Elevator pitch
There is growing concern that much of the empirical research in labor economics and other applied areas may not be reproducible. Correspondingly, recent years have seen an increase in replication studies published in economics journals. Despite this increase, there are many unresolved issues about how replications should be done, and how to interpret their results. Replications have demonstrated a potential for clarifying the reliability and robustness of previous research. Much can be done to encourage more replication research, and to exploit the scientific value of existing replication studies.
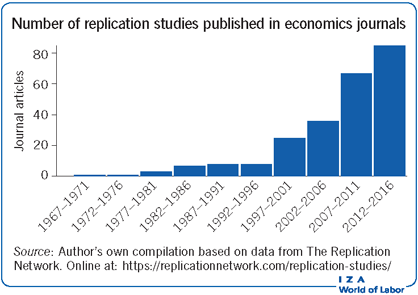
Key findings
Pros
Replications can help to confirm that an original study was done correctly.
Replications can be used to determine if the findings of a study generalize to other, similar settings.
Replications have demonstrated their usefulness in assessing the results of previous, high-profile research.
Cons
There is no consensus about what a replication is, nor about what constitutes a “successful replication.”
Little is known about how frequently replications occur, or how often they confirm or disconfirm the original studies.
There is no standardized system to collect, categorize, and link replications to the respective original studies.
Author's main message
Empirical research inherently involves uncertainty. Across studies, this uncertainty is reflected in the fact that many studies investigating similar subjects report different results. Efforts to reduce this uncertainty can help solidify what is known, and unknown, in labor economics. Replication is one such effort. While the concept is seemingly straightforward, conducting and interpreting replications is difficult. Researchers and policymakers alike could benefit by improving access to original data sources, encouraging journals to publish more replications, and establishing a cataloging system to link replications to the original studies.
Motivation
The evidence on the reproducibility rate of scientific research is thin but alarming. In 2016, an article in the journal Nature reported the results of a survey in which 1,576 researchers were asked about the reproducibility of scientific findings. In response to the question, “Is there a reproducibility crisis?” 52% responded “Yes, a significant crisis.” More than 70% reported failing to reproduce another scientist’s experiments at least once.
Probably the most famous attempt to determine a discipline’s reproducibility rate is the Open Science Collaboration’s study on reproducibility of experiments in psychology, published in the journal Science in 2015. This collaborative effort attempted to replicate 100 experiments published in three top psychology journals. While acknowledging that there is no objective measure to determine “replication success,” the authors judged that less than 40% of the replications were able to successfully reproduce the original result.
Discussion of pros and cons
Tracking replications
There are no commonly accepted criteria for a study to be classified as a “replication.” The main issue is how closely a follow-up study must adhere to an original study in order to be classified as a replication. Does it need to use exactly the same data? If so, does that mean that experiments are inherently non-replicable because they use different subjects?
Suppose the original data are unavailable and a follow-up study tries to reproduce the data from the same sources. How much is the re-created sample allowed to differ before the study is no longer a replication? And suppose a study tries to estimate exactly the same phenomenon as the original study, targeting the same population, but using a different data source? For example, suppose an original study uses survey data to determine the effect of minimum wages on employment for a given community. A follow-up study investigates the same target population and community using administrative employment data. Should it be considered a replication?
In some cases, the effort to reproduce a finding from another study may be tangential to the main purpose of a research project. For example, a study of real wages may report that it estimates real wages to be pro-cyclical, noting the similarity to a previously published study. Does that make it a replication? Suppose the main purpose of the study was to investigate employment, so that the observation about real wage behavior was incidental? More generally, how much effort needs to be expended to establish the veracity or robustness of a previous study in order for that research to be classified as a replication?
In addition to the problem of classification, there is also the problem of compiling the results of replication research. There is no authoritative source that collects and records replication studies. As a result, any measure of the frequency of replications will be incomplete and controversial.
How common are replications?
One approach to measuring the rate of replication involves selecting a sample of journal articles, finding all studies that cite them, and then categorizing the citing articles as to whether they are “replications.” A recent effort using a sample of 70 articles from the centenary volume of the American Economic Review found that 20 of these had been “replicated,” where a replication was defined as “any project that reports results that speak directly to the veracity of the original paper’s main hypothesis” [1]. However, only eight of these had replication as their main purpose. Another recent effort comprised a sample of ten of the most cited papers in empirical labor economics [2]. To be classified as a “replication,” citing papers had to analyze aspects of the original study using some of the same data. All ten papers had been replicated at least once, with seven having been replicated at least five times.
Of course, the article samples above are highly selective. Replication Wiki is a website that tries to keep an up-to-date account of replication studies. As of August 2017, it reported 346 replication studies from both published and unpublished sources. The Replication Network is another website that compiles information about replication studies. It defines a replication study as “any study published in a peer-reviewed journal whose main purpose is to verify the reliability of a previously published study.” It records 242 published replication studies in economics journals, going back to 1967.
New Economics Papers (NEP) is part of the Research Papers in Economics (RePEc) collaboration that collects and disseminates new working papers across a large number of economic disciplines. Labor economics is represented by two NEP collections: Supply, Demand, and Wages (NEP-LMA) and Labor Economics (NEP-LAB). The archives are searchable and together list 26,752 documents going back to 1999. Searching the titles and abstracts for all documents containing the string “replic*” produces 242 working papers, of which 26 are “studies whose primary purpose is to verify the findings of a previous study.” By this measure, only 0.1% of all working papers in labor economics replicate previous research.
As noted above, there are many studies that replicate results from previous research but whose main purpose is other than verification of a previous study. These studies would largely be excluded from the above measure. Tracking these is a challenging undertaking, making it difficult to construct an overall measure of the rate of replication in labor economics.
In conclusion, replications—at least when defined as studies whose primary purpose is to verify previous research—are generally very rare in labor economics. This is not necessarily a bad thing. Given scarce research resources, most studies should probably not be replicated. Replication efforts should be focused on high prestige, influential papers, and here the replication rate appears to be high, at least for the most elite studies in the discipline.
Types of replications
“Replication” is a general term that is applied to a number of different activities. There are many ways of describing and categorizing replications, with no consensus about which is best. The following classification, while somewhat original, borrows from a number of sources (Figure 1). It identifies six types of replications, based on the nature of the data being studied and the methods used to analyze them. However, it should be emphasized that there is no generally accepted nomenclature, and there are some researchers who would object to one or more of the types/activities below being called “replications.”
The first, reproduction, is the simple (or sometimes not so simple) act of attempting to duplicate the findings from an original study. Its purpose is to verify that computations in the original study were done correctly.
Robustness analysis—same data set, is the second and applies different analyses to the same data to determine whether reasonable alternative procedures produce the same results. For example, one might eliminate outliers to determine if the same result occurs; or apply a different estimating procedure to the same data, say, by using an alternative weighting scheme; or use a different variable combination in the regression equation.
Third, repetition follows in the same spirit as reproduction, except that it examines a different data set, while targeting the same population. An example would be the minimum wage study mentioned above, where the original study used survey data and the follow-up study used administrative data. Another example would be an experiment carried out using the same design as the original study, but with a different set of subjects drawn from the same population.
Fourth, robustness analysis—same population incorporates many of the same kind of estimation procedures included in robustness analysis—same data set but with a different data set. It also includes a number of analyses that would be difficult to perform using the original data set, such as addressing omitted variable bias/endogeneity with variables not available in the original data set.
Fifth, extension examines whether the findings from an original study extend to a different but related population. For example, an original study that examined the effects of immigration on local employment in one country might be replicated by using the same methods to analyze the effects of immigration in another country. A replication that updated data from the original study could be either a repetition or an extension, depending on whether the more recent data were considered to be drawn from the same population as the original study.
Finally, sixth, robustness analysis—different population applies different measurements or analyses to a sample drawn from a population related to, but different from, that of the original study. An example here would be an analysis of the relationship between abortion and crime that was patterned after studies in the US, but applied to data from other countries with different abortion laws using different measures of crime.
Obviously, the lines separating the different categories are blurry, with some being particularly fuzzy. Complicating the picture is the fact that replication studies often do more than one kind of replication, so they are not so easily pigeonholed.
To get a better idea of the kinds of replications done in labor economics, the sample of 242 replication studies listed at The Replication Network website were analyzed, with 46 identified as belonging to labor economics. Roughly half of these made a good faith effort to exactly reproduce the original study, using the same data (or nearly the same data), same model specifications, and same estimation procedures. Approximately 60% made an attempt to extend the original study (e.g. by checking whether the results were valid for a different country, or a different time period), or conducted a robustness analysis using a different model specification or estimation technique. A number of studies did both. Of the 46 studies, 12 were published in the Journal of Human Resources, with most of these being published before 2000. The next most frequent journal outlet was the American Economic Review, with six replication studies.
Do replications generally confirm or disconfirm the original studies?
Most research investigating reproducibility rates have focused on experimental studies, where it is far easier to recreate the original research environment, and most of these have been outside of economics. A recent effort from 2015 tried to gauge the replication rate in the non-experimental economics literature [3]. The authors selected 67 papers from a variety of top economics journals and then attempted to replicate the results. Even after contacting the original authors for assistance, they were only able to successfully reproduce the original findings in about half the cases. It should be noted, however, that in most cases failure to replicate was due to data being unavailable.
A different approach was taken by another 2015 study [4]. The authors reviewed 162 replication studies published in peer-reviewed economics journals. They categorized a replication as “successful” if, in their judgment, the original authors would have reached the same conclusion had they obtained the results reported by the replication study. Among these published replication studies, they report that 66% were not “successfully replicated,” with another 12% being “mixed,” unable to confirm at least one major finding of the original study.
Using the same standard of replication success employed by [4], the above-mentioned sample of 46 labor economics replication studies was analyzed further. Of the 46 studies, 34 (approximately 74%) did not “successfully replicate” the originals. Another seven were “mixed.” This corresponds to an overall reproducibility rate of approximately 10%. It is unlikely the true reproducibility rate in labor economics is really this low, as there is evidence that journals are more likely to publish replication studies when they overturn the results of the original study. Even so, this low rate of reproducibility is concerning.
While the categorization of replications into “successful” or “unsuccessful” has a place, there are many nuances to replications that are blurred by this binary classification. Replications can overturn findings from a previous study. But they can also identify weaknesses and ambiguities in the original study, or even lead to new interpretations of the original study’s results.
Replication case study #1: Abortion and crime
In 2001, John Donohue and Steven Levitt published a sensational study in the Quarterly Journal of Economics that claimed that an earlier rise in abortion was a major contributor to decreased crime rates observed in the US in the 1990s [5]. They hypothesized that abortion reduced the number of unwanted children, and that these unwanted children, had they been born, would have committed crimes at a higher rate than wanted children (“selection effect”). The main analysis consisted of panel data on US states from 1985 to 1997. The dependent variable was a measure of crime, and the key explanatory variable was “effective abortion rate,” which measured the weighted impact of earlier abortions on current arrestees.
In 2008, a replication of the above study claimed to refute the existence of a relationship between abortion and crime [6]. First, the authors found an error in the original study’s computer codes that reduced, but did not eliminate, the estimated relationship between abortion and crime. Second, they substituted an alternative, arguably better measure of crime. Lastly, they included state-specific time trends to capture the effect of changes in states that preceded the legalization of abortion. When all was said and done, the replication found no evidence of a selection effect on crime. The original study’s authors responded by presenting new evidence based on an improved measure of “effective abortions.”
While sifting through the US data resulted in different conclusions based on particular regression specifications, each with its own strengths and weaknesses, perhaps the most persuasive argument against the original study was provided by yet another replication. This second replication attempted to reproduce the original findings using data from England and Wales [7]. The study was able to generate a negative relationship between abortion and crime along the lines of the original results. However, a series of robustness checks to address labor market conditions, immigration, demographics of aborting mothers, and other factors consistently resulted in an insignificant relationship between crime and abortion.
In this case, it can be concluded that replications show that the negative relationship between crime and abortion becomes insignificant when the influence of other factors is controlled for. Accordingly, they have served to substantially weaken the argument that the legalization of abortion reduced crime.
Replication case study #2: Minimum wages
The literature on the effect of minimum wages on employment is vast. One strand that has received much attention has focused on individual states (case studies). In 1994, David Card and Alan Krueger published a landmark study in the American Economic Review on the effect of an increase in New Jersey’s minimum wage [8]. The higher wage took effect on April 1, 1992. In anticipation of this increase, the authors conducted telephone interviews with 410 fast-food restaurants in New Jersey and Pennsylvania (the control group) in the two months preceding the event. They followed this up with a subsequent survey of the same restaurants later in the year. Based on the responses they received regarding employment before and after the increase, the authors concluded that the increase had, at worst, no impact on employment, or, at best, a positive impact.
A study from 2000 replicated the original, using state payroll records that drew from the same geographic areas and restaurant chains [9]. The authors concluded that the minimum wage increase reduced employment in New Jersey relative to Pennsylvania. A subsequent reply from the original study’s authors questioned the more recent sample, and concluded that the differences were due to (i) unrepresentativeness of the Pennsylvania sample, and (ii) the fact that the replication study measured employment by hours worked, while the original measured employment by number of workers.
Based on this example, it can be said that replications using different data sources and measures have produced conflicting conclusions regarding the employment effects of New Jersey’s 1992 minimum wage increase. In this case, the results from replication are inconclusive.
Replication case study #3: Racial discrimination
An innovative approach to identifying racial discrimination in labor markets is the use of audit studies, in which researchers enlist subjects to pose as job applicants for real job vacancies. Subjects are selected to be identical in every respect except ethnicity. Any observed differences in labor market outcomes across races are then attributed to employer discrimination.
To get around the difficulty of selecting “identical” job applicants, some studies have resorted to using resumes. One of the most famous of these studies is by Marianne Bertrand and Sendhil Mullainathan, published in the American Economic Review in 2004 [10]. The authors created a set of fictitious job applicants with names chosen to sound African-American (e.g. Lakisha and Jamal) or white (e.g. Emily and Greg). The names were randomized across fake resumes and these were sent to help wanted adverts that appeared in Boston and Chicago newspapers in the US. Dedicated phone lines were created to receive callbacks for job interviews. A total of 4,870 resumes were sent, with half coming from “African-American” job applicants, and half coming from “white” applicants. The study found significant differences in the callback rates by race. Overall, white applicants received approximately 50% more callbacks than African Americans, with little difference by gender or city.
Two studies highlight how replications can change the interpretation of an original study. The first of these was modeled after the original study, but with one major difference [11]. In addition to African-American and white sounding names, it included a third group of fictitious job applicants with “foreign-born sounding” names. The authors found that while African Americans received fewer callbacks than white applicants, there was little difference in the callback rates between African Americans and foreign-born candidates. They hypothesize that the driving force was not so much racial discrimination, but “ethnic homophily,” discrimination against any group that is perceived as being different from the ethnic majority.
In 2015, the American Economic Review published another correspondence study, this one focusing primarily on the perceived value of different kinds of postsecondary degrees [12]. As part of that study, the authors studied the effect of race by including white and non-white (i.e. African-American and Latino) sounding names. The study found no difference in the callback rates by race. While a number of potential reasons were given for this discrepancy, one possibility is that the names used in the original study confounded ethnicity with socio-economic status [13]. So, while Lakisha and Jamal may be African-American sounding, they may also be perceived as communicating a lower socio-economic status than Emily and Greg, and the latter may be relevant for successful job performance.
The takeaway in this case is that replications of the original study on racial discrimination have identified alternative explanations that moderate interpretation of the original authors’ evidence for racial discrimination in the labor market.
Limitations and gaps
A major limitation to the usefulness of replications is that there is no accepted criterion for determining “replication success.” This is partly due to the fact that there is no generally accepted definition of a replication. But the issue is even more fundamental than that, and perhaps can best be illustrated with an example. Suppose a study reports that a 10% increase in unemployment benefits is estimated to increase unemployment duration by 5%, with a 95% confidence interval of [4%, 6%]. Two subsequent replications are undertaken. Replication #1 finds a mean effect of 2% with corresponding confidence interval of [1%, 3%]. Replication #2 estimates a mean effect of 5%, but the effect is insignificant with a corresponding confidence interval of [0%, 10%]. In other words, consistent with the original study, Replication #1 finds that unemployment durations are positively and significantly associated with unemployment insurance benefits. However, the estimated effect falls significantly short of the effect reported by the original study. Replication #2 estimates a mean effect exactly the same as the original, but due to its imprecision, the effect is statistically insignificant. Did either of the two replications “successfully replicate” the original? Did both? Did none? This issue has not been addressed in the economics replication literature.
After a replication is completed, after the results are reported and interpreted, there remains a final challenge for replications: Cataloguing the results. For those who read the original study, there is no easy way to determine whether replications of that study have been done, and what they have found. As a result, original studies may continue to have important policy impacts, even when subsequent replication efforts have weakened or overturned their results.
Summary and policy advice
Replications have many challenges, only some of which have been touched on here. Even when data and computer code exist that allow one to repeat the analysis of a previous study, there is no formula, no commonly accepted procedure, for how a replication should be done. Further, there is no commonly agreed set of criteria by which a replication can be said to “successfully replicate” a previous study.
Even so, replications have the potential to greatly clarify researchers’ and policymakers’ understanding of empirical relationships. By carefully reviewing and re-testing the conclusions of previous studies, replications can clarify which empirical findings in labor economics are reliable and robust, and which are not.
Three major actions could increase both the number of replications done and the value from doing them. First, while much progress has been made, it is still difficult to obtain data and code to reproduce results from previously published research, even when the journals require that the authors provide these. Strengthened open access policies for authors’ data and code would lower the cost of doing replications and encourage more replications to be undertaken. Second, if journals were willing to publish more replications, the benefits to doing replication would increase, resulting in more research of this type. And lastly, a system to catalogue replications and to link the original studies to subsequent replications would greatly increase the scientific value of replications. As things currently stand, a replication can overturn the results of an original study, and yet many subsequent readers of the original study will be unaware of its existence.
Acknowledgments
The author thanks the IZA World of Labor editors for many helpful suggestions on earlier drafts. Tom Coupé and Brian Haig also provided valuable comments. Previous work of the author has been relied upon throughout this article and contains a larger number of background references for the material presented here [4].
Competing interests
The IZA World of Labor project is committed to the IZA Guiding Principles of Research Integrity. The author declares to have observed these principles.
© W. Robert Reed