbacks to the use of experiments in program evaluation
Despite their clear benefits, experiments have some unique features relative to non-experimental program evaluations that can lead them to produce inferior estimates. In addition, random assignment may exacerbate problems that also arise in some non-experimental evaluations. However, not all of the drawbacks apply to all experimental designs, and most limit “external validity,” which is the ability to generalize the experimental findings to other populations, rather than “internal validity,” which is the causal interpretation for those actually randomized.
First, consider the interpretational issues that arise when not everyone in the treatment group receives the program, and/or some individuals in the experimental control group receive it or some similar program (despite the specific intention that they should not). Some individuals assigned to the treatment group may fail to participate (“no-shows”), or to participate fully (“dropouts”). No-shows (and dropouts) may arise because treatment group members find a job, or move, or go to jail, or just learn more about a voluntary program and decide they do not like it. Similarly, control group members may defeat the experimental protocol by enrolling in the program, or, more commonly, they may receive the same, or similar, services from another source or with alternative funding; the literature calls this “control group substitution.” The potential for no-shows and dropouts depends on features of the experimental design, e.g. the temporal lag between random assignment and service receipt, and on the nature of the treatment. Treatments that manipulate the budget set (e.g. an earnings subsidy) usually do not have these issues, because individuals receive them no matter what, while treatments that involve service receipt typically do. Control group substitution also depends on the programmatic environment: centralized environments where only one agency provides a given service type will have less of it. Empirically, many experimental evaluations exhibit treatment group no-shows (and dropouts) and control group participation in the same or similar programs at substantively relevant levels [4].
The literature offers two main approaches to deal with this assignment issue. The first approach reinterprets the experimental contrast—the difference in mean observed outcomes between the experimental treatment group and the experimental control group—as the mean impact of the offer of treatment rather than the receipt of treatment. The literature calls this the “intention to treat” (ITT) parameter. In the context of a voluntary program, where the government can offer the program but not require it, the mean impact of the offer answers a relevant policy question: “What is the mean impact of adding one option to the set of programs already available?” That answer may differ quite substantially from the policy question that gets answered in an experiment wherein every treatment group member gets treated and no control group members do, namely: “What is the mean impact of treatment versus no treatment?”
The second approach divides the experimental mean difference by the difference in the fraction of individuals receiving the program in the experimental treatment group and the fraction of those receiving something similar to it in the control group. For example, in an experimental evaluation wherein the probability of participating in the program in the experimental treatment group equals 0.6 and the probability of a control group member participating in a very similar program equals 0.2, then the experimental mean difference gets scaled up by 0.6 – 0.2 = 0.4. To see the intuition, suppose that both the program and its close substitute have a common impact of 10 on everyone, and that the members of both groups all have the same untreated outcome of 100. In this case, the mean outcome in the treatment group equals 106 = 100 + (0.6)(10) and the mean outcome in the control group equals 102 = 100 + (0.2)(10). The experimental mean difference equals 4 (= 106 – 102). Dividing the experimental mean difference by the difference in the probability of participation recovers the common impact of 10 = 4 / 0.4. In the more general case where program impacts differ across individuals, the rescaled experimental mean difference provides (under certain, usually plausible, assumptions) the mean impact on the compliers—so-called because they comply with the experimental protocol by receiving treatment when assigned to the treatment group, and not receiving treatment when assigned to the control group. The mean impact on the compliers informs a cost–benefit analysis of the ITT policy question, but has nothing to say about the impact of the program on those who would take it, or something like it, when assigned to either the treatment group or the control group.
In many institutional settings, individuals must explicitly agree to participate (i.e. to opt in rather than to opt out) in a study using random assignment but may be included in non-experimental studies without explicit consent. In practice, some individuals will decline to undergo random assignment. Such individuals may have very high levels of risk aversion, or philosophical objections to random assignment, or just be contrary or confused. The number of such people tends to be small (and can be made smaller by thoughtful marketing efforts) but not trivial. The very limited empirical evidence on this phenomenon raises the possibility that treatment may have a different average impact on individuals who exclude themselves than it does on those who agree to participate, implying that the experimental impact provides an imperfect guide to the impact for the group of individuals who would have participated in the program in the absence of the experiment [5].
In many contexts, some or all of the individuals in an experiment will know that they are taking part in an evaluation that might have policy consequences, while individuals in a non-experimental evaluation will not. This knowledge increases the potential for changes in behavior designed to alter the outcome of the experiment and thereby influence policy. For instance, the literature includes examples of caseworkers who ignored information on optimal training assignments from a statistical treatment rule, perhaps because they did not see the value in them, or possibly because they viewed the statistical treatment rule as a threat to their jobs and thought they could kill it by behaving in ways that would lead to a null finding in the impact evaluation [6]. Similarly, teachers in the control group of an experimental evaluation in which the treatment group receives financial performance incentives might, for ideological reasons, work extra hard. These kinds of responses undermine the integrity of an experimental evaluation and render its findings of limited value for policy.
Experimental evaluations of existing programs (as opposed to, say, demonstration programs) face a trade-off between the size of the control group and the desire to maintain the program at the scale at which it operates in the absence of the experiment. Consider a program that serves about 1,000 participants per year. Randomly assigning half of those participants to a control group reduces the number served to 500. This might imply layoffs of program workers or, if the workers are kept, that the individuals randomly assigned to the treatment group receive better service than they would have in the absence of the experiment. The former may cause political trouble or imply the loss of valuable employees that the organization would like to have around after the experiment, while the latter changes the nature of the program and so renders the experimental estimates a problematic guide to the impact of the program as it usually operates. Alternatively, in some contexts the program may have the option of recruiting additional participants from among those it would not have served in the absence of random assignment. In the current example, this would allow keeping the number served at 1,000. But if the program has a different average impact on the newly recruited participants than on those that the program would have served when operating normally, then the experimental estimates will again provide a misleading picture of the program’s impact under normal conditions.
A final drawback with experiments concerns local cooperation in decentralized programs. Consider the case of an active labor market program operated via a network of local employment centers. An evaluation aiming for maximum generalizability would either consider all of the centers or a (sufficiently large) random sample of them. In a non-experimental evaluation, getting the chosen centers to participate will typically be easy because participation will likely require little from them other than perhaps sharing some data. In contrast, obtaining local cooperation in an experiment poses a larger challenge due to the much higher costs imposed by a random assignment evaluation: selected sites must set up, operate, and document random assignment, and they must deny services to individuals they would have served otherwise. Even in environments where the central administration need not ask local offices to participate, implementation of random assignment requires a relatively high level of local cooperation. In the US JTPA experiment, evaluators had to contact about 200 of the 600 training centers (and had to offer substantial side payments and other concessions) in order to get 16 to participate in the experiment [7]. Needless to say, concerns about the reasonableness of generalizing the impacts obtained from these 16 centers plague discussions of the experimental findings.
Limitations and gaps
Experiments, like most non-experimental evaluations, rely on the (usually implicit) assumption that the program being evaluated does not affect individuals who do not participate in it. Put differently, most experimental evaluations assume no spillovers to individuals in the control group or in the larger non-participant population. What might such spillovers look like? They might take the form of changes in the prices of particular types of skills in the labor market due to a program-induced increase in their supply. Adding 100 additional hairdressers or welders to the labor market in a small city may lead to reduced wages for these skills, not just for the trainees but for incumbents as well. A program that trains some teachers in a school in new instructional techniques may experience “informational spillovers” if the teachers share the new ideas with their untreated colleagues. A program that teaches some unemployed workers how to search for work more effectively, say by improving their interviewing and resume-writing skills, may lead them to take vacancies that, in the absence of the training, would have gone to non-participants. In this last case, the program slows the return of non-participants (most of who will not, in general, belong to the control group) to employment. To the extent that most (or all) of the affected non-participants lie outside the control group, the spillovers matter for thinking about the social cost–benefit calculation, but do not have a major effect on the causal interpretation of the experimental estimates for those randomly assigned.
The (limited) available evidence suggests the potential for substantively large effects on non-participants, large enough in some cases to overturn the conclusion of a cost–benefit analysis that ignores them [8]. One notable evaluation of an active labor market program estimates effects on non-participants via a multi-level experimental design. The top level randomly assigns the fraction of the eligible population served in the local labor market. In some places, most get served, whilst in others only a modest fraction do. The bottom level randomly assigns eligible unemployed workers to the program in the proportion determined by the top level randomization. If the experimental impact at the local labor market level increases with the fraction assigned to the program, this signals the importance of negative spillovers on the non-participants [9]. Most evaluations will lack the financial and organizational (and political) resources to mount such a design; in such cases, the evaluator should either make a substantive argument for the unimportance of effects on non-participants in their particular context, or they should consider the sensitivity of any cost–benefit calculations to reasonable estimates of effects on non-participants drawn from the broader literature.
Additionally, experimental data (as with observational data) do not directly identify all the parameters that an evaluator might care about [10]. For example, some parameters relate to choices made after random assignment, choices that the treatment may affect. For instance, the effect of a training program on wages holds great substantive interest, but wages are observed only for the employed. A comparison of the wages of employed treatment group members with the wages of employed control group members conflates the effect of treatment on wages with the (likely selective) effect of the program on employment. For example, suppose that the treatment group is one-third employed at a wage of 12, one-third employed at a wage of 10, and one-third not employed, while the control group is one-third employed at a wage of 10, and two-thirds not employed. In this scenario, the program increases the wages of one-third of the individuals by 2, but because it also increases the employment of workers earning 10, a comparison of the wages of employed treatment and control group members yields an impact on wages of only 1.
Another limitation arises because experiments often provide only limited information about causal mechanisms (i.e. about where the causal impacts they estimate come from), and even such limited insight usually requires some clever combination of evaluation design, program design, and data collection. Experiments share this feature with many non-experimental evaluations, but the frequent reliance solely on administrative data in experiments exacerbates the problem. Consider an evaluation of an active labor market program for the unemployed that combines frequent, relatively unpleasant meetings with caseworkers (a “leisure tax”) with separate high-quality instruction in job search techniques. An experimental evaluation conducted using only administrative data on earnings might find a compelling, substantively and statistically meaningful effect on earnings while shedding no light on whether the meetings or the job search instruction (or some combination of the two) drove the impacts.
To see how program design can help, suppose that the unemployed learn about the required meetings in advance and that their job search assistance takes place after the first caseworker meeting. In this scenario, the timing of the impacts on earnings may speak to the question of mechanisms. In particular, earnings impacts prior to the first meeting suggest the importance of threat effects [11]. Alternatively, the collection of data on the quality and quantity of job search allows the experimental estimation of treatment effects on those mediators; lack of change in job search behavior following the job search instruction strongly suggests that any treatment effects on earnings result from the caseworker meetings. In the other direction, data showing that most of the unemployed skipped their meetings without any sanction supports the view that the job search instruction drives any impacts. The general point concerns the ability of data on behaviors related to specific mechanisms to provide suggestive evidence on the importance (or non-importance) of those mechanisms.
Yet another issue arises due to some observers seeing ethical challenges associated with experiments, particularly those that involve randomly denying the control group access to potentially valuable services. In a sense, control group members bear real costs in order to help produce the public good of knowledge about program effectiveness. Evaluators can respond to these concerns in several different ways. First, just as individuals who surrender their property for public goods such as roads get compensated, control group members could be compensated as well (though this might have its own behavioral effects). Second, experimental evaluations can focus on cases of real ignorance of program effects, so that it is not clear to the designers of the experiment whether assignment to the control group means missing out on a great program or not wasting time and energy on an ineffective one. Third, in the case of over-subscribed programs, evaluators can emphasize the fairness of random assignment as a means to allocate scarce program slots. Fourth, experimental evaluations can focus on designs, such as randomization at the margin of participation or randomization of incentives to participate, that (at least in part) mitigate the ethical concerns (while at the same time changing the substantive meaning of the experimental impact estimate). Fifth, experimental evaluations can focus on aspects of program operation (e.g. the number and timing of meetings with caseworkers) or on alternative service mixes (e.g. job search assistance versus training) rather than on contrasts between services and no services [12].
Finally, randomization provides a compelling solution to just one of the many vexing issues that arise in attempting to wring evaluative knowledge out of data, that of non-random selection into programs. That issue is a particularly important one, but the many other issues that plague any empirical evaluation still arise in experiments. For example, experiments that rely on survey data often end up with different response rates from their treatment and control groups. Depending on the nature of this differential attrition, it may bias the impact estimates. Outliers (i.e. unusual observations), whether they represent measurement error or just unlikely draws, may sway evaluations that look only at conditional means. Differences in measurement error correlated with treatment status may bias impact estimates, as when the treatment under study moves workers from the informal sector to the formal sector and the administrative data used to measure earnings outcomes includes only formal sector jobs. And so on.
Summary and policy advice
Experiments produce incredibly valuable exogenous variation in the receipt of programs or in terms of their design and operation. This variation leads to compelling causal estimates that answer many questions of academic and policy interest, and which policymakers and taxpayers can understand and appreciate.
At the same time, experiments are not a substitute for thinking. They have limitations that require careful design and thoughtful empirical analysis, including consideration of the sensitivity of the experimental estimates across many dimensions. Deriving the policy implications from experimental data requires not only close attention to the empirical analysis, but reference to institutional knowledge and the relevant economic theory as well. Random assignment alone does not guarantee a high-quality, policy relevant, or scientifically informative evaluation. Indeed, one can easily find high-quality non-experimental evaluations that provide more credible evidence than low-quality experiments.
The bottom line: despite their limitations, experiments have great value. They remain underutilized around the world, particularly outside the US. Room for improvement exists on the quality dimension as well as the quantity dimension, especially with respect to the issues of external validity, control group substitution into similar programs, effects on non-participants, and attention to mechanisms. Further methodological research on these dimensions, as well more attention to them in evaluation practice, both represent sound investments.
Acknowledgments
The author thanks three anonymous referees and the IZA World of Labor editors for many helpful suggestions on earlier drafts. Previous work by the author contains a large number of background references for the material presented here and has been used intensively in all major parts of the article [3], [4], [8], [10], [11].
Competing interests
The IZA World of Labor project is committed to the IZA Guiding Principles of Research Integrity. The author declares to have observed these principles.
© Jeffrey A. Smith